Lightweight Industrial Image Classifier based on Federated Few-Shot Learning
IEEE Transactions on Industrial Informatics(2022)
摘要
Image classification using convolutional neural networks (CNNs) is critical for broader industrial applications like defect detection. To protect sensitive data during the industrial process, increasing institutions are highly interested in training CNN classifiers collaboratively with federated learning (FL). However, the existing FL solutions cannot address the sample deficiency and heterogeneous learning resource issues at different practical institutions. In this article, we present a federated lightweight relation network (FLRN), a lightweight industrial image classifier based on our federated few-shot learning (FFSL) architecture. Results of extensive experiments considering different real-world FFSL scenarios indicate that, unlike the state-of-the-art few-shot learning method relation network (RN), the FLRN performs well on not only FL participants with mutually isolated classes of samples but also external institutions with limited samples from unseen classes. Compared to the RN with the predominating FedAvg-based FL deployment, the FLRN manages to achieve as low as
$\text{29.6}\times$
less client–cloud communication,
$\text{5.2}\times$
less computation, and
$\text{22.0}\times$
less storage costs of clients.
更多查看译文
关键词
learning,industrial,image,few-shot
AI 理解论文
溯源树
样例
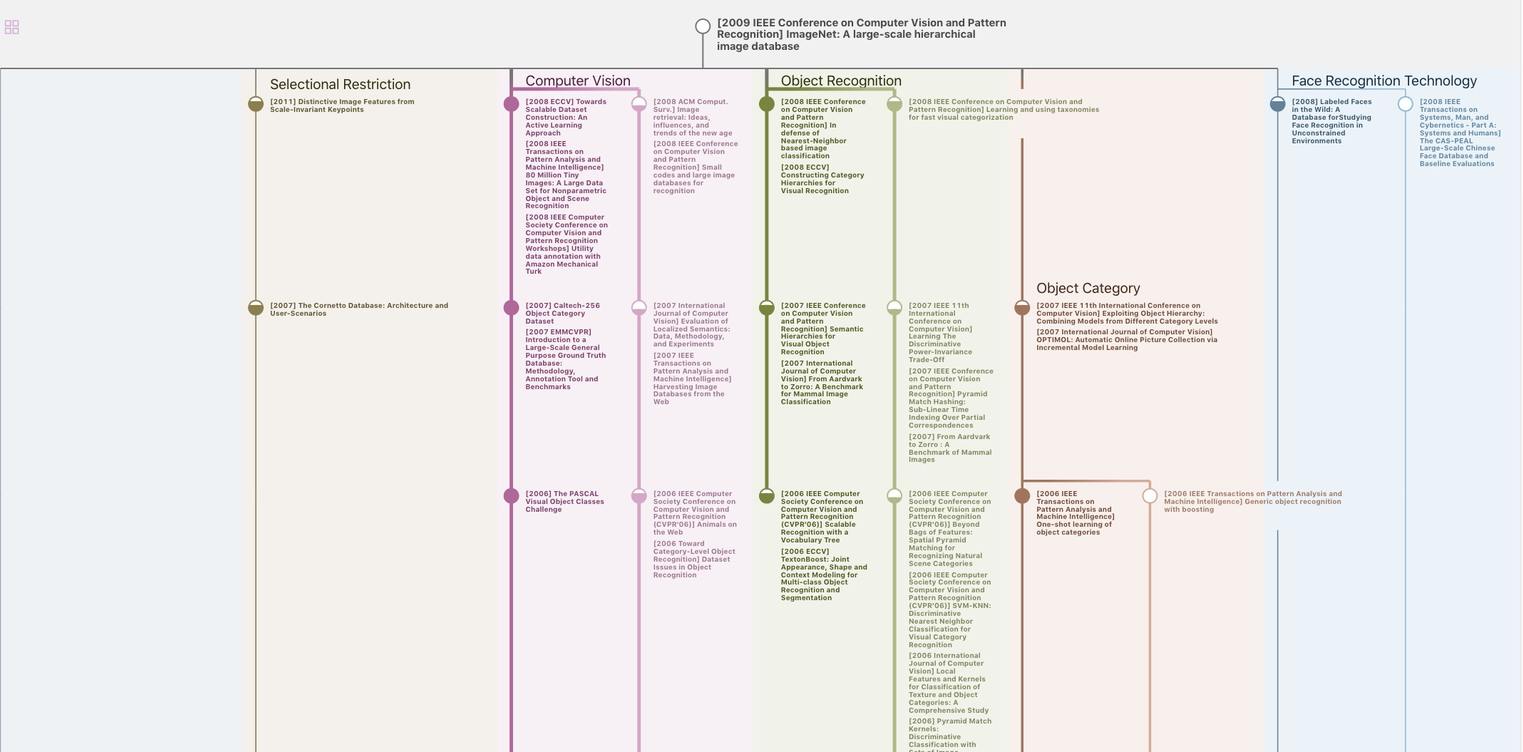
生成溯源树,研究论文发展脉络
Chat Paper
正在生成论文摘要