Fourier filter-based physics- information convolutional recurrent network for 2D incompressible flow
Frontiers in Physics(2022)
摘要
Physics-informed convolutional recurrent network (PhyCRNet) can solve partial differential equations without labeled data by encoding physics constraints into the loss function. However, the finite-difference filter makes the solution of 2D incompressible flow challenging. Hence, this paper proposes a Fourier filter-based physics-informed convolution recurrent network (named Fourier filter-based PhyCRNet), which replaces the finite-difference filter in PhyCRNet with the Fourier filter to solve the 2D incompressible flow problem. The suggested network improves the accuracy of the partial derivatives, solves the inverse Laplacian operator, and has similar generalization ability due to inheriting the framework of PhyCRNet. Four examples, including the 2D viscous Burger, FitzHugh–Nagumo RD, vorticity and the two-dimensional Navier- Stokes (N-S) equations, validate the correctness and reliability of the proposed Fourier filter-based PhyCRNet.
更多查看译文
关键词
fourier filter,convolutional recurrent network,2D incompressible flow,inverse laplacian operator,physics-informed
AI 理解论文
溯源树
样例
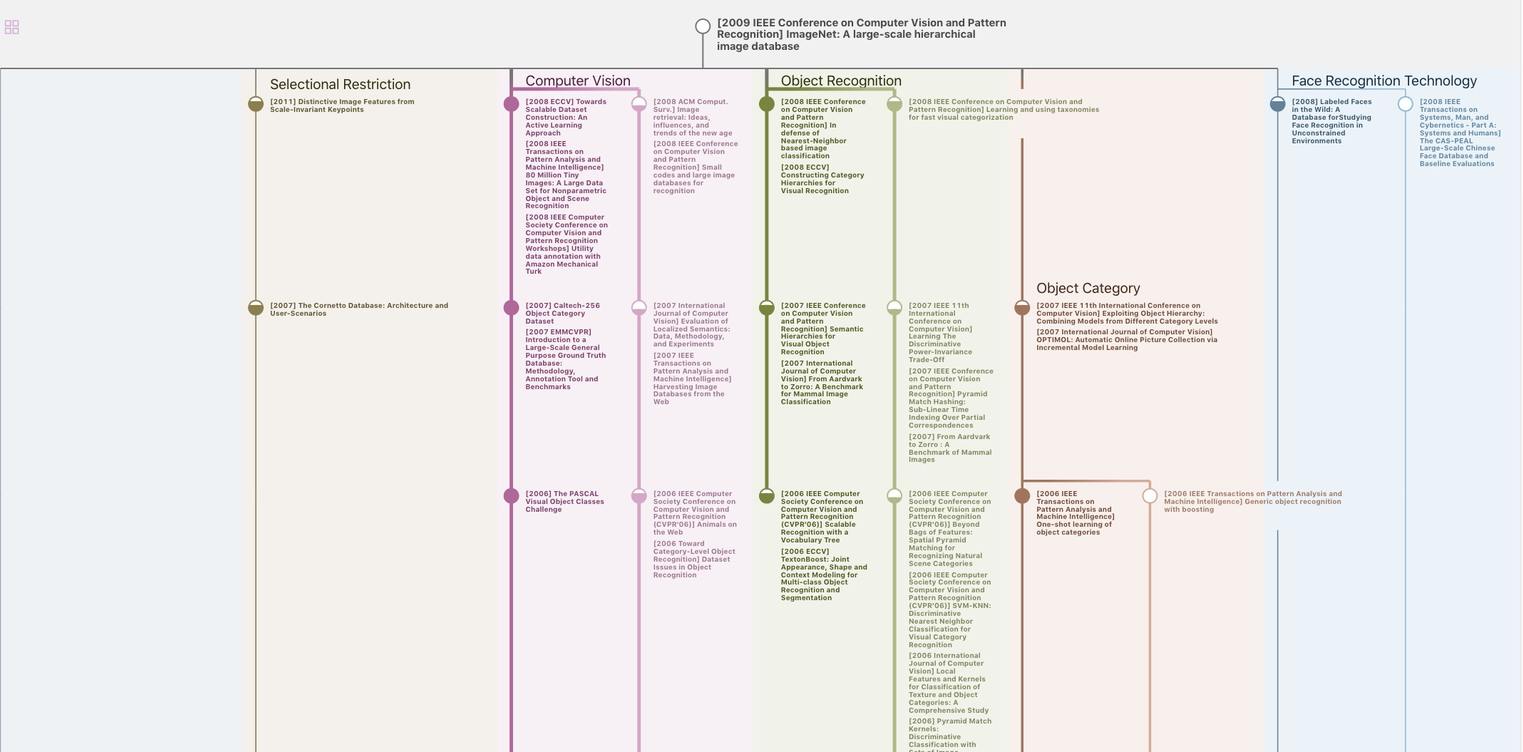
生成溯源树,研究论文发展脉络
Chat Paper
正在生成论文摘要