Accelerated T2-weighted MRI of the liver at 3 T using a single-shot technique with deep learning-based image reconstruction: impact on the image quality and lesion detection
ABDOMINAL RADIOLOGY(2022)
摘要
Purpose Fat-suppressed T2-weighted imaging (T2-FS) requires a long scan time and can be wrought with motion artifacts, urging the development of a shorter and more motion robust sequence. We compare the image quality of a single-shot T2-weighted MRI prototype with deep-learning-based image reconstruction (DL HASTE-FS) with a standard T2-FS sequence for 3 T liver MRI. Methods 41 consecutive patients with 3 T abdominal MRI examinations including standard T2-FS and DL HASTE-FS, between 5/6/2020 and 11/23/2020, comprised the study cohort. Three radiologists independently reviewed images using a 5-point Likert scale for artifact and image quality measures, while also assessing for liver lesions. Results DL HASTE-FS acquisition time was 54.93 ± 16.69, significantly ( p < .001) shorter than standard T2-FS (114.00 ± 32.98 s). DL HASTE-FS received significantly higher scores for sharpness of liver margin (4.3 vs 3.3; p < .001), hepatic vessel margin (4.2 vs 3.3; p < .001), pancreatic duct margin (4.0 vs 1.9; p < .001); in-plane (4.0 vs 3.2; p < .001) and through-plane (3.9 vs 3.4; p < .001) motion artifacts; other ghosting artifacts (4.3 vs 2.9; p < .001); and overall image quality (4.0 vs 2.9; p < .001), in addition to receiving a higher score for homogeneity of fat suppression (3.7 vs 3.4; p = .04) and liver-fat contrast ( p = .03). For liver lesions, DL HASTE-FS received significantly higher scores for sharpness of lesion margin (4.4 vs 3.7; p = .03). Conclusion Novel single-shot T2-weighted MRI with deep-learning-based image reconstruction demonstrated superior image quality compared with the standard T2-FS sequence for 3 T liver MRI, while being acquired in less than half the time. Graphical abstract
更多查看译文
关键词
Artificial intelligence,Accelerated MR imaging,MR technique,Gastrointestinal,Liver,MRI
AI 理解论文
溯源树
样例
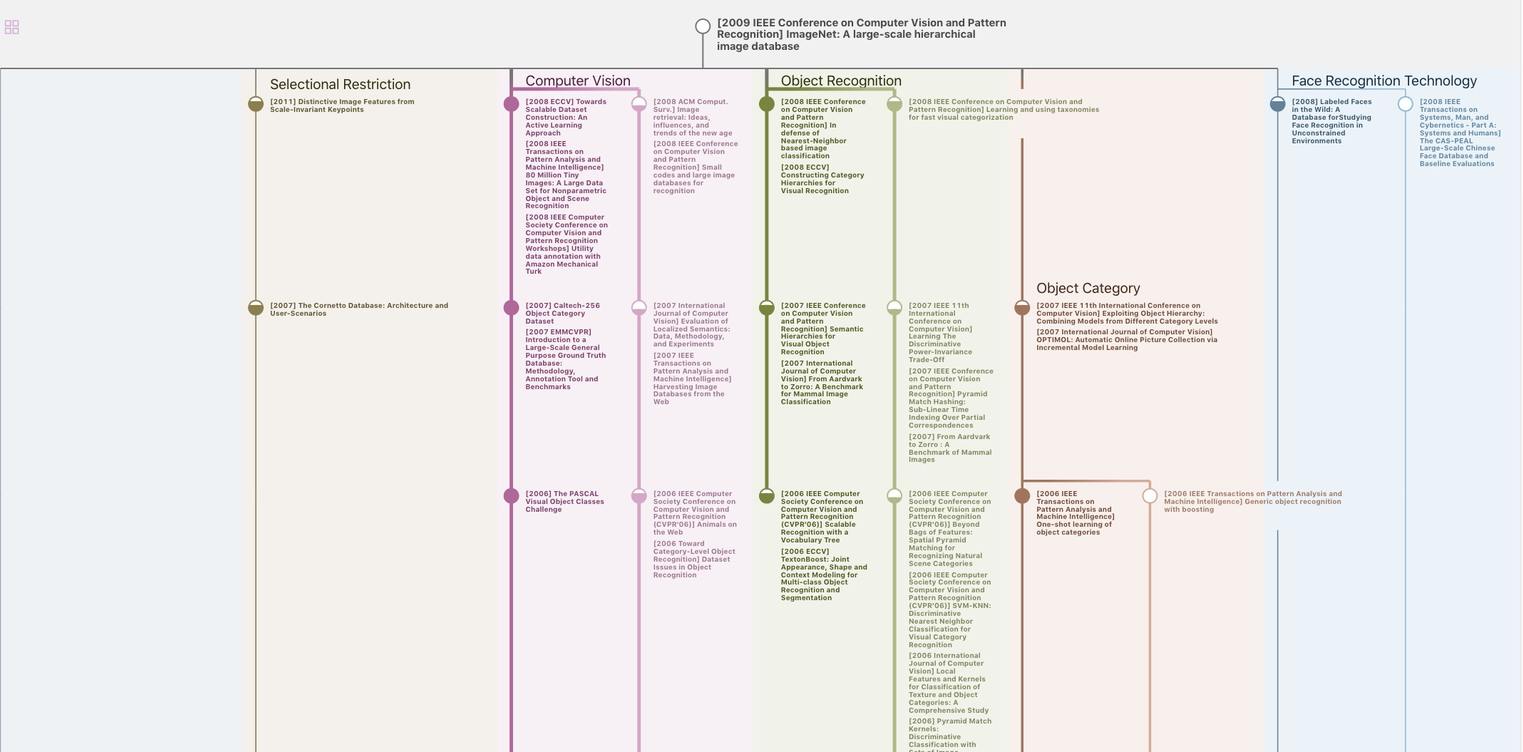
生成溯源树,研究论文发展脉络
Chat Paper
正在生成论文摘要