Intrinsic Dimensionality Estimation within Tight Localities: A Theoretical and Experimental Analysis
arxiv(2022)
摘要
Accurate estimation of Intrinsic Dimensionality (ID) is of crucial importance in many data mining and machine learning tasks, including dimensionality reduction, outlier detection, similarity search and subspace clustering. However, since their convergence generally requires sample sizes (that is, neighborhood sizes) on the order of hundreds of points, existing ID estimation methods may have only limited usefulness for applications in which the data consists of many natural groups of small size. In this paper, we propose a local ID estimation strategy stable even for `tight' localities consisting of as few as 20 sample points. The estimator applies MLE techniques over all available pairwise distances among the members of the sample, based on a recent extreme-value-theoretic model of intrinsic dimensionality, the Local Intrinsic Dimension (LID). Our experimental results show that our proposed estimation technique can achieve notably smaller variance, while maintaining comparable levels of bias, at much smaller sample sizes than state-of-the-art estimators.
更多查看译文
关键词
intrinsic dimensionality estimation,tight localities
AI 理解论文
溯源树
样例
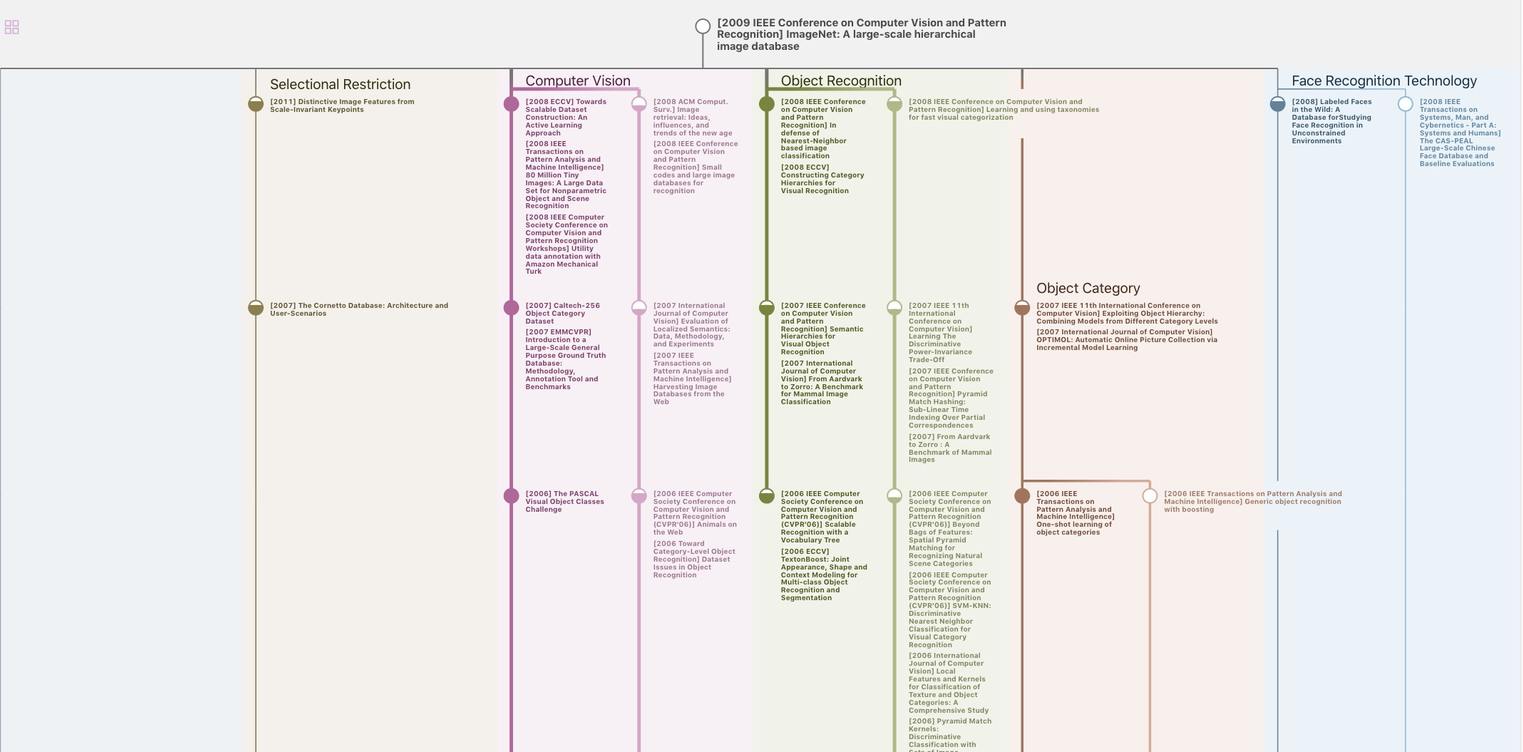
生成溯源树,研究论文发展脉络
Chat Paper
正在生成论文摘要