Delving into Light-Dark Semantic Segmentation for Indoor Scenes Understanding
Proceedings of the 1st Workshop on Photorealistic Image and Environment Synthesis for Multimedia Experiments(2022)
摘要
State-of-the-art segmentation models are mostly trained with large-scale datasets collected under favorable lighting conditions, and hence directly applying such trained models to dark scenes will result in unsatisfactory performance. In this paper, we present the first benchmark dataset and evaluation methodology to study the problem of semantic segmentation under different lighting conditions for indoor scenes. Our dataset, namely LDIS, consists of samples collected from 87 different indoor scenes under both well-illuminated and low-light conditions. Different from existing work, our benchmark provides a new task setting, namely Light-Dark Semantic Segmentation (LDSS), which adopts four different evaluation metrics that assess the performance of a model from multiple aspects. We perform extensive experiments and ablation studies to compare the effectiveness of different existing techniques with our standardized evaluation protocol. In addition, we propose a new technique, namely DepthAux, that utilizes the consistency of depth images under different lighting conditions to help a model learn a unified and illumination-invariant representation. Our experimental results show that the proposed DepthAux can provide consistent and significant improvements when applied to a variety of different models. Our dataset and other resources are publicly available on our project page: http://mercy.cse.lehigh.edu/LDIS/
更多查看译文
AI 理解论文
溯源树
样例
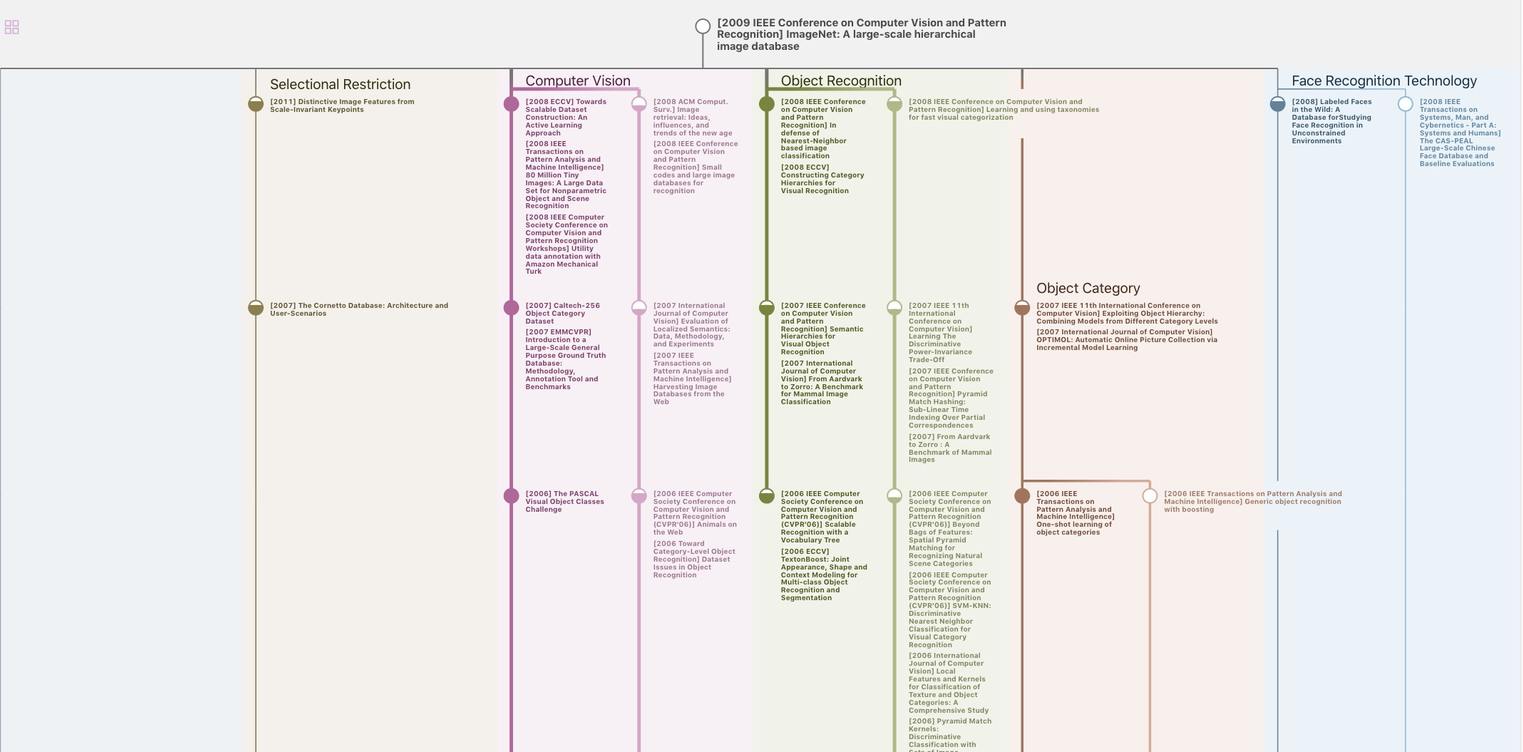
生成溯源树,研究论文发展脉络
Chat Paper
正在生成论文摘要