Mix-up Consistent Cross Representations for Data-Efficient Reinforcement Learning
IEEE International Joint Conference on Neural Network (IJCNN)(2022)
摘要
Deep reinforcement learning (RL) has achieved remarkable performance in sequential decision-making problems. However, it is a challenge for deep RL methods to extract task-relevant semantic information when interacting with limited data from the environment. In this paper, we propose Mixup Consistent Cross Representations (MCCR), a novel selfsupervised auxiliary task, which aims to improve data efficiency and encourage representation prediction. Specifically, we calculate the contrastive loss between low-dimensional and high-dimensional representations of different state observations to boost the mutual information between states, thus improving data efficiency. Furthermore, we employ a mixed strategy to generate intermediate samples, increasing data diversity and the smoothness of representations prediction in nearby timesteps. Experimental results show that MCCR achieves competitive results over the state-of-the-art approaches for complex control tasks in DeepMind Control Suite, notably improving the ability of pretrained encoders to generalize to unseen tasks.
更多查看译文
关键词
mutual information,smoothness,self-supervised learning,reinforcement learning
AI 理解论文
溯源树
样例
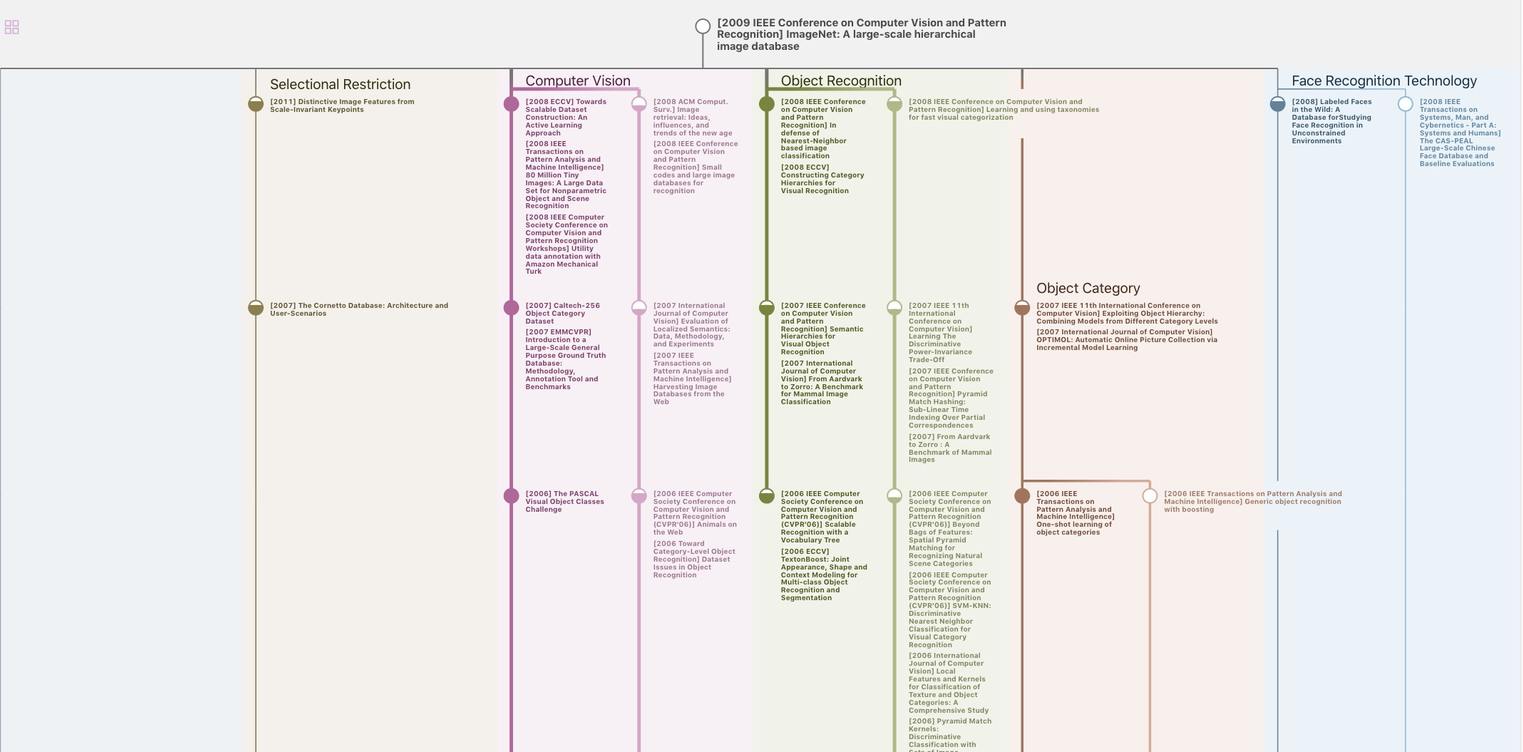
生成溯源树,研究论文发展脉络
Chat Paper
正在生成论文摘要