A Causal Network Construction Algorithm Based on Partial Rank Correlation on Time Series
2022 International Joint Conference on Neural Networks (IJCNN)(2022)
摘要
Identifying causal relationships from observational time-series data is a key problem in dealing with complex dynamical systems such as in the industrial or natural climate fields. Data-driven causal network construction in such systems is challenging since data sets are often high-dimensional and nonlinear. In response to this challenge, this paper combines partial rank correlation coefficients and proposes a new structure learning algorithm, TS-PRCS, suitable for time-series causal network models. In this article, we mainly make three contributions. First, we proved that partial rank correlation can be used as a standard of independence tests. Second, we combined partial rank correlation with constraint-based causality discovery methods, and proposed a causal network discovery algorithm (TS-PRCS) on time-series data based on partial rank correlation. Finally, the effectiveness of the algorithm is proven in experiments on time-series data generated by a time-series causal network model. Compared with an existing algorithm, the proposed algorithm achieves better results on high-dimensional and nonlinear data systems, and it also demonstrates good time performance. In particular, the algorithm has been applied to real data generated by a power plant. Experiments show that our method improves the ability to detect causality on time-series data, and further promotes the development of the field of causal network construction on time-series data.
更多查看译文
关键词
Time-series,Causal Discovery,Partial Rank Correlation
AI 理解论文
溯源树
样例
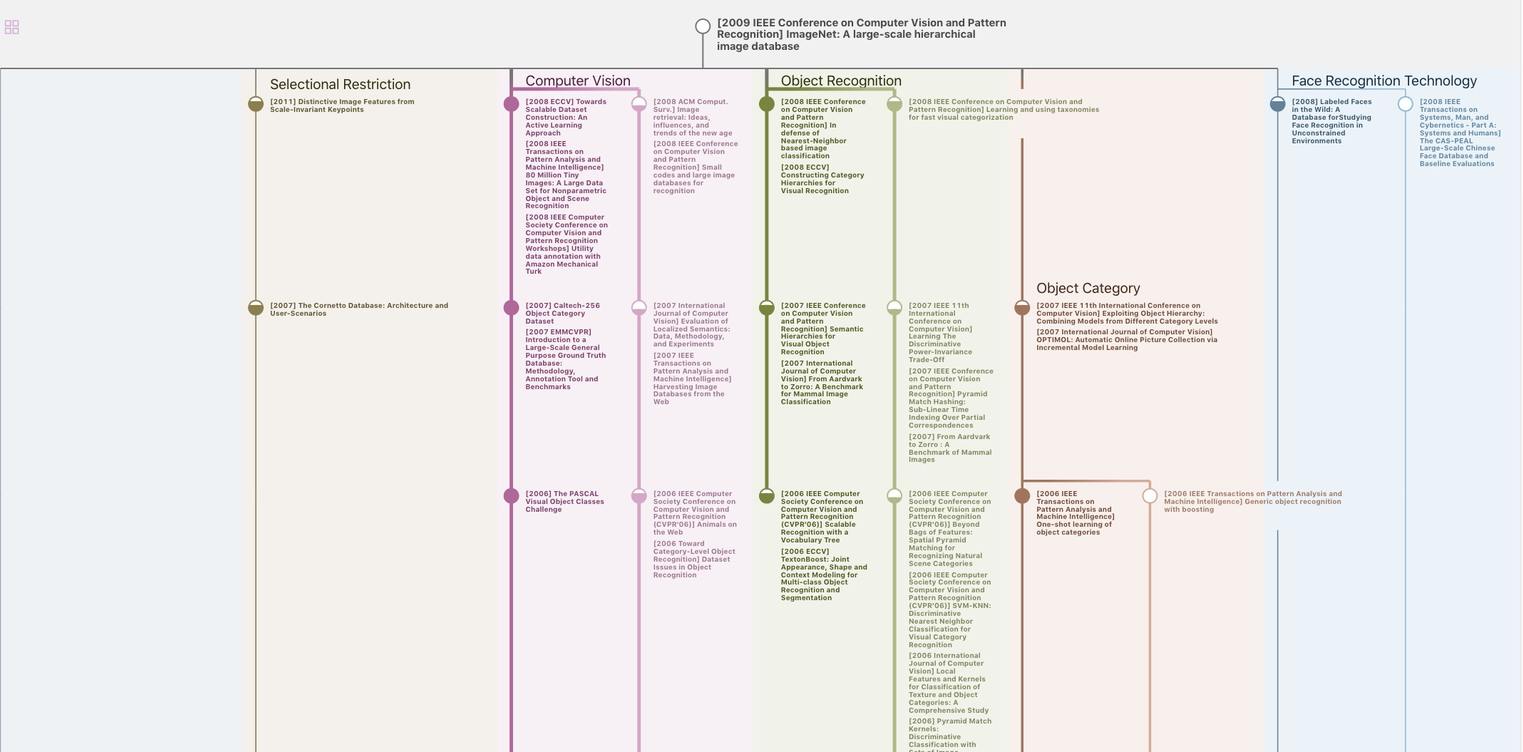
生成溯源树,研究论文发展脉络
Chat Paper
正在生成论文摘要