Multi-Dimensional Image Recovery via Fully-Connected Tensor Network Decomposition Under the Learnable Transforms
Journal of Scientific Computing(2022)
摘要
Multi-dimensional image recovery from incomplete data is a fundamental problem in data processing. Due to its advantage of capturing the correlations between any modes of the multi-dimensional image, i.e., the target tensor, the fully-connected tensor network (FCTN) decomposition has recently shown promising performance on multi-dimensional image recovery. However, FCTN decomposition suffers from computational deficiency, especially for large-scale multi-dimensional images. To address this deficiency, we propose a learnable transform-based FCTN model (termed as T-FCTN), which enjoys the remarkable advantage of FCTN decomposition with cheap computational cost. More concretely, we learn the semi-orthogonal transforms along each mode of the target tensor to project the large-scale tensor 𝒳 ∈ ℝ^I×I×⋯×I into a small-scale essential tensor ℰ ∈ ℝ^r×r×⋯×r , and then apply FCTN decomposition on the small-scale essential tensor. To tackle the proposed model, we develop an efficient proximal alternating minimization (PAM)-based algorithm with theoretical convergence guarantee. Moreover, the computational complexity of PAM for T-FCTN is 𝒪(N∑ _k=2^Nr^kR^k(N-k)+k-1+Nr^N-1R^2(N-1)+NR^3(N-1)+N∑ _k=1^Nr^kI^N-k+1) at each iteration, which is significantly lower than 𝒪(N∑ _k=2^NI^kR^k(N-k)+k-1+NI^N-1R^2(N-1)+NR^3(N-1)) of PAM for FCTN when r≪ I . Extensive numerical experiments on color videos and light field images illustrate the superiority of the proposed method over other state-of-the-art methods in terms of quality metrics, visual quality, and running time.
更多查看译文
关键词
Multi-dimensional image recovery,Fully-connected tensor network (FCTN) decomposition,Learnable transform,Proximal alternating minimization (PAM)
AI 理解论文
溯源树
样例
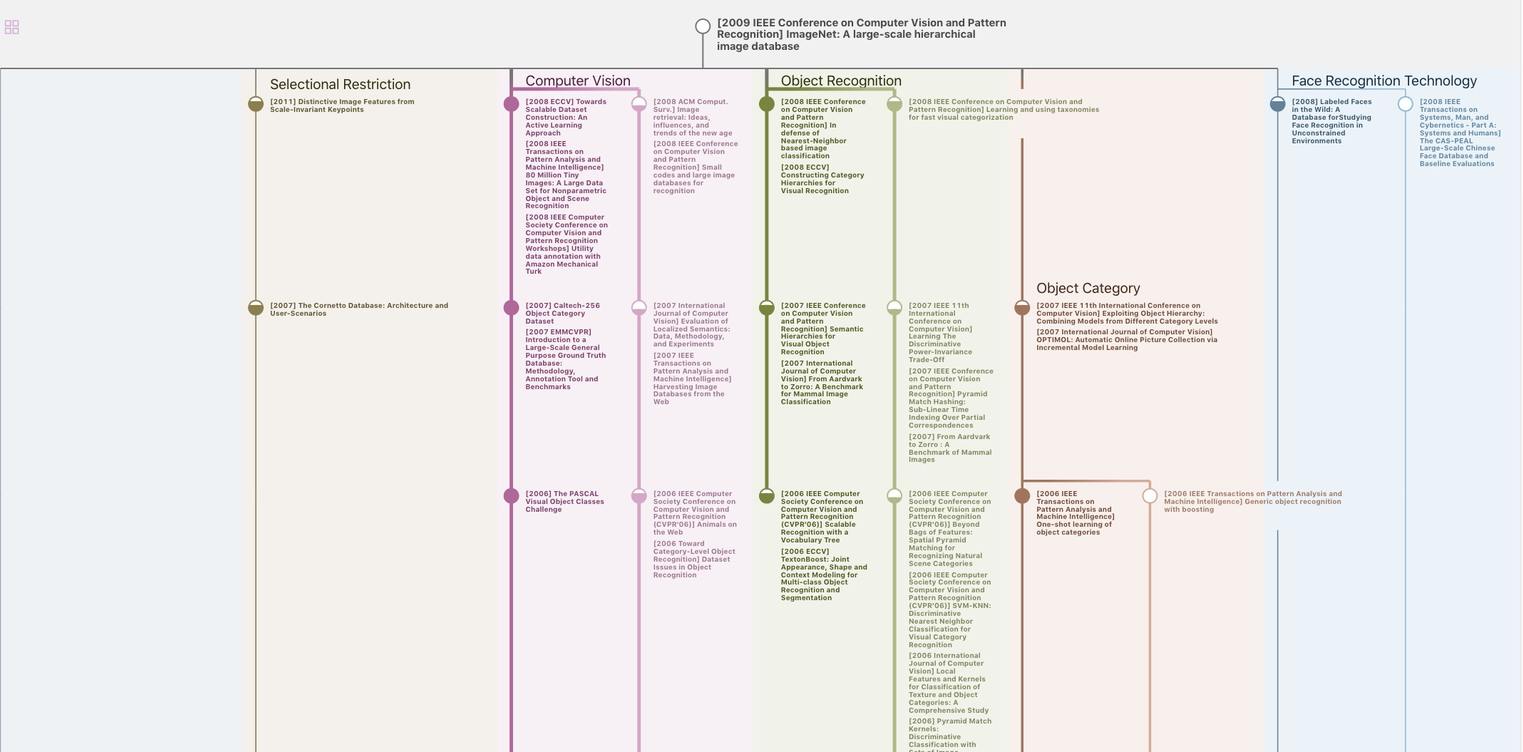
生成溯源树,研究论文发展脉络
Chat Paper
正在生成论文摘要