Hyper-Representations as Generative Models: Sampling Unseen Neural Network Weights
NeurIPS 2022(2022)
摘要
Learning representations of neural network weights given a model zoo is an emerging and challenging area with many potential applications from model inspection, to neural architecture search or knowledge distillation. Recently, an autoencoder trained on a model zoo was able to learn a hyper-representation, which captures intrinsic and extrinsic properties of the models in the zoo. In this work, we extend hyper-representations for generative use to sample new model weights. We propose layer-wise loss normalization which we demonstrate is key to generate high-performing models and several sampling methods based on the topology of hyper-representations. The models generated using our methods are diverse, performant and capable to outperform strong baselines as evaluated on several downstream tasks: initialization, ensemble sampling and transfer learning. Our results indicate the potential of knowledge aggregation from model zoos to new models via hyper-representations thereby paving the avenue for novel research directions.
更多查看译文
关键词
Weight Generation,Representation Learning,Model Zoo,Hyper-Representations,Ensembling
AI 理解论文
溯源树
样例
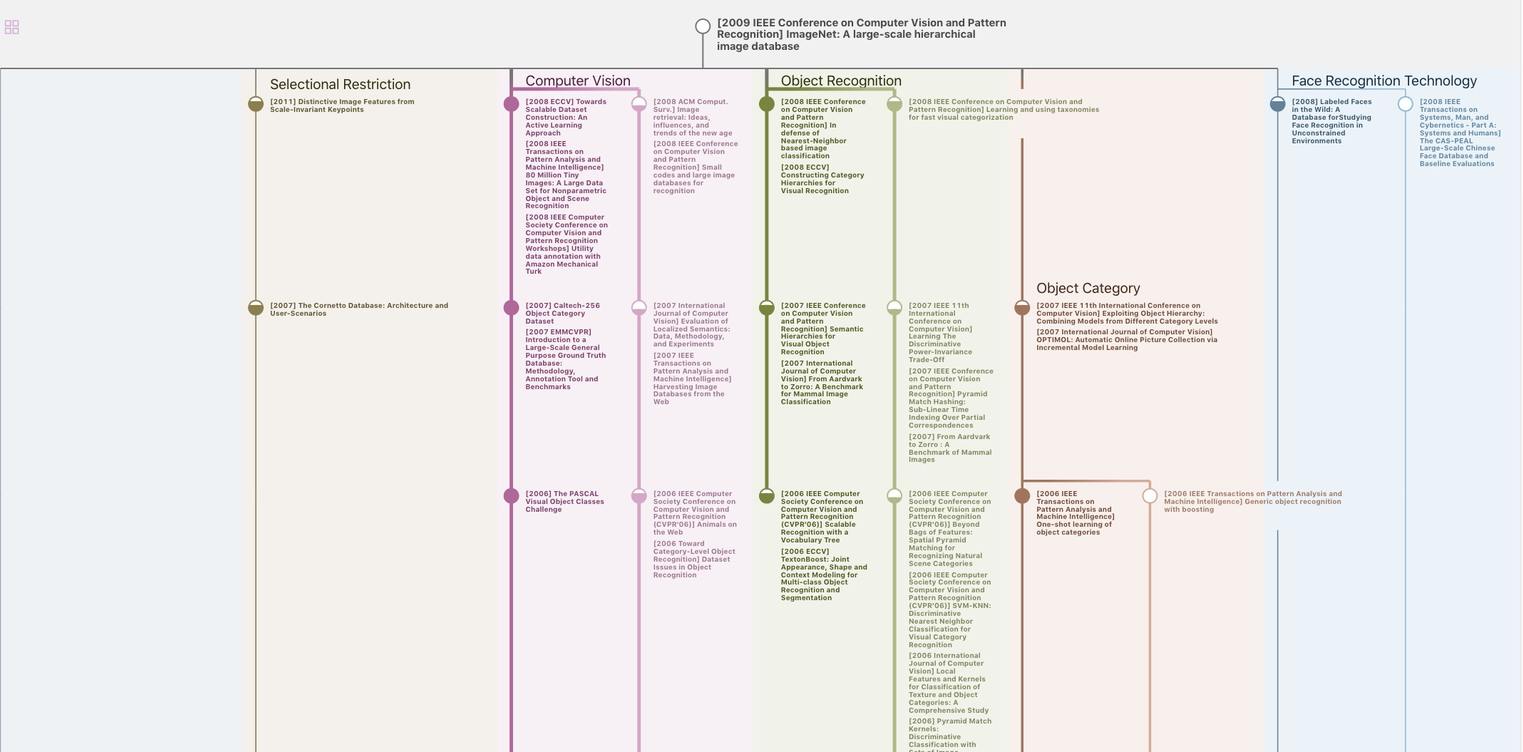
生成溯源树,研究论文发展脉络
Chat Paper
正在生成论文摘要