A novel two-stage method for well-diversified portfolio construction based on stock return prediction using machine learning
The North American Journal of Economics and Finance(2022)
摘要
The performance of portfolio model can be improved by introducing stock prediction based on machine learning methods. However, the prediction error is inevitable, which may bring losses to investors. To limit the losses, a common strategy is diversification, which involves buying low-correlation stocks and spreading the funds across different assets. In this paper, a diversified portfolio selection method based on stock prediction is proposed, which includes two stages. To be specific, the purpose of the first stage is to select diversified stocks with high predicted returns, where the returns are predicted by machine learning methods, i.e. random forest (RF), support vector regression (SVR), long short-term memory networks (LSTM), extreme learning machine (ELM) and back propagation neural network (BPNN), and the diversification level is measured by Pearson correlation coefficient. In the second stage, the predictive results are incorporated into a modified mean-variance (MMV) model to determine the proportion of each asset. Using China Securities 100 Index component stocks as study sample, the empirical results demonstrate that the RF+MMV model achieves better results than similar counterparts and market index in terms of return and return-risk metrics.
更多查看译文
关键词
Portfolio selection,Stock prediction,Machine learning,Mean-variance model,Diversification
AI 理解论文
溯源树
样例
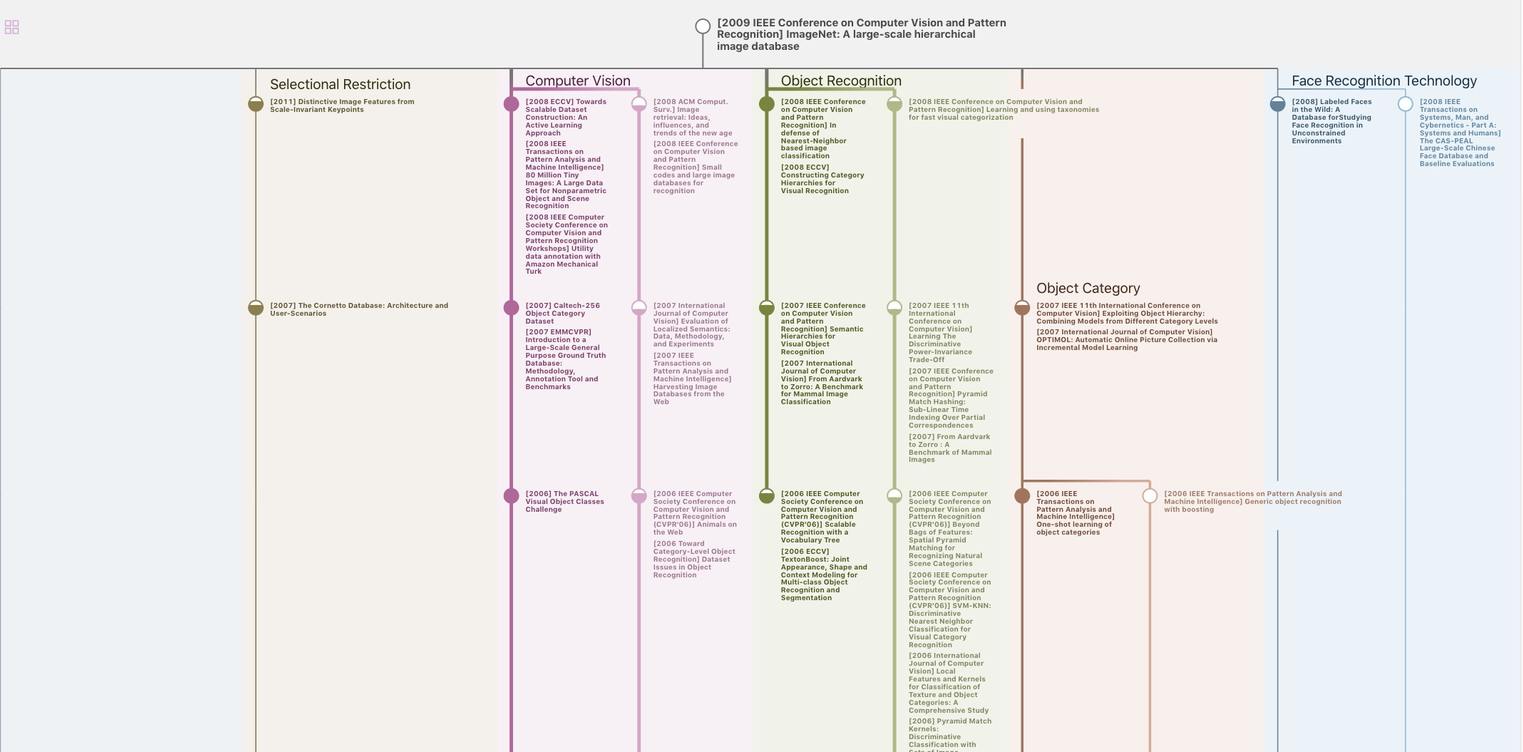
生成溯源树,研究论文发展脉络
Chat Paper
正在生成论文摘要