Evaluating YOLO Transferability Limitation for Road Infrastructures Monitoring
Novel & Intelligent Digital Systems: Proceedings of the 2nd International Conference (NiDS 2022)(2022)
摘要
Road infrastructure is positively associated with a country’s socio-economic growth and therefore road maintenance is of great importance for every country. One of the critical maintenance steps is road damage detection, which typically requires large amounts of time and high costs. In this work, the YOLOv5 two-stage detector is leveraged, in order to create an image-based solution for road defect detection and classification. The damages are classified into three main categories: cracks, potholes, and blurred markings. The YOLOv5 can achieve a relatively high detection accuracy with a score of Intersection over Union (IoU) up to 88.89% and classification accuracy with an F1 score up to 80.72%. The precision and recall scores are 84.26% and 78.38%, respectively.
更多查看译文
关键词
Road defects, Deep learning, Computer vision, Object detection
AI 理解论文
溯源树
样例
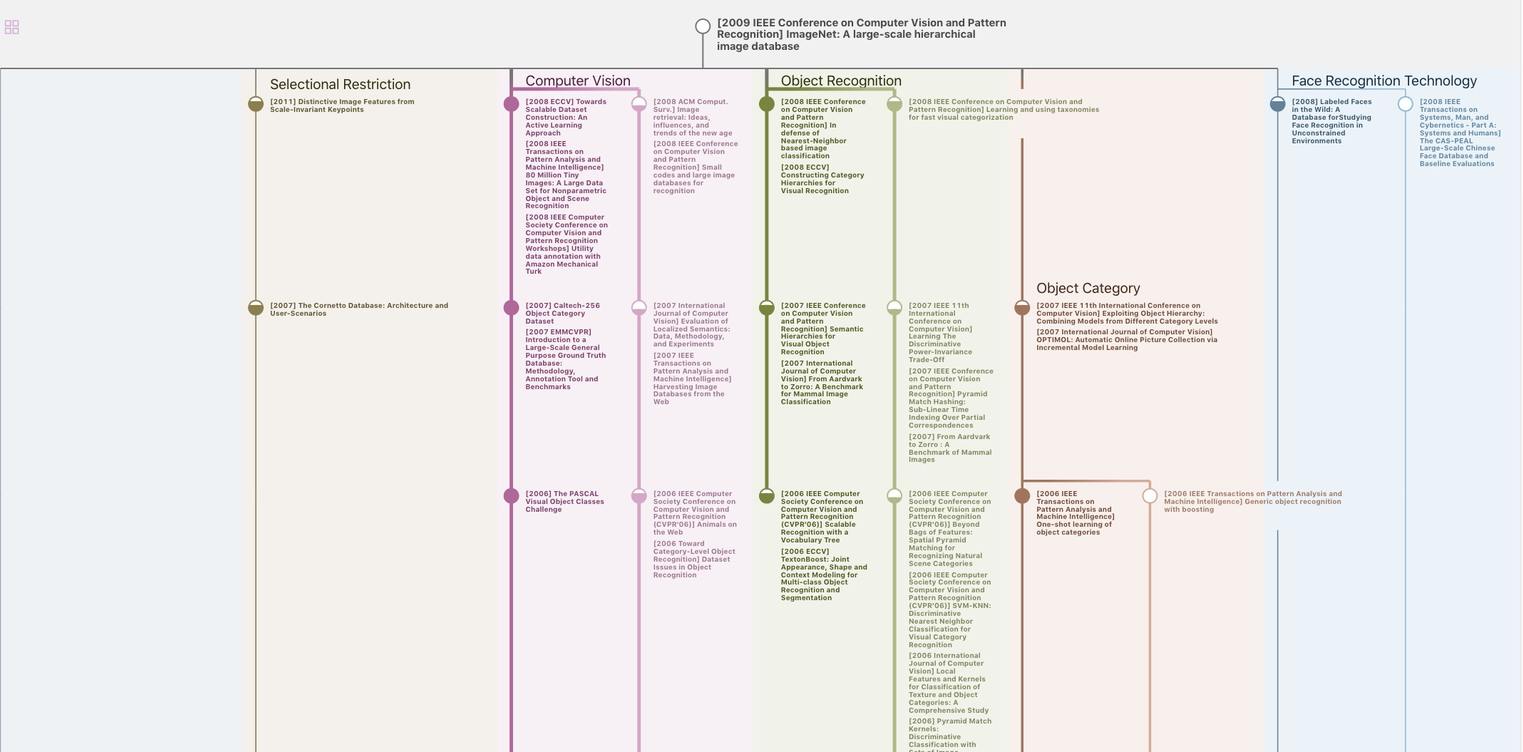
生成溯源树,研究论文发展脉络
Chat Paper
正在生成论文摘要