MATN: Multi-model Attention Network for Gait Prediction from EEG
IEEE International Joint Conference on Neural Network (IJCNN)(2022)
摘要
Predicting gait trajectories from electroencephalography (EEG) is useful for lower limb rehabilitation. The temporal dynamics of EEG pertaining to individual subjects while they are walking require a personalized treatment inside the deep neural network. Also, the EEG data collected from different sessions or days may exhibit varied distributions, further hindering the model generality. Therefore, we propose the Multi-model ATtention Network (MATN) to address these issues. MATN exploits the self-attention mechanism on the spatio-temporal EEG encoding to learn the subject-specific temporal dynamics. To adapt to varied data distributions, MATN employs knowledge distillation, which includes a teacher and student model. The latter is supervised by the intermediate feature maps from the trained teacher and the labels. Experiments of the regression of bilateral joint angles on the legs (hip, knee, and ankle) are carried out using the MoBI dataset in a subject-specific manner. Our MATN achieves more than 18% improvement on the Pearson's correlation coefficient compared to five other state-of-the-art methods.
更多查看译文
关键词
deep learning,convolutional neural network,electroencephalography,motor imagery,gait trajectories prediction
AI 理解论文
溯源树
样例
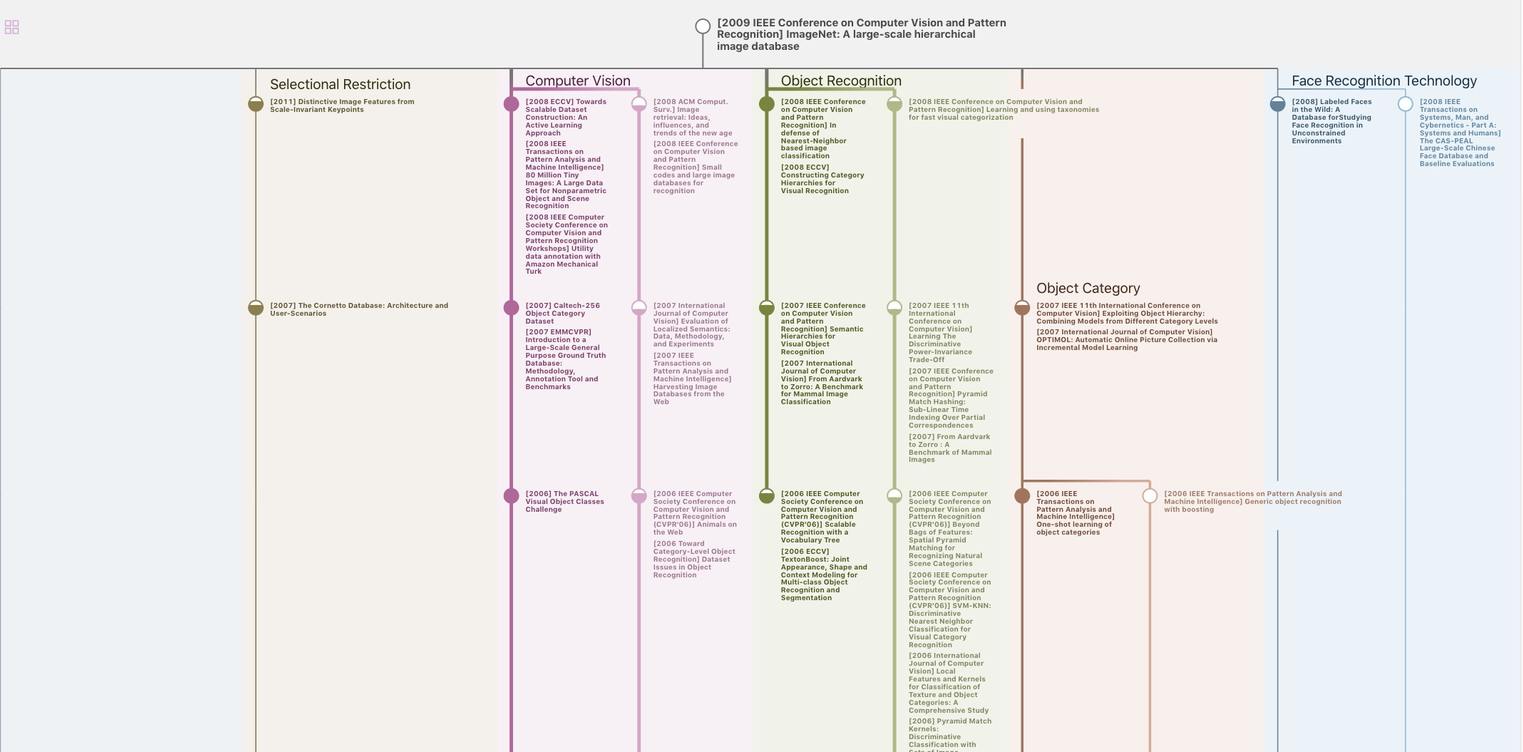
生成溯源树,研究论文发展脉络
Chat Paper
正在生成论文摘要