Denoising Diffusion Probabilistic Models for Styled Walking Synthesis
arxiv(2022)
摘要
Generating realistic motions for digital humans is time-consuming for many graphics applications. Data-driven motion synthesis approaches have seen solid progress in recent years through deep generative models. These results offer high-quality motions but typically suffer in motion style diversity. For the first time, we propose a framework using the denoising diffusion probabilistic model (DDPM) to synthesize styled human motions, integrating two tasks into one pipeline with increased style diversity compared with traditional motion synthesis methods. Experimental results show that our system can generate high-quality and diverse walking motions.
更多查看译文
关键词
diffusion probabilistic models,synthesis
AI 理解论文
溯源树
样例
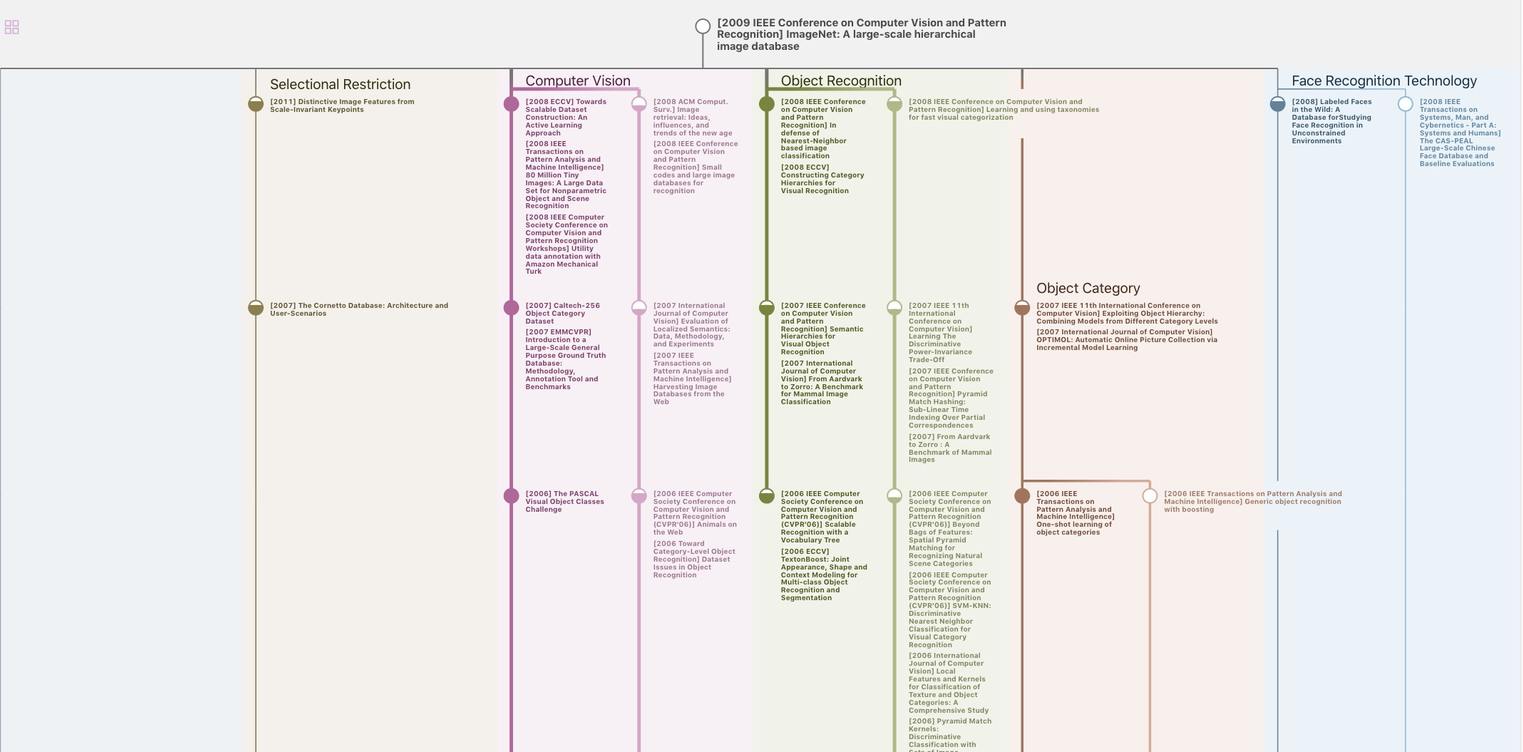
生成溯源树,研究论文发展脉络
Chat Paper
正在生成论文摘要