Predicting User Preferences of Dimensionality Reduction Embedding Quality
IEEE Transactions on Visualization and Computer Graphics(2023)
摘要
A plethora of dimensionality reduction techniques have emerged over the past decades, leaving researchers and analysts with a wide variety of choices for reducing their data, all the more so given some techniques come with additional hyper-parametrization (e.g., t-SNE, UMAP, etc.). Recent studies are showing that people often use dimensionality reduction as a black-box regardless of the specific properties the method itself preserves. Hence, evaluating and comparing 2D embeddings is usually qualitatively decided, by setting embeddings side-by-side and letting human judgment decide which embedding is the best. In this work, we propose a quantitative way of evaluating embeddings, that nonetheless places human perception at the center. We run a comparative study, where we ask people to select “good” and “misleading” views between scatterplots of low-dimensional embeddings of image datasets, simulating the way people usually select embeddings. We use the study data as labels for a set of quality metrics for a supervised machine learning model whose purpose is to discover and quantify what exactly people are looking for when deciding between embeddings. With the model as a proxy for human judgments, we use it to rank embeddings on new datasets, explain why they are relevant, and quantify the degree of subjectivity when people select preferred embeddings.
更多查看译文
关键词
Dimensionality reduction,Manifold learning,Human-centered computing
AI 理解论文
溯源树
样例
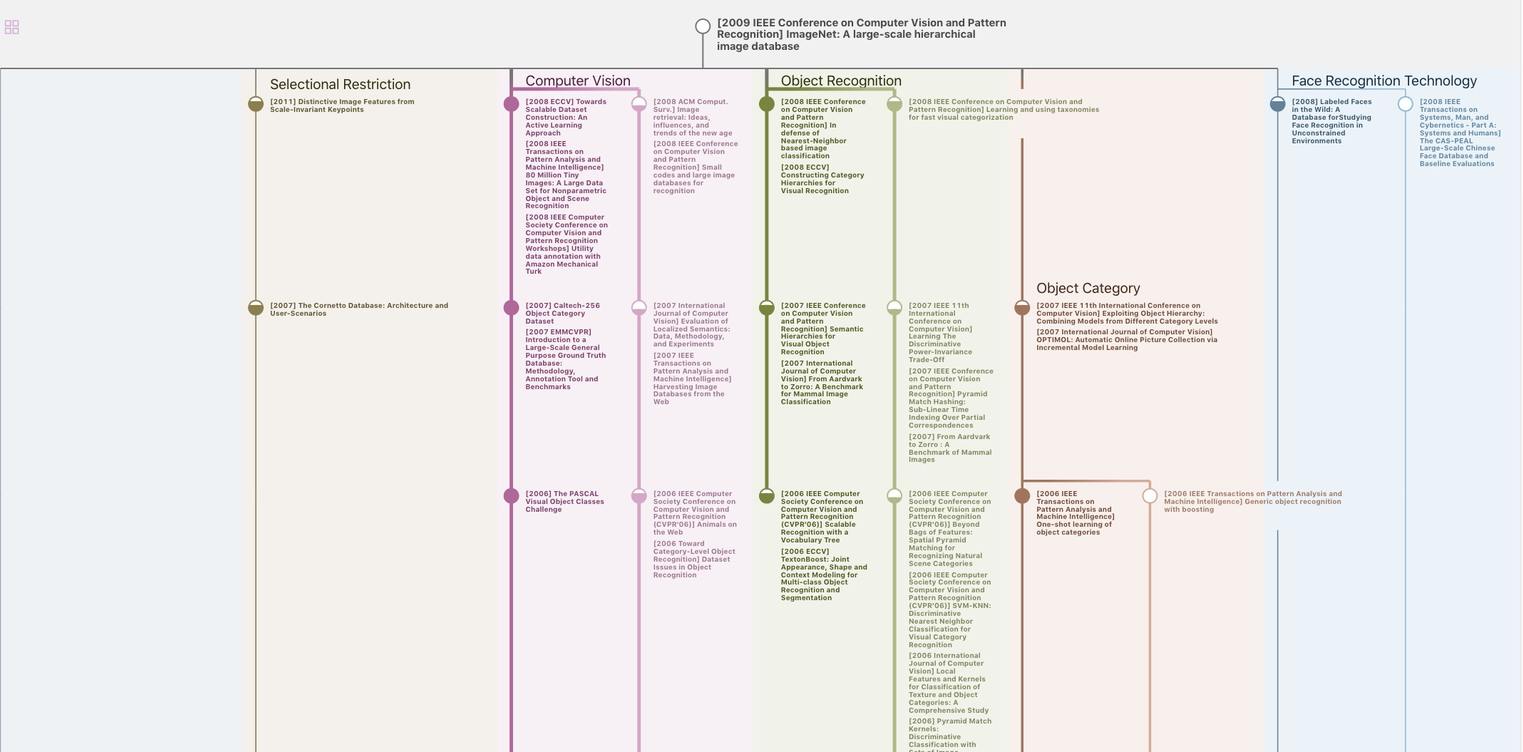
生成溯源树,研究论文发展脉络
Chat Paper
正在生成论文摘要