Sequential Robust Parameter Design With Sample Size Selection
Volume 2: Manufacturing Processes; Manufacturing Systems(2022)
摘要
Abstract In designing engineering systems, it is crucial to find a robust design whose responses have minimal variations and satisfies a constraint on the mean, also known as robust parameter design (RPD). Such optimization is challenging because collecting data is often expensive, and modern engineering systems commonly produce responses whose mean and variance are complex unknown functions of control variables. To address these challenges, we propose a stochastic constrained Bayesian optimization for RPD. We construct the Gaussian process dual response surrogate models for the mean and variance of the response by the sample mean and sample variance. The predicted mean and quantified uncertainties through the surrogate models are utilized to exploit the predictions and explore the regions with high uncertainty in the proposed Bayesian optimization method. Because the sample size of the sample mean and sample variance affects the performance significantly, we propose an effective sample size selection scheme, which effectively balances between exploitation and exploration during optimization. The performance of our method is demonstrated in numerical and case studies. In the case study, we used the real-world data for the robust design of the Graphene field-effect transistor nanosensors.
更多查看译文
AI 理解论文
溯源树
样例
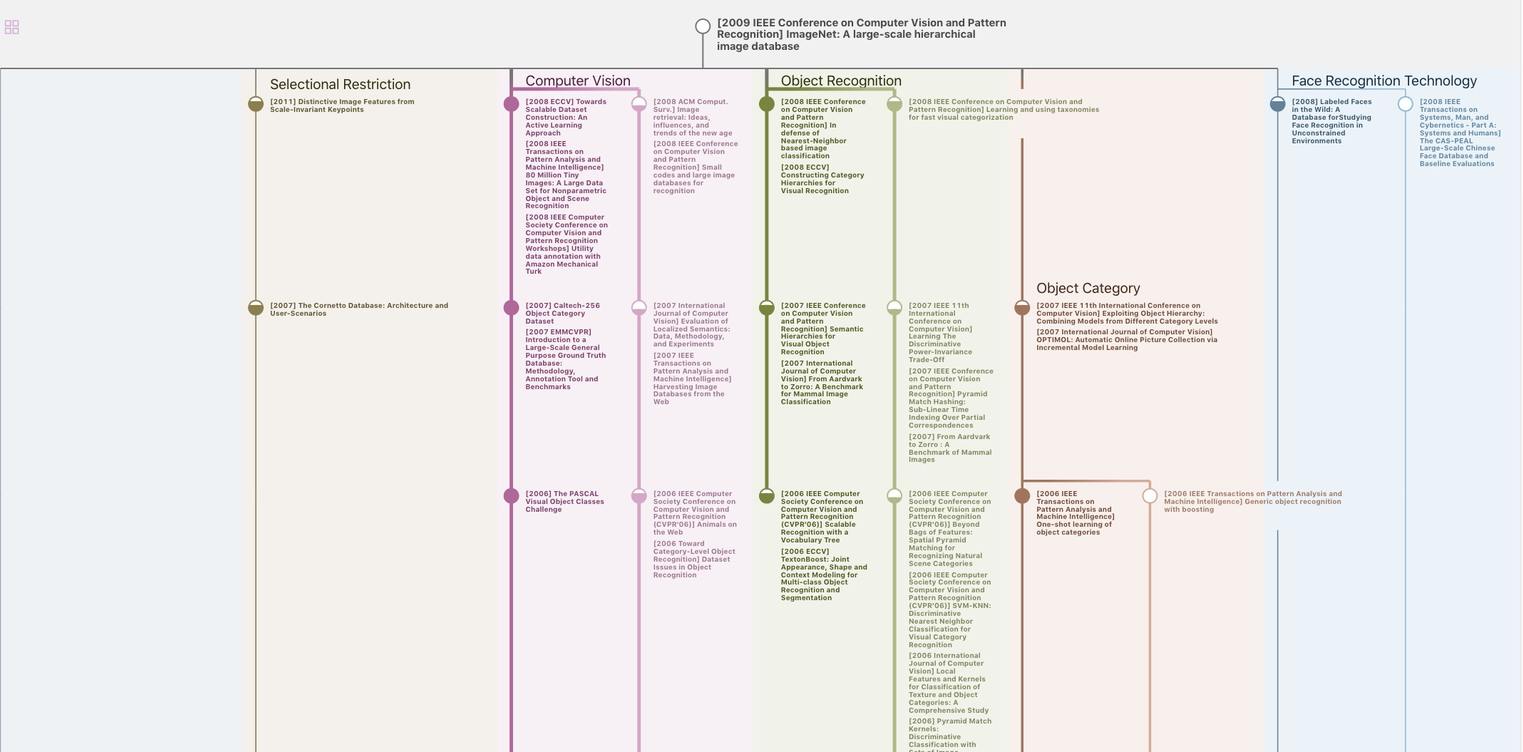
生成溯源树,研究论文发展脉络
Chat Paper
正在生成论文摘要