Calibration of the Multiple Choice Machine Reading Comprehension
2022 International Joint Conference on Neural Networks (IJCNN)(2022)
摘要
Prediction calibration devotes to making the model produce correct prediction probability, corresponding with the model's empirical measurement (i.e., the accuracy on benchmark). It plays a crucial role in the Multiple-Choice based Machine Reading Comprehension (MCRC) task. Once the model gives the wrong candidate answer with a high probability, users or downstream applications will not trust the model easily. However, few works pay attention to the prediction calibration of MCRC models. In this paper, we study the prediction calibration of the MCRC models and introduce the self-supervised target label softening (SS-TLS) training method to develop a well-calibrated MRC model while improving its performance. Specifically, the proposed SS-TLS method softens the target label to train the MCRC model instead of the standard cross-entropy objective. It employs the self-supervised confidence signal to monitor the soften scale adaptively at the instance level. Experimental results on several multiple-Choice style MRC datasets illustrate that the proposed method can improve both model prediction calibration and performance.
更多查看译文
关键词
machine reading comprehension,pre-trained language models,calibration,predictive uncertainty
AI 理解论文
溯源树
样例
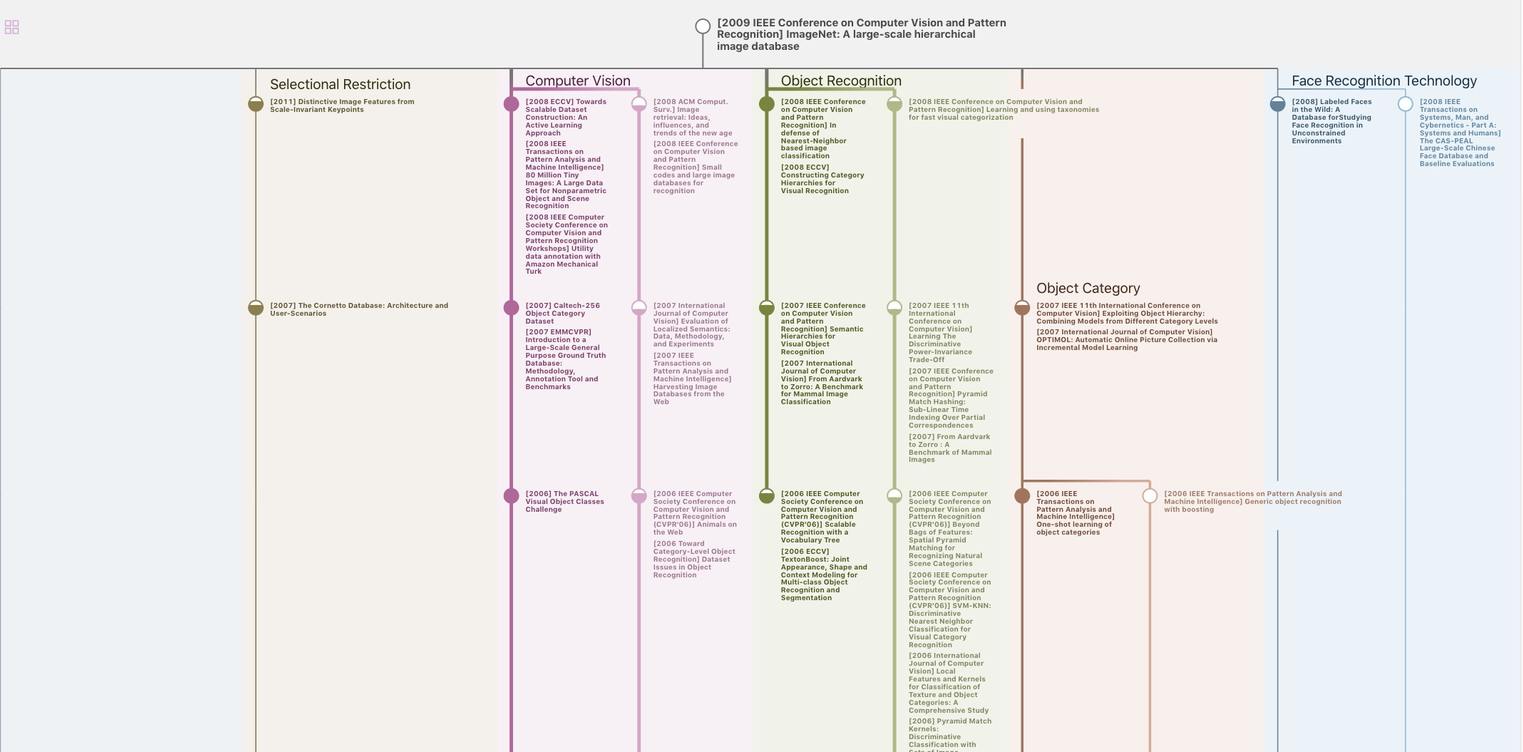
生成溯源树,研究论文发展脉络
Chat Paper
正在生成论文摘要