A Multi-Agent Deep Reinforcement Learning Framework for VWAP Strategy Optimization
2022 International Joint Conference on Neural Networks (IJCNN)(2022)
摘要
As a classical optimal trade execution algorithm, Volume Weighted Average Price (VWAP) strategy is favored by brokers. Since it is schedule-based and cannot perform well in a dynamic stock market, optimizing the traditional VWAP strategy via reinforcement learning is worth investigating. Most of the existing reinforcement learning-based execution strategies focus on formulating trading volumes or trading prices separately, ignoring the cooperation between trading volumes and trading prices. To address this issue, we propose a Multi-Agent Deep Q-Network (MADQN) trading framework to optimize the traditional VWAP strategy, which can dynamically adapt to the complex stock market and simultaneously formulate trading prices and volumes at each transaction period. Specifically, we design two different types of agents: 1) volume-driven agent for determining trading volumes at each transaction period and 2) price-driven agent for deciding trading prices. We model our stock market environment in which multiple agents participate as a fully cooperative stochastic game. The volume-driven agent and the price-driven agent take joint actions to interact with the stock market environment and then update their network respectively. We use 9-month level-2 data of eight stocks from different sectors on Shanghai Stock Exchange as experimental data. Experimental results demonstrate that our MADQN trading framework outperforms baselines in terms of several evaluation metrics.
更多查看译文
关键词
VWAP Strategy,Optimal Trade Execution,Multi-Agent Deep Reinforcement Learning,Deep Q-Network
AI 理解论文
溯源树
样例
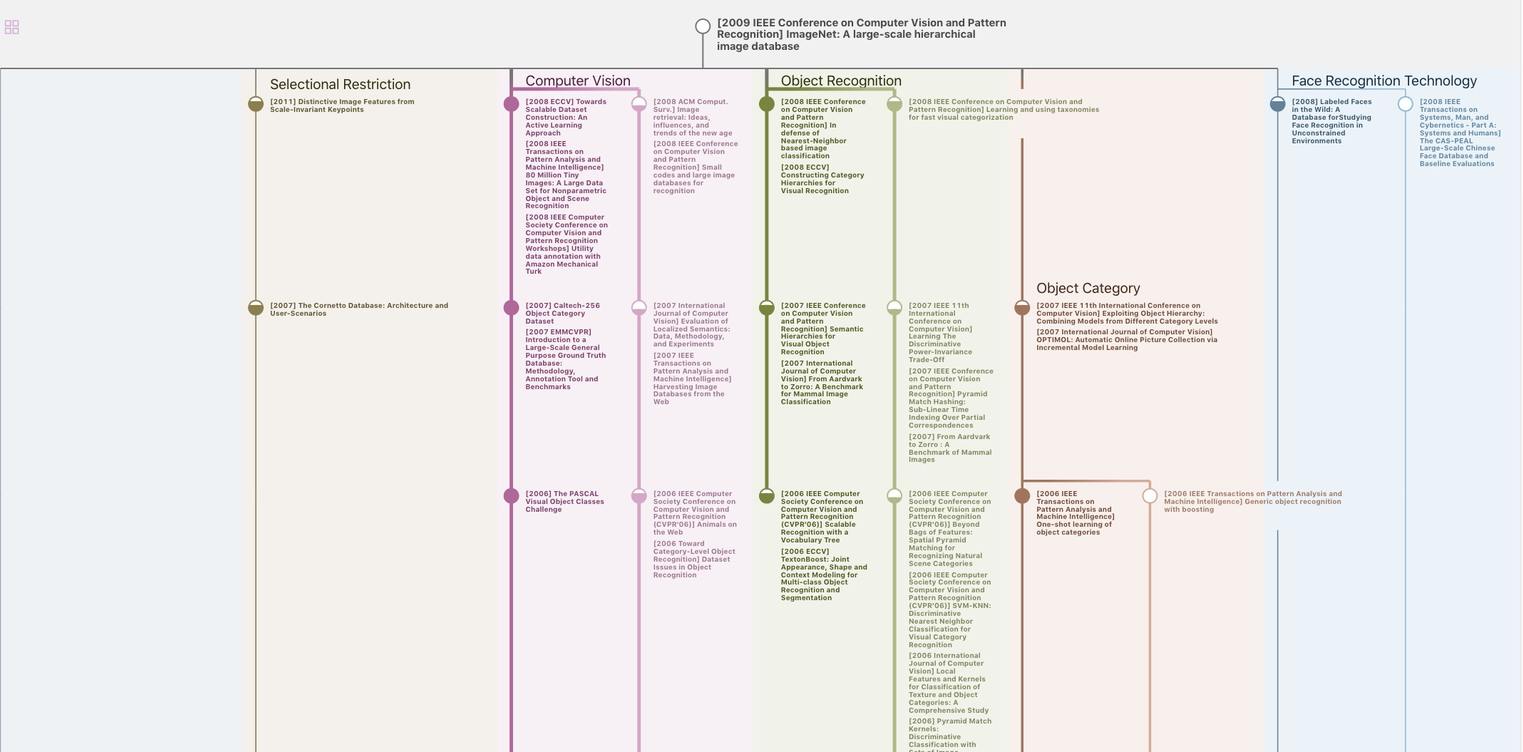
生成溯源树,研究论文发展脉络
Chat Paper
正在生成论文摘要