Deep Learning Based Short-Term Total Cloud Cover Forecasting
IEEE International Joint Conference on Neural Network (IJCNN)(2022)
摘要
In this research, we conduct deep learning based Total Cloud Cover (TCC) forecasting using satellite images. The proposed system employs the Otsu's method for cloud segmentation and Long Short-Term Memory (LSTM) variant models for TCC prediction. Specifically, a region-based Otsu's method is used to segment clouds from satellite images. A time-series dataset is generated using the TCC information extracted from each image in image sequences using a new feature extraction method. The generated time series data are subsequently used to train several LSTM variant models, i.e. LSTM, bi-directional LSTM and Convolutional Neural Network (CNN)-LSTM, for future TCC forecasting. Our approach achieves impressive average RMSE scores with multi-step forecasting, i.e. 0.0543 and 0.0823, with respect to both the first half of daytime and full daytime TCC forecasting on a given day, using the generated dataset.
更多查看译文
关键词
Long Short-Term Memory,Deep Learning,Total Cloud Cover,Time-series Forecasting,Satellite Imaging
AI 理解论文
溯源树
样例
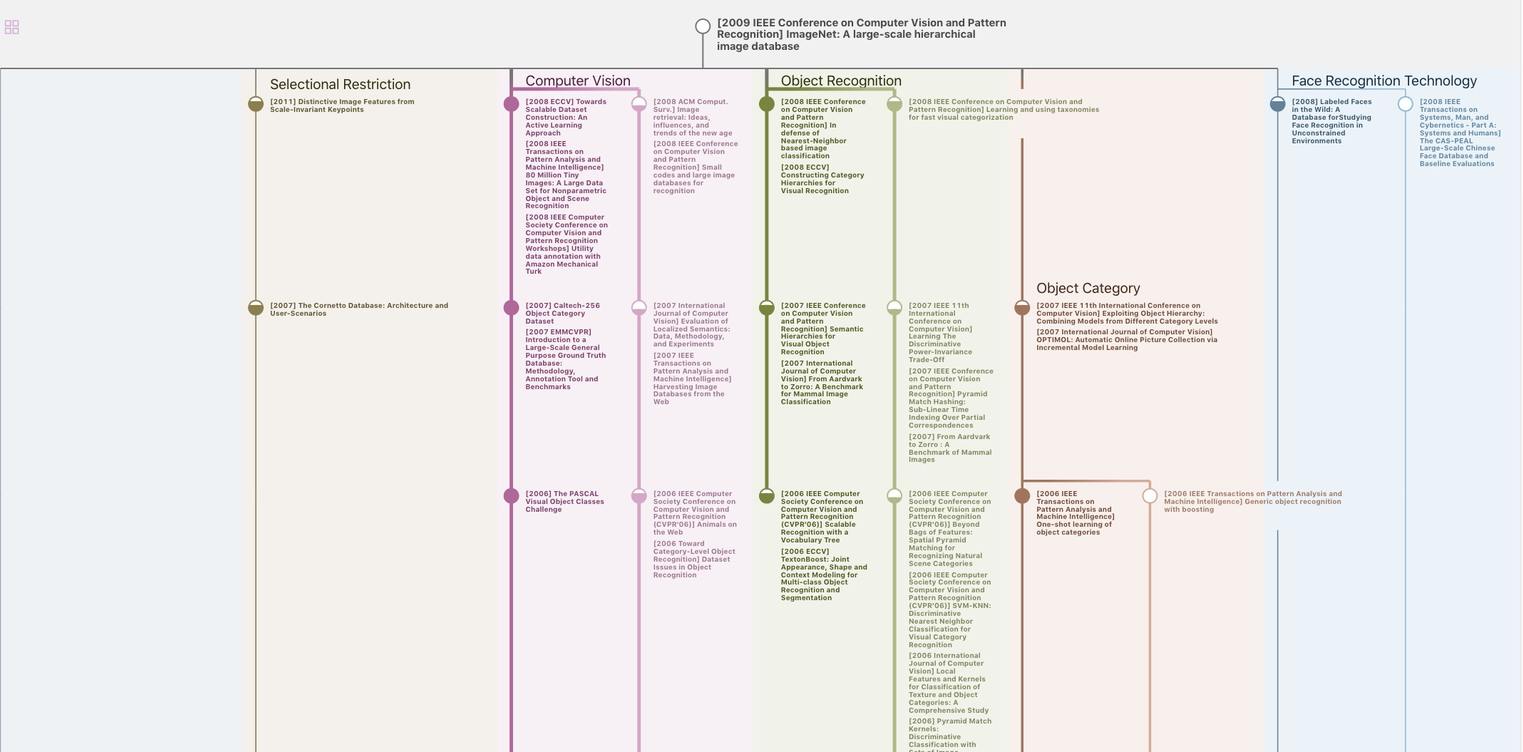
生成溯源树,研究论文发展脉络
Chat Paper
正在生成论文摘要