Metric learning algorithms for meta learning
Meta-Learning with Medical Imaging and Health Informatics Applications(2023)
摘要
This chapter introduces metric-learning approaches for meta learning. The central theme is to learn a good feature embedding space where learning systems can easily classify different classes given only a few training examples. The first method is Siamese Networks, which takes a pair of samples and produces a similarity score. Matching Networks improve over Siamese Networks with more sophisticated attention-based embedding functions to make the final features dependent on the entire support set. Prototypical Networks seek an embedding space where data samples for each class form a compact cluster around a prototype. Instead of using nonparametric classifiers like previous approaches, Relation Networks aim to learn both the embedding and classification function. Finally, Graph Neural Networks can generalize metric-learning-based meta learning.
更多查看译文
关键词
metric learning algorithms,meta learning
AI 理解论文
溯源树
样例
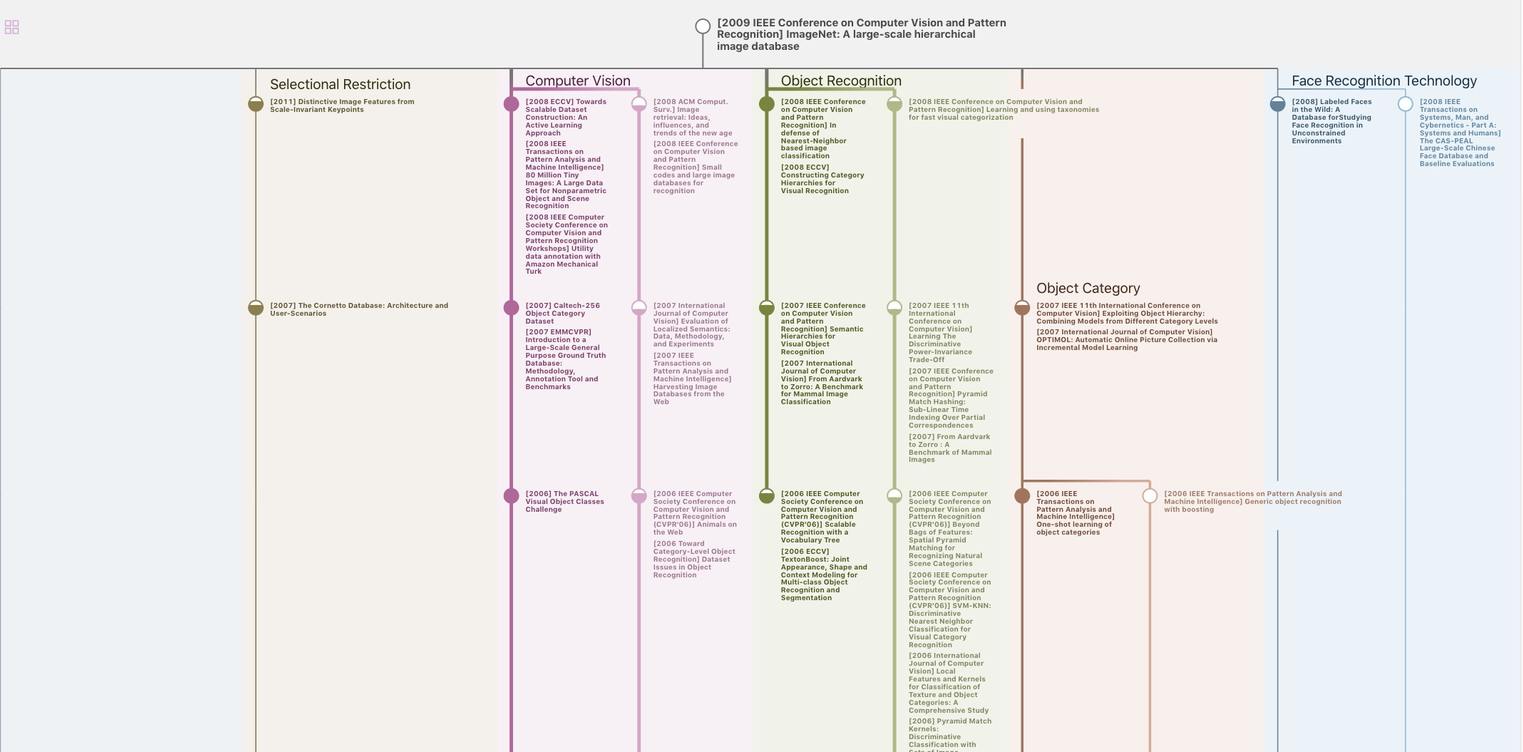
生成溯源树,研究论文发展脉络
Chat Paper
正在生成论文摘要