Complex Question Answering over Incomplete Knowledge Graph as N-ary Link Prediction
2022 International Joint Conference on Neural Networks (IJCNN)(2022)
摘要
The Question Answering over Knowledge Graph (KGQA) task seeks entities (answers) from the Knowledge Graph (KG) in order to answer natural language questions. In practice, KG is often incomplete, with numerous missing links and nodes. With such an incomplete KG, it is tricky to use the semantics inside the KG to get the golden answers, particularly for complex questions. Some current efforts concentrate on using external corpora to overcome KG sparsity; however, identifying and obtaining the corpora is challenging. Other types of work aim to leverage the pre-trained embeddings to resolve the issue but perform slightly worse on complex questions involving numerous triple facts in KG. To address the aforementioned problems, we present a framework CAPKGQA, which transforms Complex KGQA into an n-Ary link Prediction task capable of explicitly modeling complex questions. Furthermore, previous methods also suffer from incomplete KG throughout the candidate answer generation phase. Therefore, we devise an embedding-based retrieval strategy to extract more reliable candidate answers from incomplete KG. Extensive experiments reveal that our approach beats the state-of-the-art models on incomplete and complex KGQA tasks by a significant margin.
更多查看译文
关键词
question answer,knowledge graph,link prediction,neural network
AI 理解论文
溯源树
样例
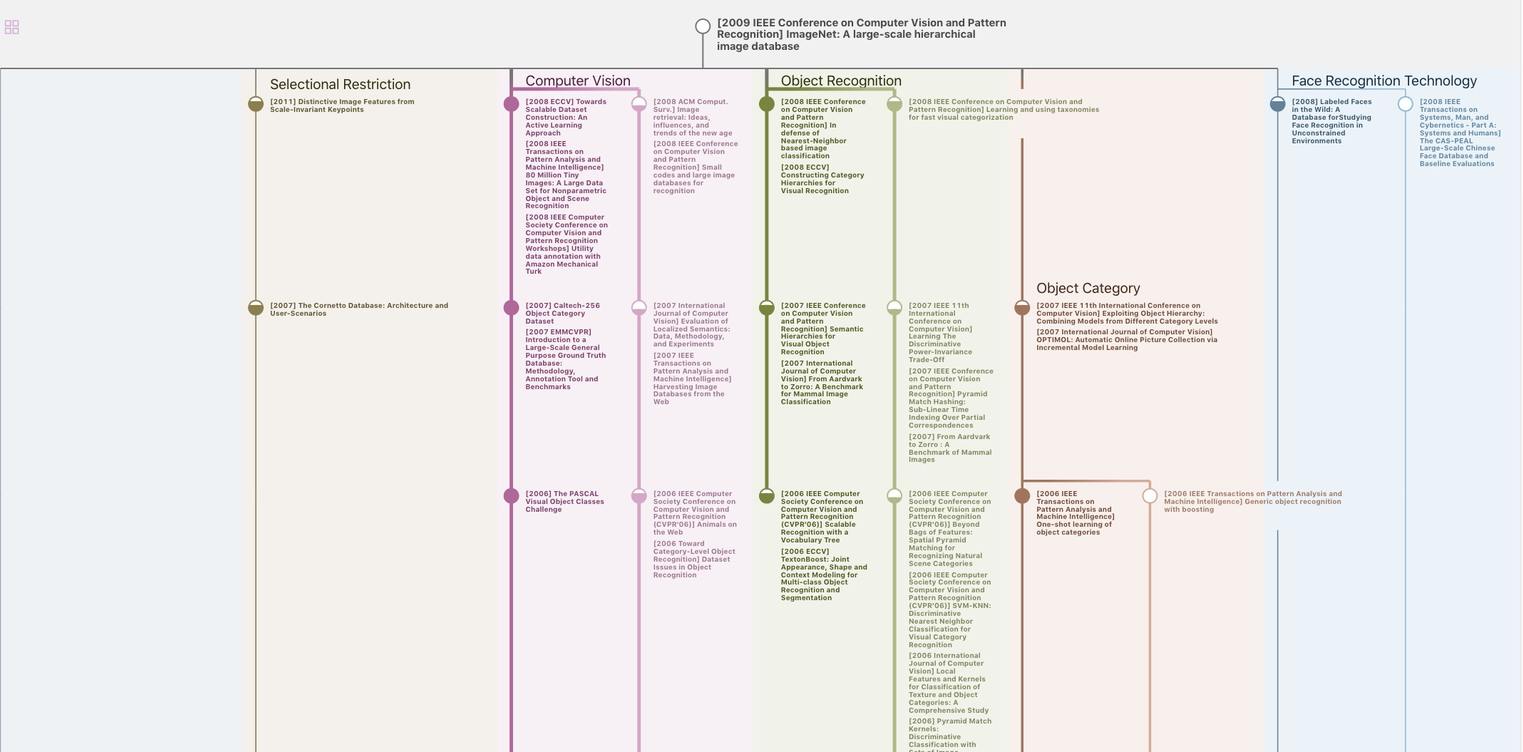
生成溯源树,研究论文发展脉络
Chat Paper
正在生成论文摘要