Spatio-Temporal Graph-based Semantic Compositional Network for Video Captioning
IEEE International Joint Conference on Neural Network (IJCNN)(2022)
摘要
Video Captioning aims to generate natural language descriptions for given videos and is one of the challenging problems in computer vision's high-level understanding tasks. Existing methods are relatively lacking in the mining of object-level spatio-temporal relationships, which is important for generating captions with accurate object information. In this paper, we improve the existing SCN-LSTM method from the perspective of modeling spatio-temporal relationships and propose the Spatio-Temporal Graph-based Semantic Compositional Network for Video Captioning (STG-SCN). In terms of spatial-temporal relationships modeling, we propose the Spatial Relation Graph (SRG) and the Temporal Relation Graph (TRG) based on the Graph Attention Network, respectively. SRG is employed to establish the spatial relationships between spatially Neighboring objects within each keyframe conditioned on their correlation with the current keyframe. TRG is used to model the temporal relationship between all the objects at different time steps and incorporates the object-level information into frame-level features. Based on the proposed Semantics Guided Decoder, visual representations enhanced by object-level information are dynamically fused with high-level semantic concepts to generate captions that not only consider the global visual content but also have stronger language expressiveness. Extended experiments show that our proposed method achieves significant performance gains on Microsoft Video Description (MSVD) and Microsoft Research Video-to-Text (MSR-VTT) datasets, outperforming existing methods.
更多查看译文
关键词
Graph neural networks,semantic compositional networks,video captioning
AI 理解论文
溯源树
样例
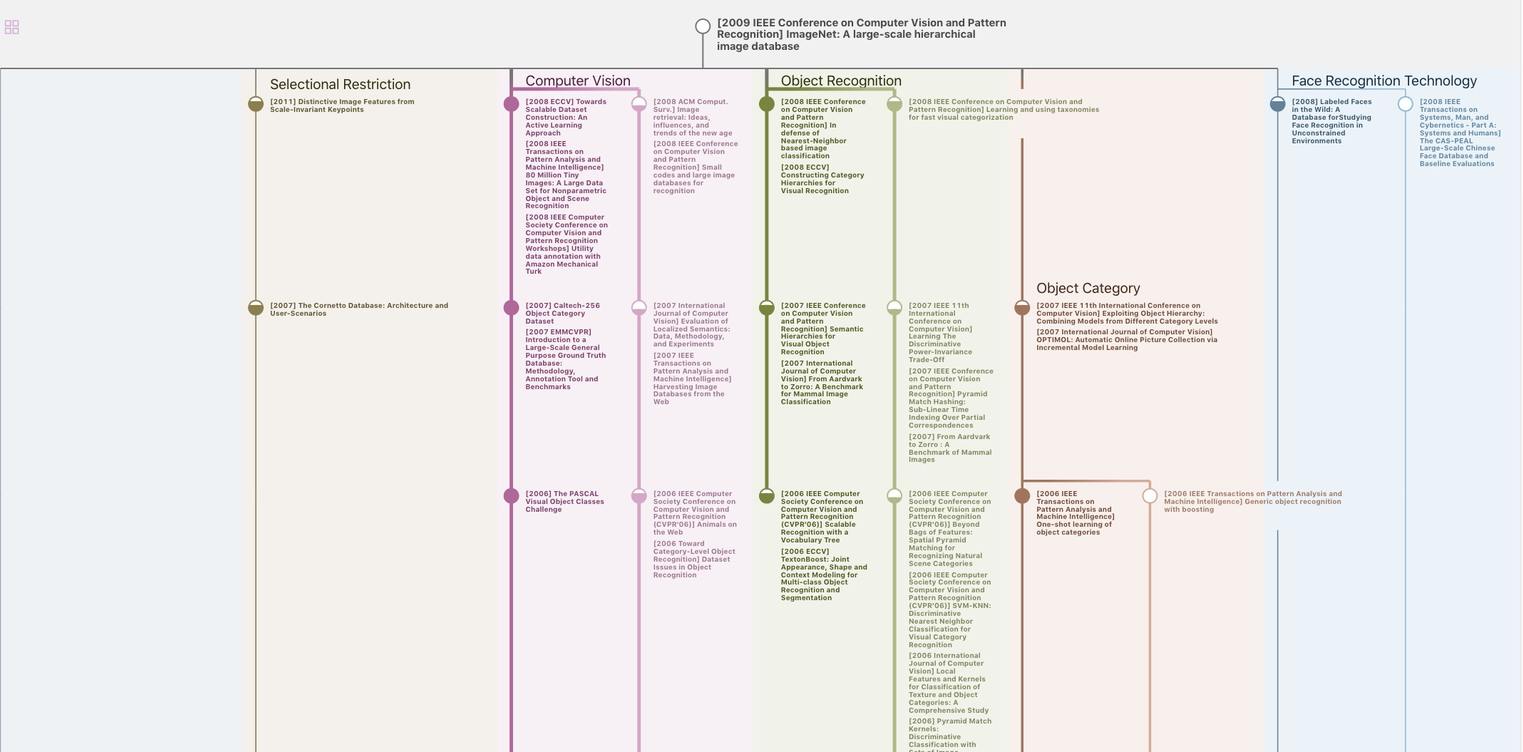
生成溯源树,研究论文发展脉络
Chat Paper
正在生成论文摘要