Optimal Stopping with Gaussian Processes
3RD ACM INTERNATIONAL CONFERENCE ON AI IN FINANCE, ICAIF 2022(2022)
摘要
We propose a novel group of Gaussian Process based algorithms for fast approximate optimal stopping of time series with specific applications to financial markets. We show that structural properties commonly exhibited by financial time series (e.g., the tendency to mean-revert) allow the use of Gaussian and Deep Gaussian Process models that further enable us to analytically evaluate optimal stopping value functions and policies. We additionally quantify uncertainty in the value function by propagating the price model through the optimal stopping analysis. We compare and contrast our proposed methods against a sampling-based method, as well as a deep learning based benchmark that is currently considered the state-of-the-art in the literature. We show that our family of algorithms outperforms benchmarks on three historical time series datasets that include intra-day and end-of-day equity asset prices as well as the daily US treasury yield curve rates.
更多查看译文
关键词
Bayesian Modeling,Optimal Stopping,Gaussian Processes
AI 理解论文
溯源树
样例
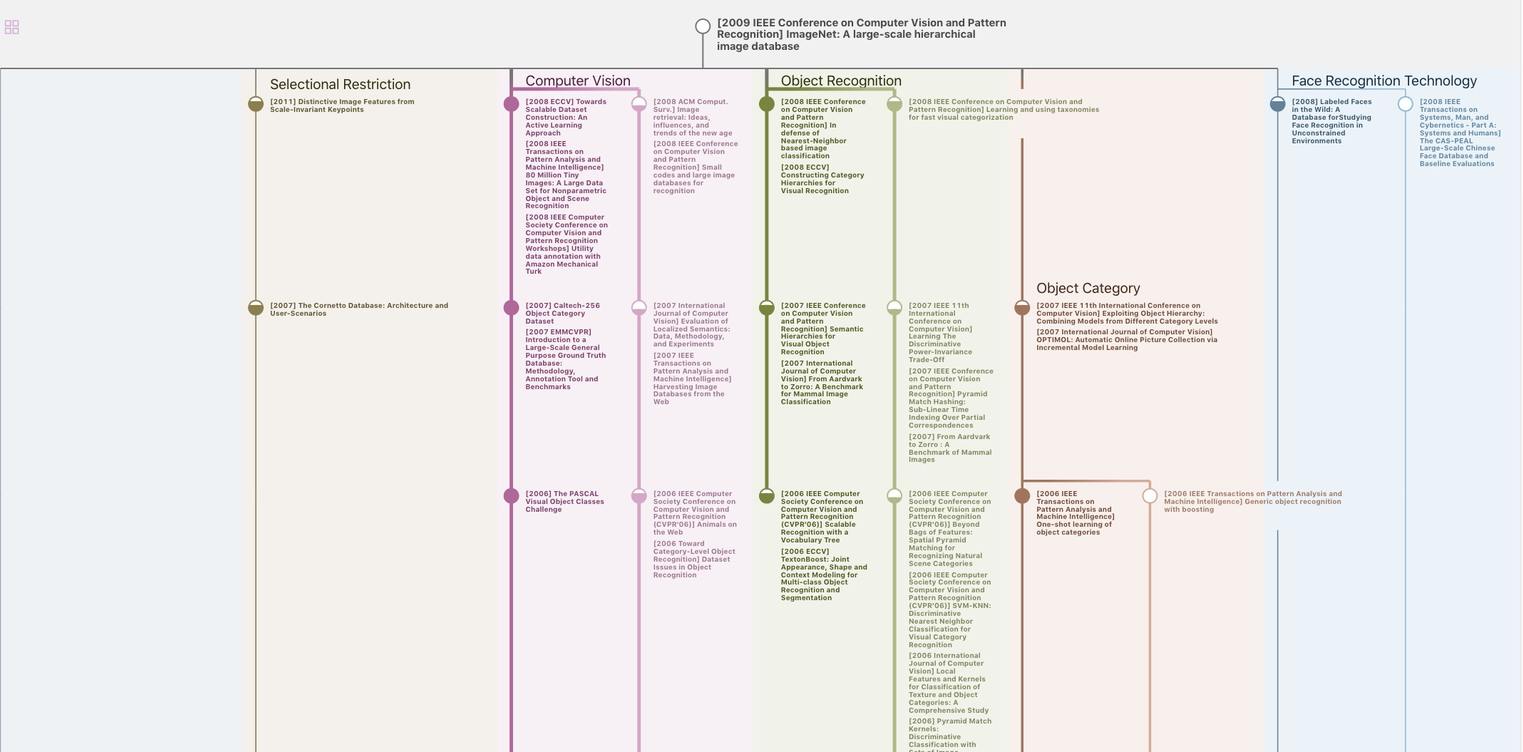
生成溯源树,研究论文发展脉络
Chat Paper
正在生成论文摘要