A Comparison Between Convolutional and Transformer Architectures for Speech Emotion Recognition
IEEE International Joint Conference on Neural Network (IJCNN)(2022)
摘要
Creating speech emotion recognition models comparable to the capability of how humans recognise emotions is a long-standing challenge in the field of speech technology with many potential commercial applications. As transformer-based architectures have recently become the state-of-the-art for many natural language processing related applications, this paper investigates their suitability for acoustic emotion recognition and compares them to the well-known AlexNet convolutional approach. This comparison is made using several publicly available speech emotion corpora. Experimental results demonstrate the efficacy of the different architectural approaches for particular emotions. The results show that the transformer-based models outperform their convolutional counterparts yielding F1-scores in the range [7033 %, 75.76 %1. This paper further provides insights via dimensionality reduction analysis of output layer activations in both architectures and reveals significantly improved clustering in transformer-based models whilst highlighting the nuances with regard to the separability of different emotion classes.
更多查看译文
关键词
speech emotion recognition,transformers,wav2vec2,convolutional neural networks,alexnet,transfer learning,mel spectrograms
AI 理解论文
溯源树
样例
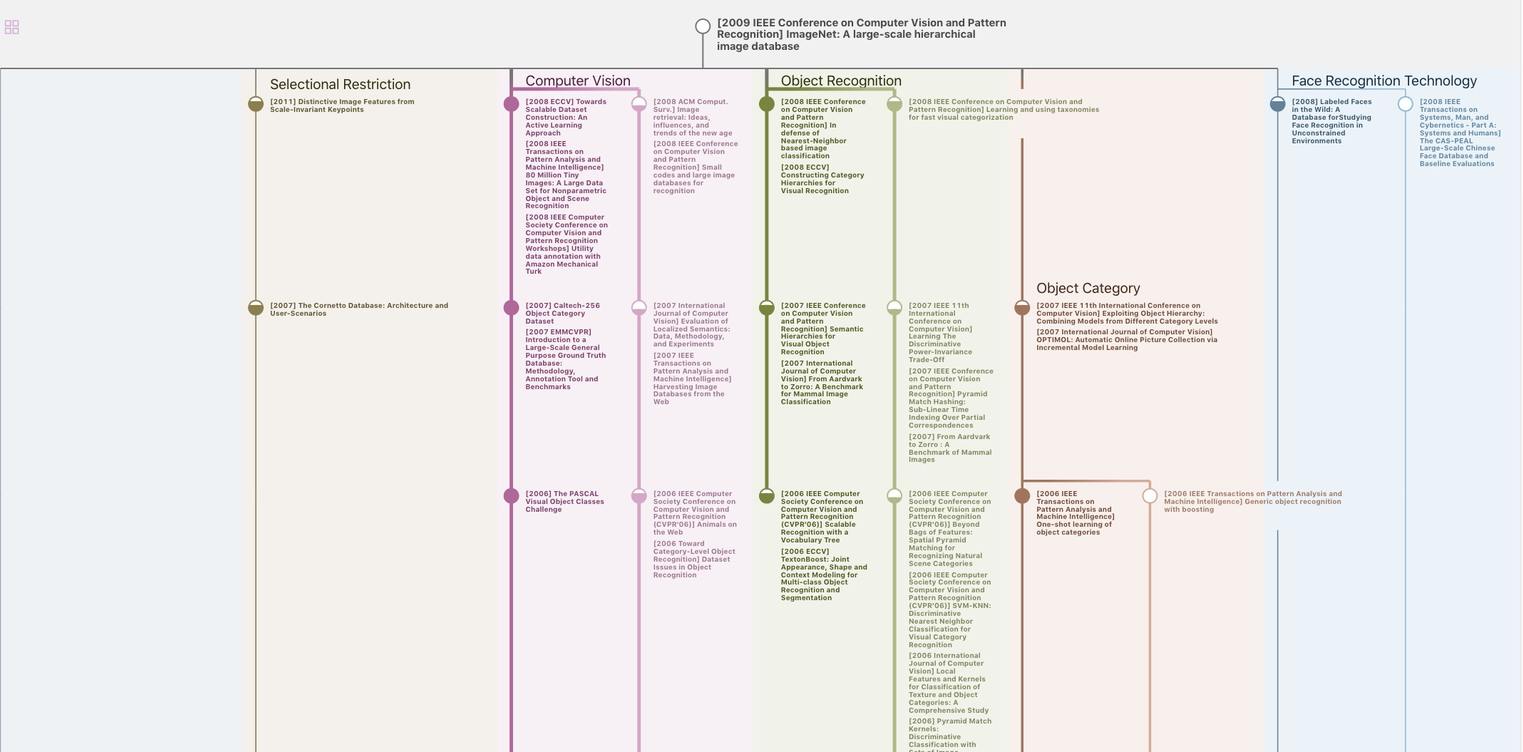
生成溯源树,研究论文发展脉络
Chat Paper
正在生成论文摘要