Effective Sentiment Analysis of Bengali Corpus by Using the Machine Learning Approach
Data Management, Analytics and Innovation(2022)
摘要
With the evolution of the social media-based application, users share their views, opinions and emotions liberally in language English and any native language like Bengali. The posts or comments collected from the social networking sites are needed to be analysed for identifying the positive or negative public sentiments regarding the products or services of any organization in order to improve their services. Over the last few years, research on sentiment analysis using Machine Learning (ML) tools is very popular. However, the sentiment analysis of Bengali data corpus using a Machine Learning tool is limited. It has been observed that the ML models for sentiment analysis are suffering from misclassification and researchers are still trying to come up with better solutions. In this paper, a Machine Learning approach is proposed for sentiment analysis of the Bengali data set collected from Facebook. Since the posts or comments that exhibit negative polarity are more actionable than the positive ones, the prime aim of this paper is to identify the negative sentiments without being misclassified and introduces a new technique to achieve almost 100% specificity by tuning the class weight of the ML classification model. We have also tried to improve the accuracy of ML classifiers during the sentiment analysis by combining the Part-of-Speech (POS) tagging with Term Frequency—Inverse Document Frequency (TF-IDF) vectorization. The overall accuracy of each ML classifier for combining the POS tagging with TF-IDF shows an increment of 2.74–4.17% from the TF-IDF vectorization without combining with POS tagging.
更多查看译文
关键词
Class-weight, POS (part-of-speech) tagging, Specificity, Sentiment analysis, TF-IDF (Term Frequency–Inverse Document Frequency)
AI 理解论文
溯源树
样例
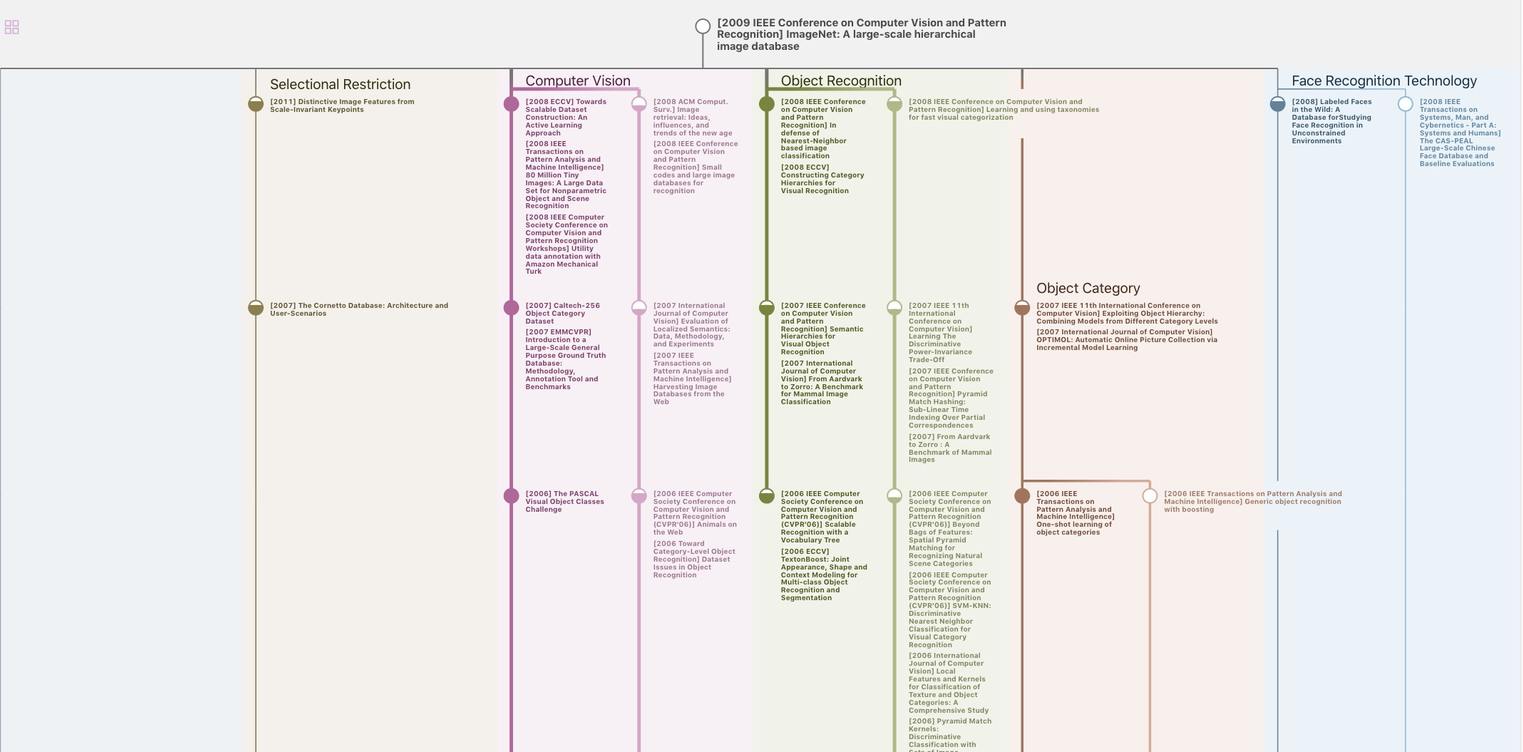
生成溯源树,研究论文发展脉络
Chat Paper
正在生成论文摘要