On Modelling Statistically Independent Nonlinear Normal Modes with Gaussian Process NARX Models
Nonlinear Structures & Systems, Volume 1(2022)
摘要
Linear modal analysis has provided a robust and eminently useful framework for the analysis of structural dynamic systems. Considerable attention has been directed towards the development of a nonlinear variant of modal analysis that is effective in the presence of nonlinearities. Thus far, essentially two approaches to constructing nonlinear normal modes (NNM) have gained traction. The first was that of Rosenberg, whereby the modes are defined in terms of synchronous motions of the structure. The second is the geometrically more general approach of Shaw and Pierre, wherein modes are defined on invariant manifolds of the phase space of the system. A recent third approach from Worden and Green proposes a statistical definition of a nonlinear normal mode. Under this framework, the modal coordinates are defined by latent directions in the configuration space that result in statistically uncorrelated time series. This paper examines the properties of the NNMs generated by this framework, by applying techniques from nonlinear system identification (NLSI). Both linear and nonlinear time domain models are fitted to the physical and modal coordinates of a two degree-of-freedom simulated system with a cubic nonlinearity. It is demonstrated in this work, that the NNMs generated are able to decompose the system into independent functionals, and that the modal transformation generalises to lower excitation levels, while maintaining excellent reconstruction of the physical displacements.
更多查看译文
关键词
Nonlinear normal modes, Time series analysis, Gaussian process NARX models
AI 理解论文
溯源树
样例
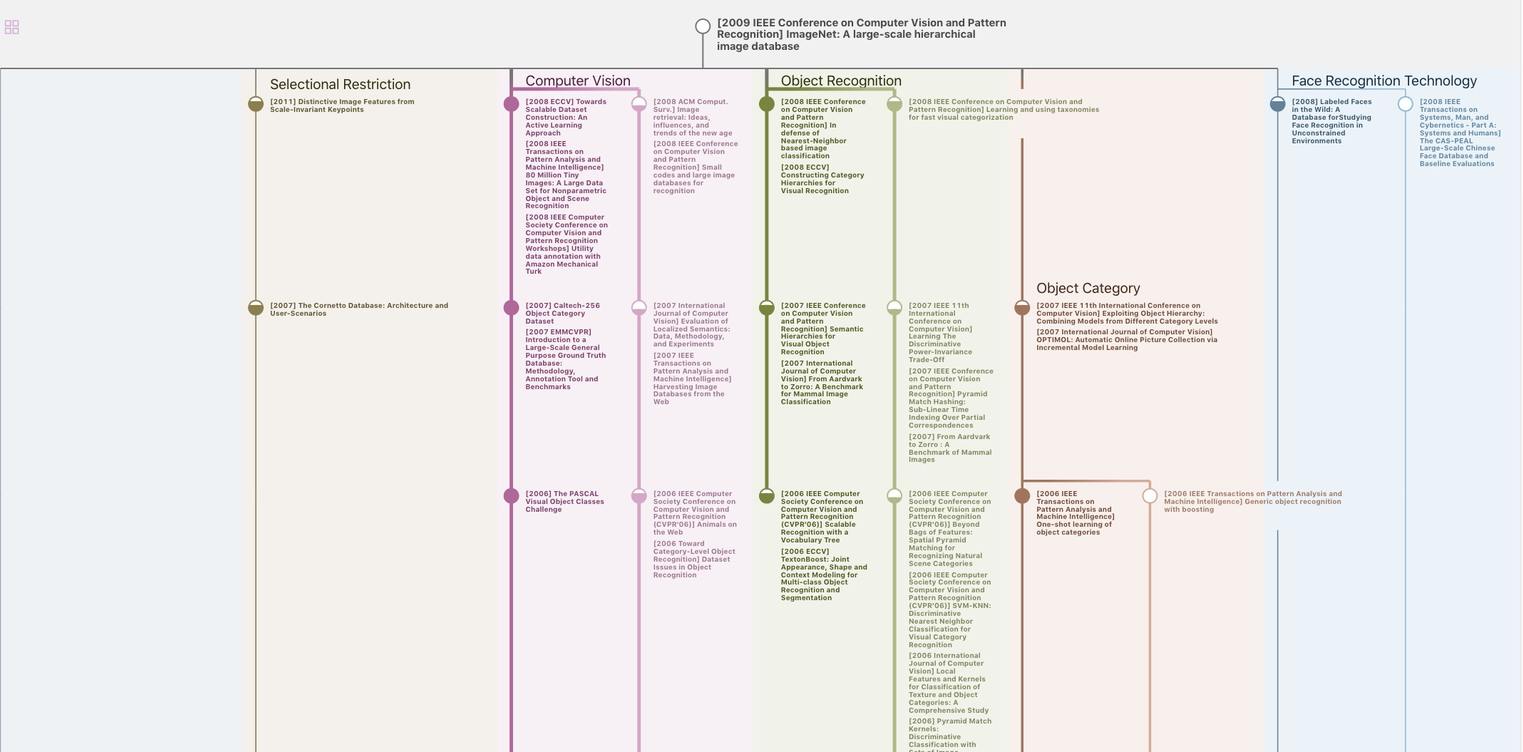
生成溯源树,研究论文发展脉络
Chat Paper
正在生成论文摘要