Hformer: Hybrid convolutional neural network transformer network for fringe order prediction in phase unwrapping of fringe projection
Optical Engineering(2022)
摘要
Deep learning based on convolutional neural network (CNN) has attracted more and more attention in phase unwrapping of fringe projection three-dimensional (3D) measurement. However, due to the inherent limitations of convolutional operator, it is difficult to accurately determine the fringe order in wrapped phase patterns that rely on continuity and globality. To attack this problem, in this paper we develop a hybrid CNN-transformer model (Hformer) dedicated to phase unwrapping via fringe order prediction. The proposed Hformer model has a hybrid CNN-transformer architecture that is mainly composed of backbone, encoder, and decoder to take advantage of both CNN and transformer. Backbone is used as a wrapped phase pattern feature extractor. Encoder and decoder with cross attention are designed to enhance global dependency for the fringe order prediction. Experimental results show that the proposed Hformer model achieves better performance in fringe order prediction compared with the CNN models such as U-Net and DCNN. Our work opens an alternative way to the CNN-dominated deep learning phase unwrapping of fringe projection 3D measurement. (C) 2022 Society of PhotoOptical Instrumentation Engineers (SPIE)
更多查看译文
关键词
fringe order, deep learning, transformer, phase unwrapping, Hformer
AI 理解论文
溯源树
样例
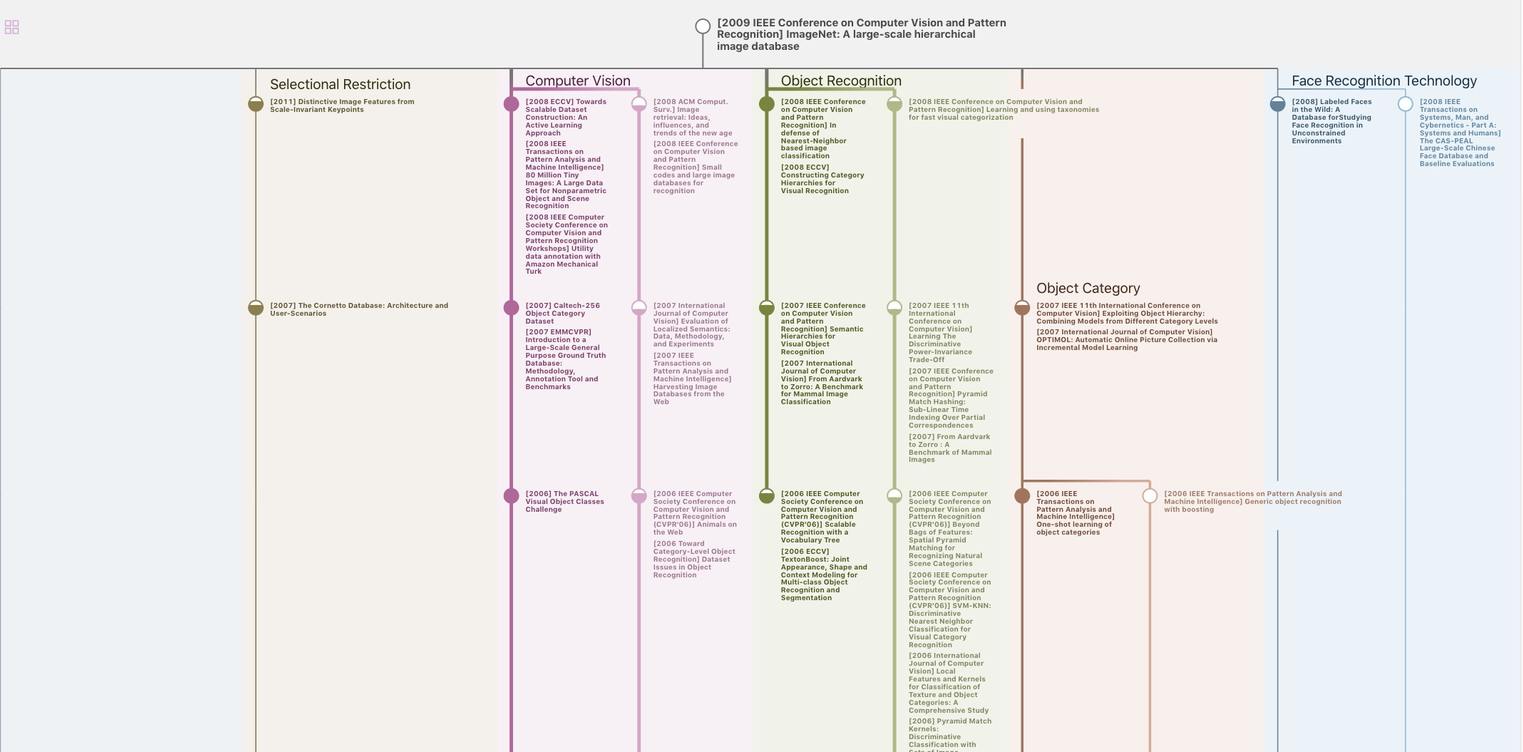
生成溯源树,研究论文发展脉络
Chat Paper
正在生成论文摘要