Learning to Stabilize Extreme Neural Machines with Metaplasticity
IEEE International Joint Conference on Neural Network (IJCNN)(2022)
摘要
Training recurrent reservoir networks to solve complex tasks is a difficult problem due to extensive training times and the ambiguity of adjusting synaptic weights located deep within the architecture of the network. Recently, a novel model termed Extreme Neural Machine (ENM) has been proposed to perform one-shot learning in recurrent networks. A drawback of this approach, however, is that synaptic weights may become destabilized after training due to the random activation of recurrent units. In this paper, we propose a solution to this problem by incorporating metaplasticity in the learning rule of ENMs. With this novel approach, networks learned complex functions that can be recalled after a delay period. Using real-world data, networks were trained to produce sound waveforms obtained from spoken English words. Results show that metaplasticity improved task recall in recurrent neural networks.
更多查看译文
关键词
recurrent networks,extreme neural machines,one-shot learning,speech production
AI 理解论文
溯源树
样例
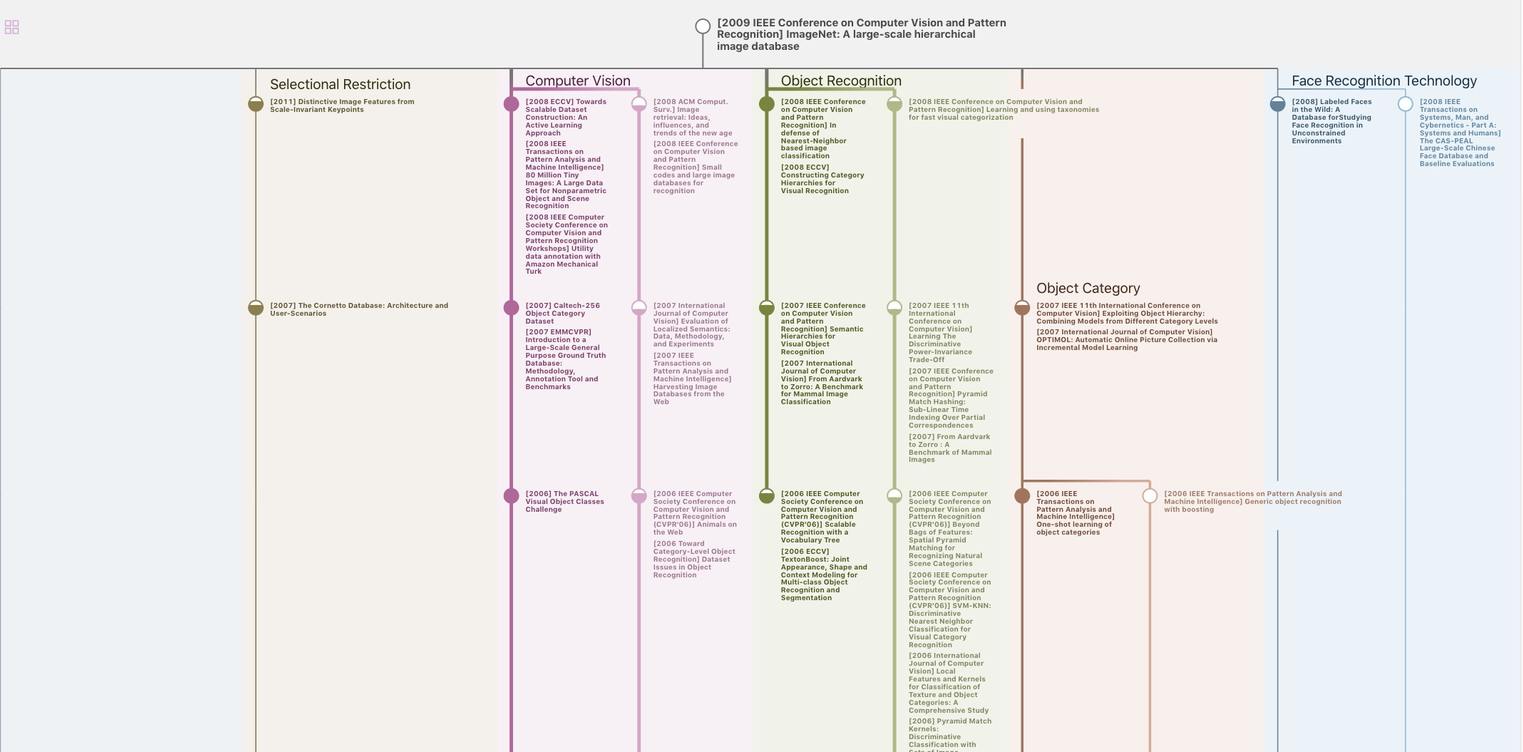
生成溯源树,研究论文发展脉络
Chat Paper
正在生成论文摘要