Bayesian Neural Network Versus Ex-Post Calibration For Prediction Uncertainty
arxiv(2022)
摘要
Probabilistic predictions from neural networks which account for predictive uncertainty during classification is crucial in many real-world and high-impact decision making settings. However, in practice most datasets are trained on non-probabilistic neural networks which by default do not capture this inherent uncertainty. This well-known problem has led to the development of post-hoc calibration procedures, such as Platt scaling (logistic), isotonic and beta calibration, which transforms the scores into well calibrated empirical probabilities. A plausible alternative to the calibration approach is to use Bayesian neural networks, which directly models a predictive distribution. Although they have been applied to images and text datasets, they have seen limited adoption in the tabular and small data regime. In this paper, we demonstrate that Bayesian neural networks yields competitive performance when compared to calibrated neural networks and conduct experiments across a wide array of datasets.
更多查看译文
关键词
uncertainty,prediction
AI 理解论文
溯源树
样例
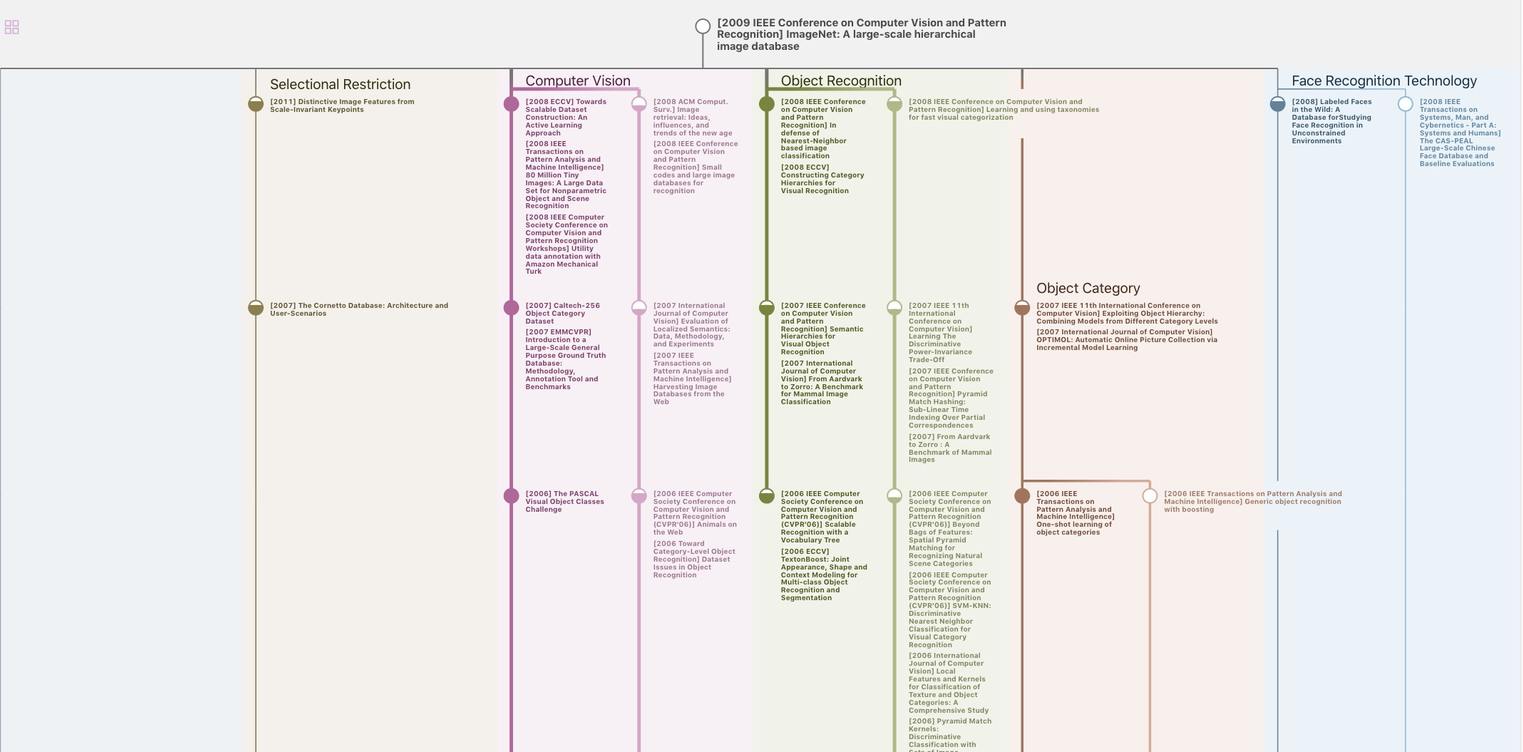
生成溯源树,研究论文发展脉络
Chat Paper
正在生成论文摘要