Comparing Neural Networks for Speech Emotion Recognition in Customer Service Interactions
2022 International Joint Conference on Neural Networks (IJCNN)(2022)
摘要
Automatic speech emotion recognition (SER) may assist call center service employees in deciphering and regulating customer emotions. In order to contribute to a successful augmentation of service employees with AI, the main goal of this study is to identify effective machine learning approaches to classify discrete basic emotions in customer service conversations. A comparison is presented of the recognition performance of different neural network architectures on speech features extracted from service interactions in a naturalistic customer service setting. Baseline classifiers, including a zero-rule classifier, a random classifier, a frequency classifier, and non-sequential multi-class classifiers are compared to different neural network architectures. A multi-layer perceptron (MLP), a one-dimensional convolutional neural network (CNN), and a neural machine translation (NMT) outperform the baseline classifiers, suggesting a pattern in the data relating to emotion labels. While the neural machine translation model with attention attains the highest f1-score, no significant difference in performance among the neural networks is detected. Results therefore support the use of the the multi-label multi-layer perceptron as the simplest model.
更多查看译文
关键词
Deep neural networks,Neural machine translation,Speech emotion recognition,Call center service interactions
AI 理解论文
溯源树
样例
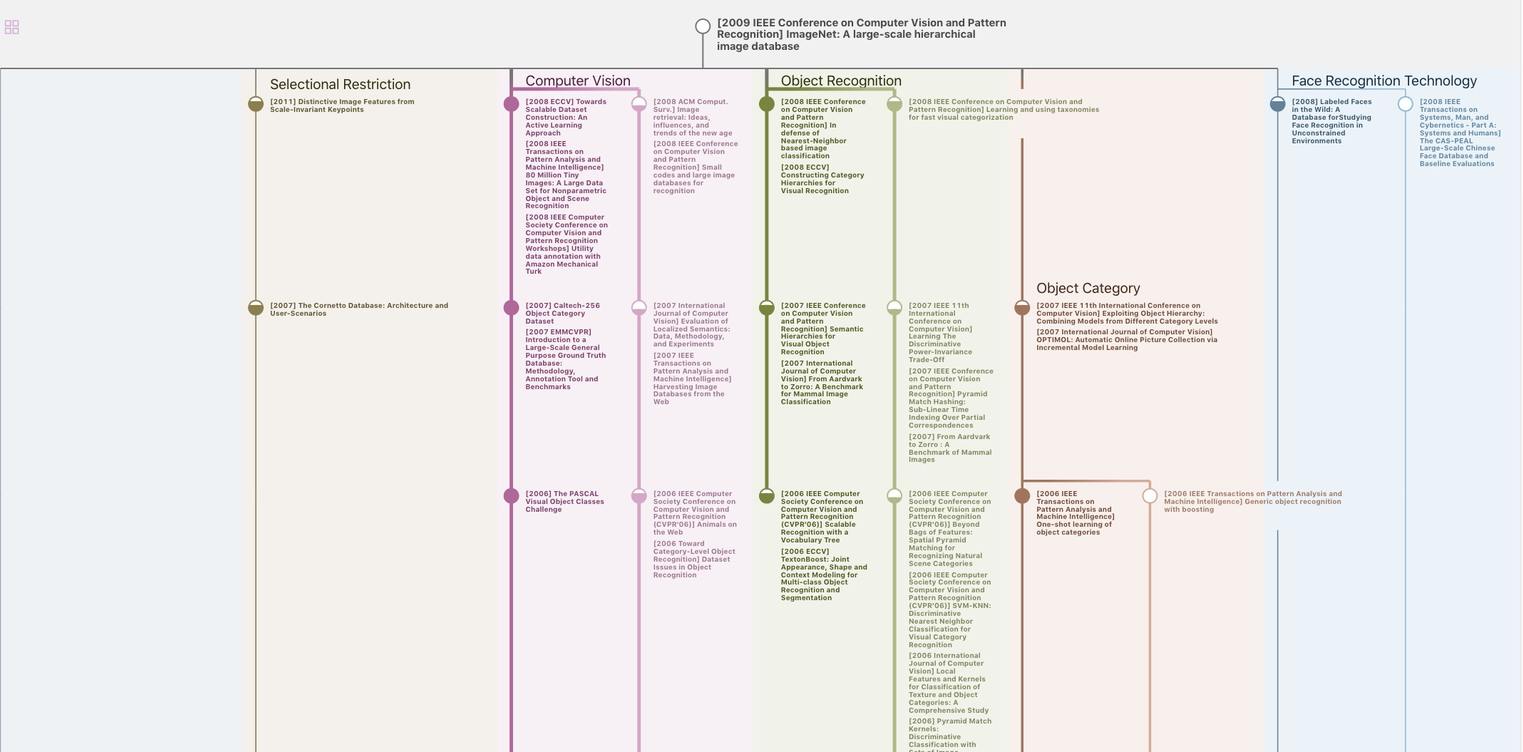
生成溯源树,研究论文发展脉络
Chat Paper
正在生成论文摘要