High-order Correlation Network for Video Recognition
IEEE International Joint Conference on Neural Network (IJCNN)(2022)
摘要
How to model global video representation is an important research content of video recognition. Among current convolutional neural network(CNN) based methods, only using first-order representations (i.e. global average pooling) has limitations in capturing spatiotemporal features of videos. Recent studies have shown that high-order statistics are more suitable to model complex feature distributions. To better characterize the spatiotemporal structure for video recognition, we propose a novel High-order Correlation Network (HoCNet) in this work, the core of which is to explore high-order video representations through correlation computation and covariance pooling. HoCNet leverages the correlation module to obtain complex temporal dynamic information of frames via computing dot product of features in the fixed sliding window of two adjacent frames. As an approximate high-order calculation, the correlation module can be inserted into any stage of the deep network to model high-order representations in various spatial resolutions. Additionally, a robust high-order pooling module, i.e., iterative matrix square root normalization of covariance pooling (iSQRT-COV), is also introduced at the end of the network, and this further boosts modeling complex spatiotemporal distributions of video features. Experiments conducted on four widely used video benchmarks demonstrate the effectiveness of HoCNet, which achieves the comparable performance with the state-of-the-art models.
更多查看译文
关键词
video recognition,covariance pooling,second-order,correlation,deep network
AI 理解论文
溯源树
样例
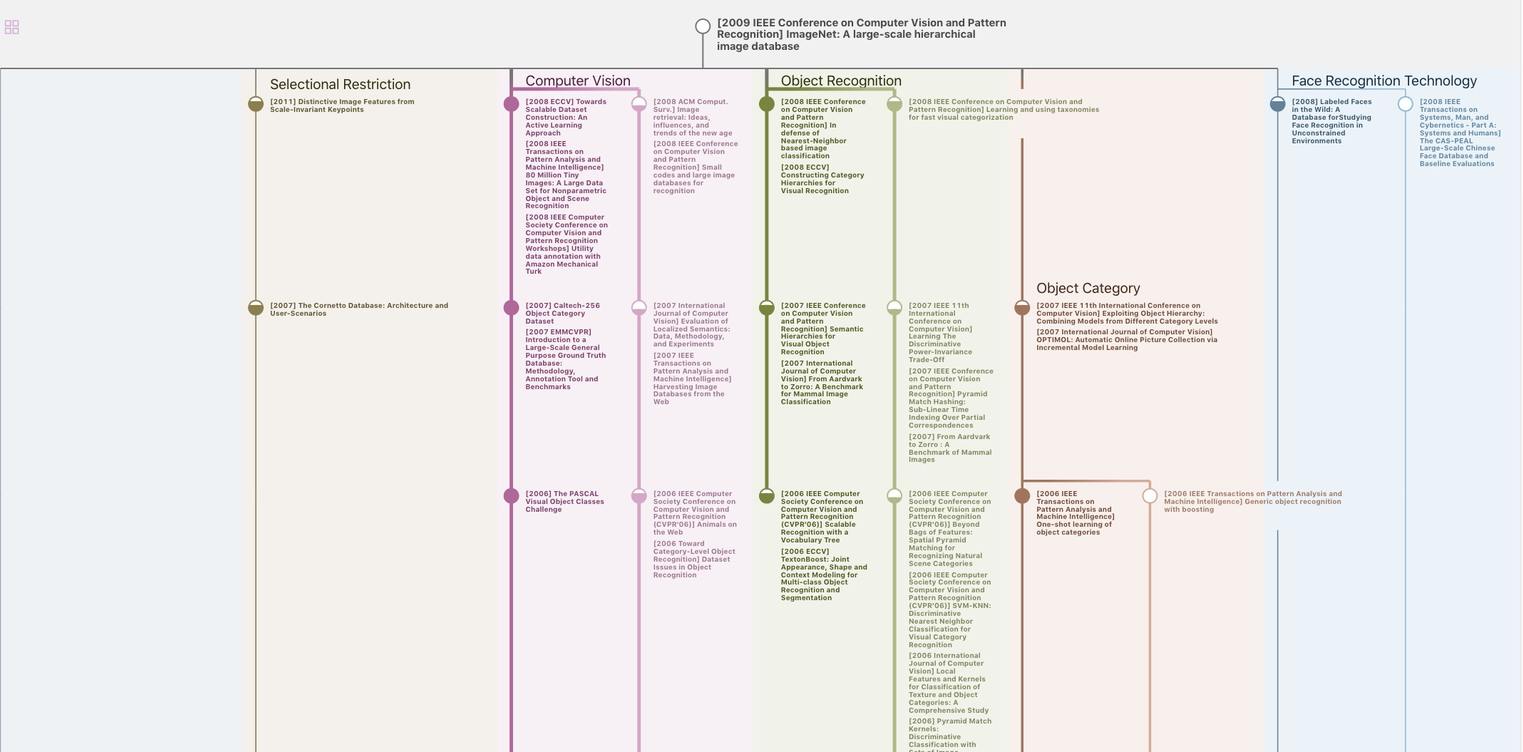
生成溯源树,研究论文发展脉络
Chat Paper
正在生成论文摘要