A Comparison of Uncertainty Quantification Methods for Active Learning in Image Classification
IEEE International Joint Conference on Neural Network (IJCNN)(2022)
摘要
The adoption of machine learning (ML) technology in real-world settings like medical imaging is currently hampered by a lack of trust in ML models and a lack of labeled data. These two issues are currently addressed in parallel by two sub-disciplines of ML: uncertainty quantification (UQ) is concerned with obtaining reliable estimates of a model's confidence in its outputs, and active learning (AL) deals with efficiently training models in low-data regimes. To date, the usefulness of the new methods emerging from the field of UQ for AL remains under-explored. We here take a step to address this by comparing seven different UQ methods on three image classification data sets. Our experiments confirm previous indications in the AL literature that the ranking of sampling strategies can vary greatly across models and data sets. We find that Concrete Dropout, Least Confidence, Smallest Margin, and Entropy sampling consistently outperform Random sampling across data sets, whereas Ensembles, Monte-Carlo Dropout, and Bayes-by-Backprop do not. We also observe that AL training stability is sensitive to data quality.
更多查看译文
关键词
active learning,machine learning,uncertainty,uncertainty quantification
AI 理解论文
溯源树
样例
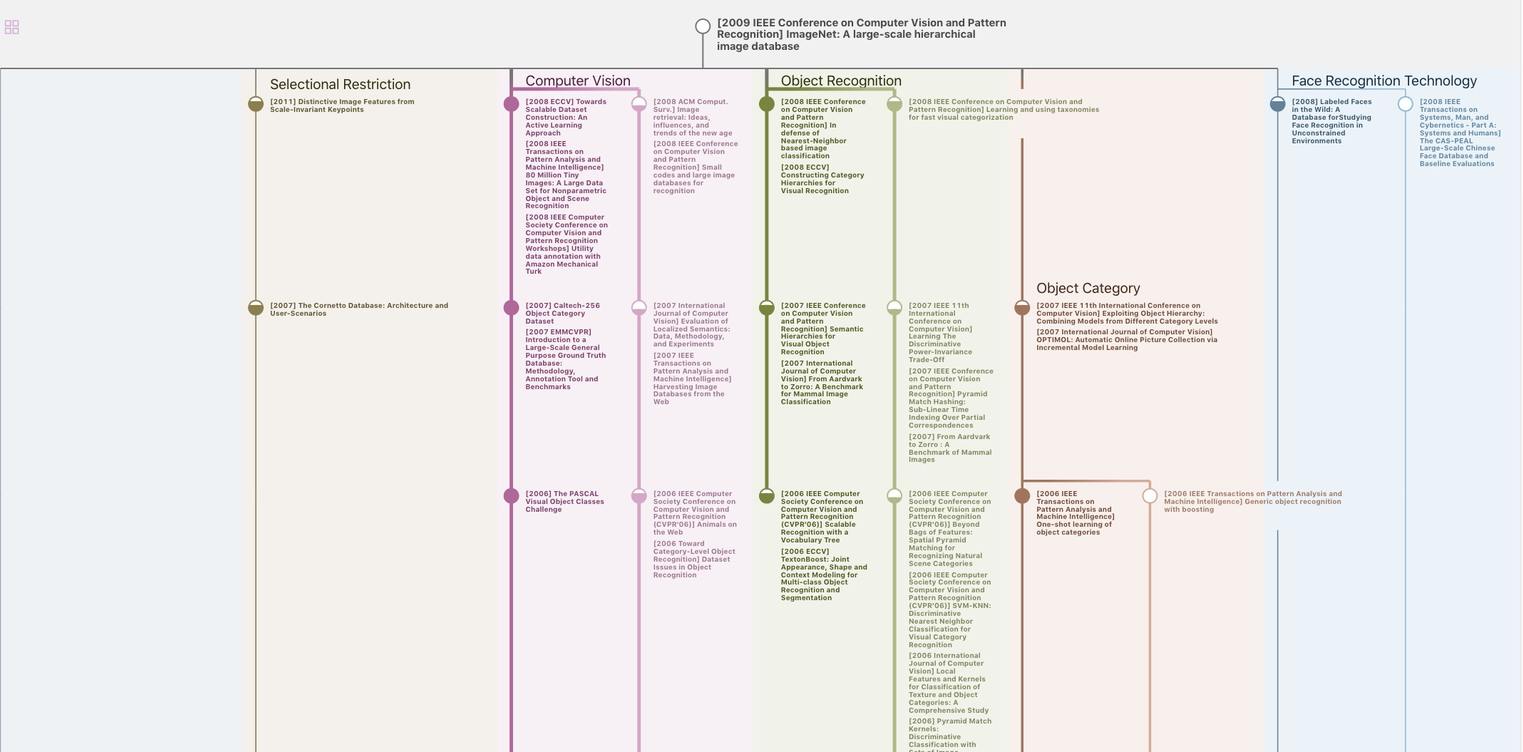
生成溯源树,研究论文发展脉络
Chat Paper
正在生成论文摘要