Finite‐level uniformly quantized learning control with random data dropouts
International Journal of Robust and Nonlinear Control(2022)
摘要
In this study, a quantized iterative learning control method with an encoding-decoding mechanism is investigated for networked control systems with constrained transmission bandwidths and random data dropouts at both the measurement and actuator sides. The intermittent update principle is used to address the problem of data asynchronism caused by two-sided data dropouts. Then, a new "process" concept is introduced for the convergence analysis. The input sequence is guaranteed to achieve asymptotic zero-error convergence to the unknown desired input for the given reference through an appropriate selection of scaling sequences. Furthermore, the quantization errors are shown bounded, where the upper bounds of the quantization level are precisely determined for selecting a finite-level uniform quantizer. To verify the proposed scheme, an example of a permanent magnet linear motor is simulated.
更多查看译文
关键词
encoding and decoding mechanism,iterative learning control,random data dropouts,uniform quantizer
AI 理解论文
溯源树
样例
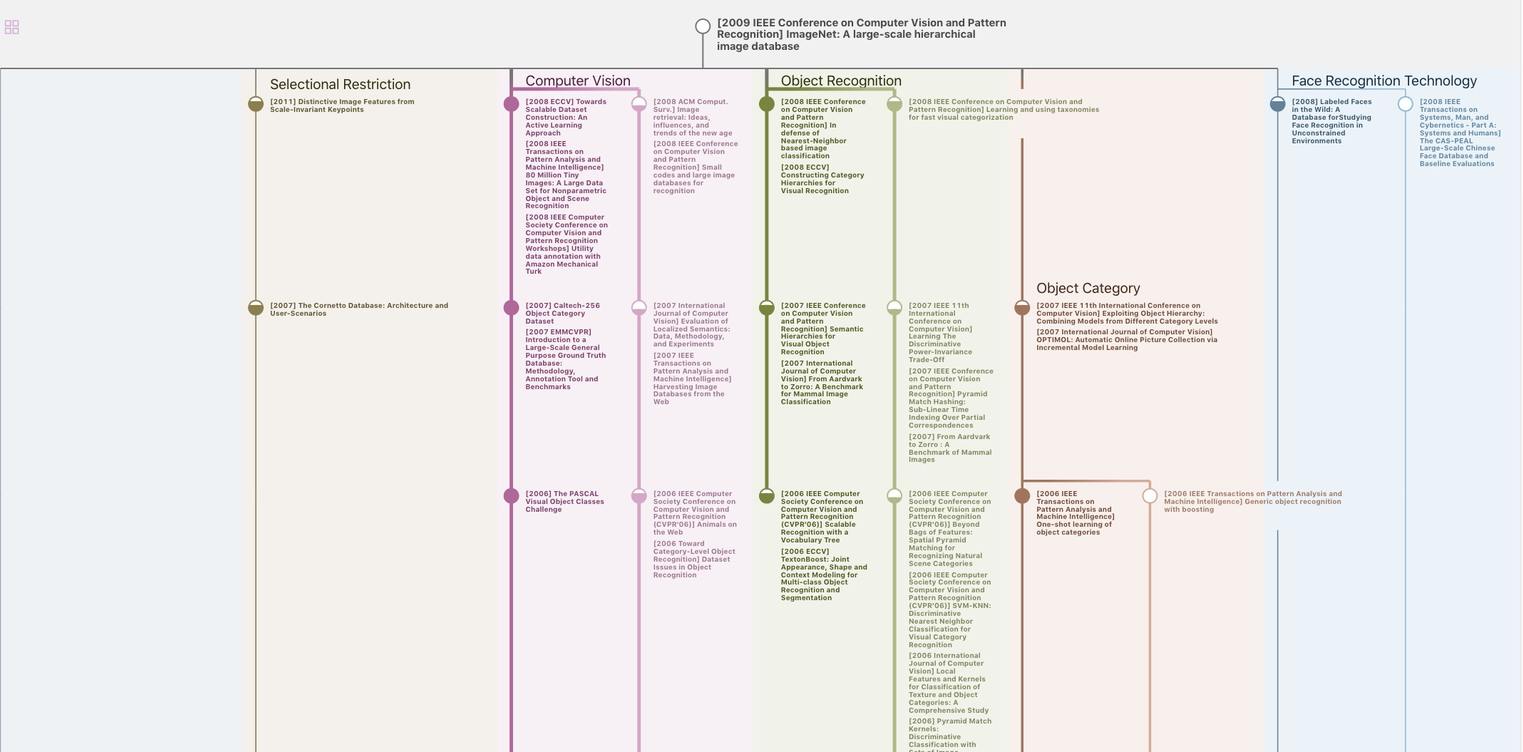
生成溯源树,研究论文发展脉络
Chat Paper
正在生成论文摘要