Two-Stage Deep Learning Approach for Speech Enhancement and Reconstruction in The Frequency and Time Domains
2022 International Joint Conference on Neural Networks (IJCNN)(2022)
摘要
Deep learning has recently shown promising improvement in the speech enhancement field, due to its effectiveness in eliminating noise. However, a drawback of the denoising process is the introduction of speech distortion, which negatively affects speech quality and intelligibility. In this work, we propose a deep convolutional denoising autoencoder-based speech enhancement network that is designed to have an encoder deeper than the decoder, to improve performance and decrease complexity. Furthermore, we present a two-stage learning approach, in which denoising is performed in the first frequency domain stage using magnitude spectrum as a training target; while, in the second stage, further denoising and speech reconstruction are performed in the time domain. Results show that our architecture achieves 0.22 improvement in the overall predicted mean opinion score (Covl) over state of the art speech enhancement architectures, using the Valentini dataset benchmark. Moreover, the architecture was trained using a larger dataset and tested using a mismatched test corpus, to achieve 0.7 and 6.35% improvement in Perceptual Evaluation of Speech Quality (PESQ) and Short Time Objective Intelligibility (STOI) scores, respectively, compared to the noisy speech.
更多查看译文
关键词
Deep learning,denoising autoencoders,speech enhancement,speech features,speech reconstruction
AI 理解论文
溯源树
样例
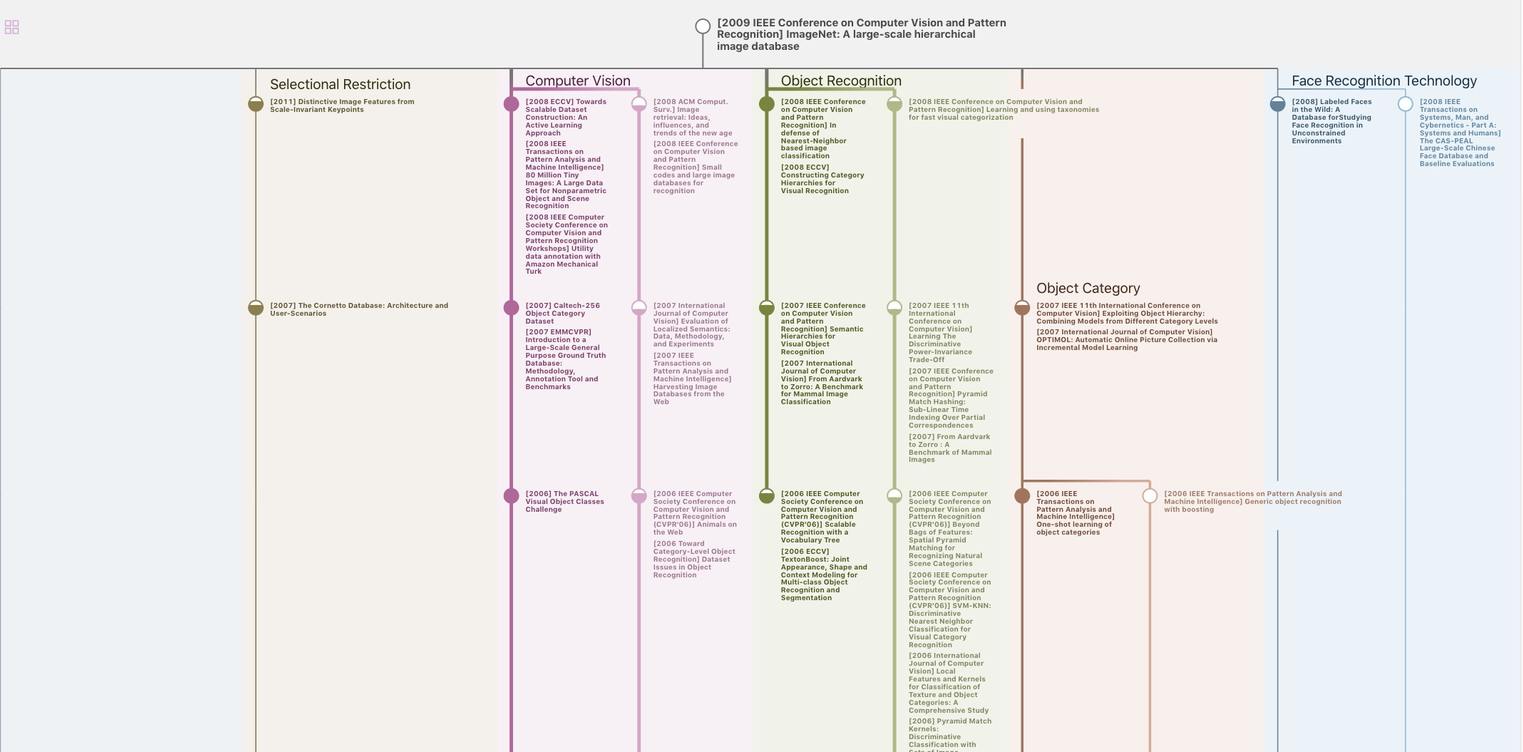
生成溯源树,研究论文发展脉络
Chat Paper
正在生成论文摘要