Composite Recurrent Convolutional Neural Networks Offer a Position-Aware Prosthesis Control Alternative While Balancing Predictive Accuracy with Training Burden
2022 International Conference on Rehabilitation Robotics (ICORR)(2022)
摘要
To mitigate the “limb position effect” that hinders myoelectric upper limb prosthesis control, pattern recognition-based models must accurately predict user-intended movements across a multitude of limb positions. Such models can use electromyography (EMG) and inertial measurement units to capture necessary multi-position data. However, this data capture solution requires lengthy user-performed model training routines, with movements in many limb positions, plus retraining thereafter due to inherent signal variations over time. While a general-purpose control model (trained with a dataset that represents numerous device users) eliminates the user-training requirement altogether, it yields low movement predictive accuracy. Conversely, a user-specific control model (trained with a smaller dataset from an individual) yields high predictive accuracy, but requires retraining over time. This study capitalizes on the benefits offered by both such control options, and contributes an alternative control solution—a novel recurrent convolutional neural network (RCNN)-based Composite Model that combines the representation portion of a general-purpose model, with the decision portion of a user-specific model. The resulting Composite Model offers moderate movement predictive accuracy across various limb positions and a reduction in user training routine requirements, suggesting a new research direction to help mitigate the limb position effect along with model training burden.
更多查看译文
关键词
Artificial Limbs,Electromyography,Humans,Movement,Neural Networks, Computer,Pattern Recognition, Automated
AI 理解论文
溯源树
样例
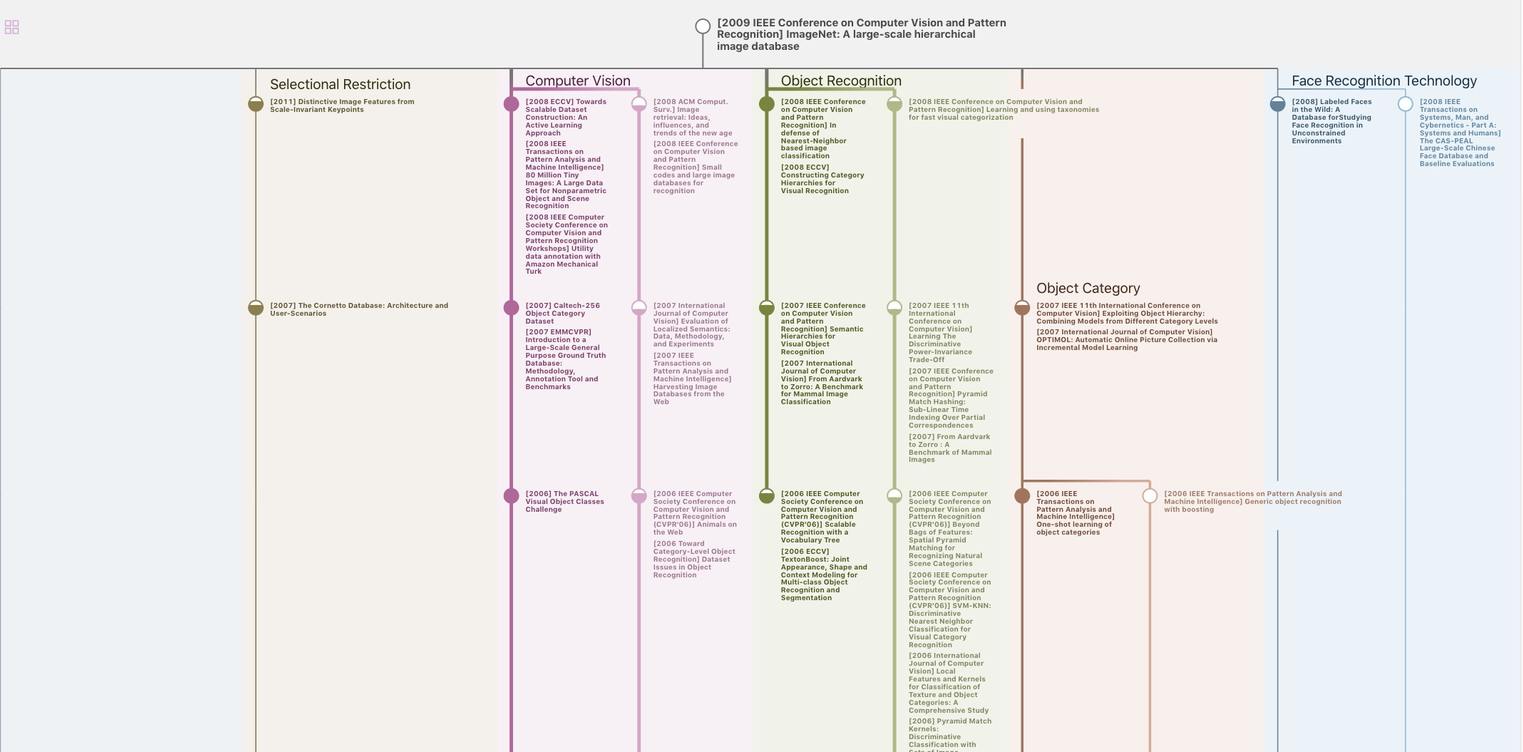
生成溯源树,研究论文发展脉络
Chat Paper
正在生成论文摘要