Exploring Pixel Alignment on Shallow Feature for Weakly Supervised Object Localization
IEEE International Joint Conference on Neural Network (IJCNN)(2022)
摘要
Weakly supervised object localization (WSOL) aims to cover the entire target object only under the image-level supervision. Most WSOL methods are stuck in mining the CAMs (class activation maps) of deep semantic features for they only focus on limited discriminative regions playing key role in classification. Recently, a new paradigm has emerged by localizing objects using the low-level feature through two stages. Existing two-stages methods usually train a classification network first to yield CAMs as pseudo labels to guide the learning of segment network, yet it does not consider the activations with more background noise or less discriminative area. In this paper, we propose a Pixel Alignment strategy to refine the object localization by improving the shallow-feature based CAMs generator with the joint supervision of pseudo-label mask, classification evaluation, and absolution size constraint on the activation map. More specifically, we utilize the class-specific pixel gradient to achieve a robust activation pseudo mask to background noise, which further supervises the activation generator with confident foreground and background regions. We also adapt a post-processing to excavate the target region in the conflict area (i.e., the non-overlap area of CAMs and the activations). Extensive experiments on CUB-2002011 and ILSVRC datasets indicate that our method outperforms the state-of-the-art among the two-stage works.
更多查看译文
关键词
Weakly Supervised Object Localization,Class Activation Maps,Two-stage,Low-level Feature
AI 理解论文
溯源树
样例
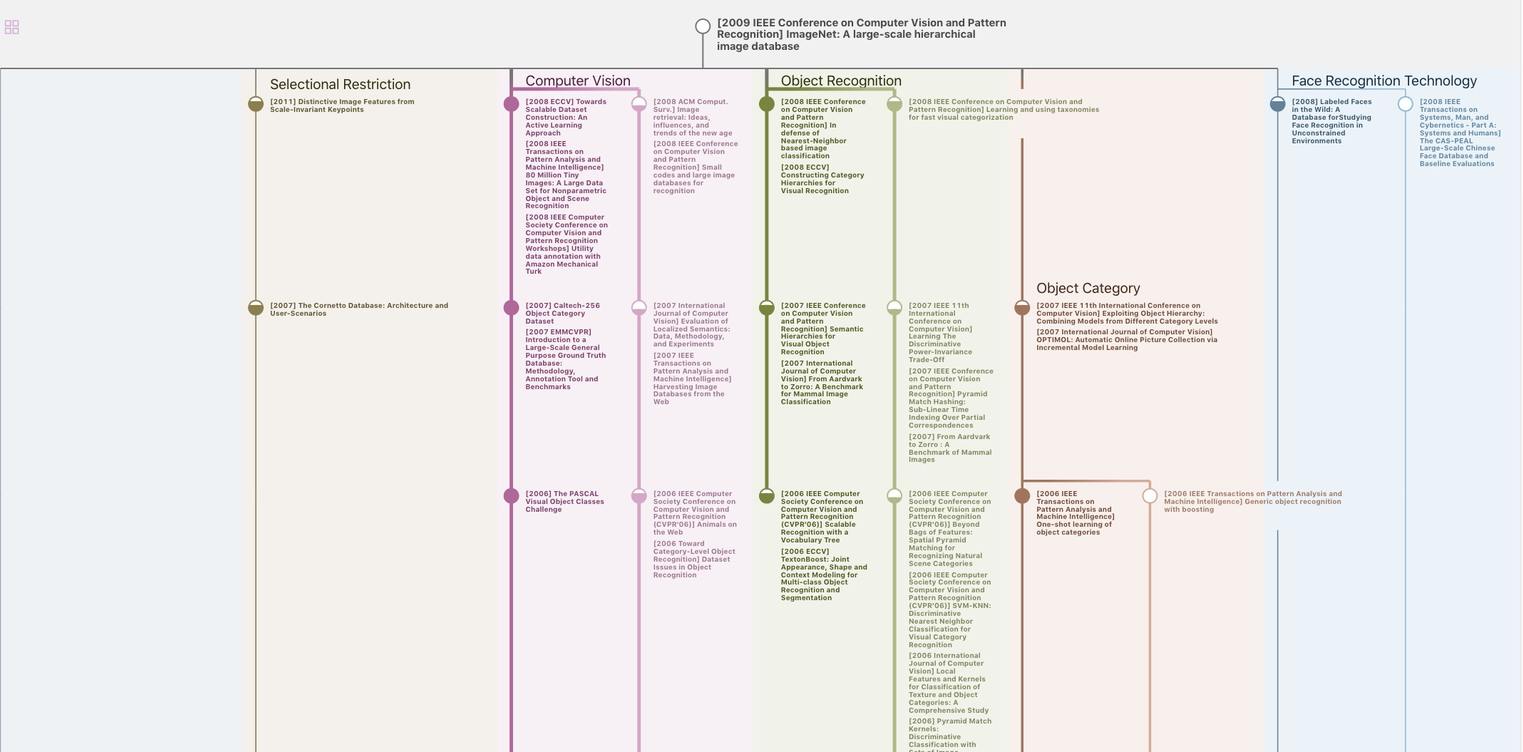
生成溯源树,研究论文发展脉络
Chat Paper
正在生成论文摘要