A comparison of deep-learning-based inpainting techniques for experimental X-ray scattering
JOURNAL OF APPLIED CRYSTALLOGRAPHY(2022)
摘要
The implementation is proposed of image inpainting techniques for the reconstruction of gaps in experimental X-ray scattering data. The proposed methods use deep learning neural network architectures, such as convolutional autoencoders, tunable U-Nets, partial convolution neural networks and mixedscale dense networks, to reconstruct the missing information in experimental scattering images. In particular, the recovered pixel intensities are evaluated against their corresponding ground-truth values using the mean absolute error and the correlation coefficient metrics. The results demonstrate that the proposed methods achieve better performance than traditional inpainting algorithms such as biharmonic functions. Overall, tunable U-Net and mixedscale dense network architectures achieved the best reconstruction performance among all the tested algorithms, with correlation coefficient scores greater than 0.9980.
更多查看译文
关键词
X-ray scattering,image inpainting,deep learning,mixed-scale dense networks,tunable U-Nets
AI 理解论文
溯源树
样例
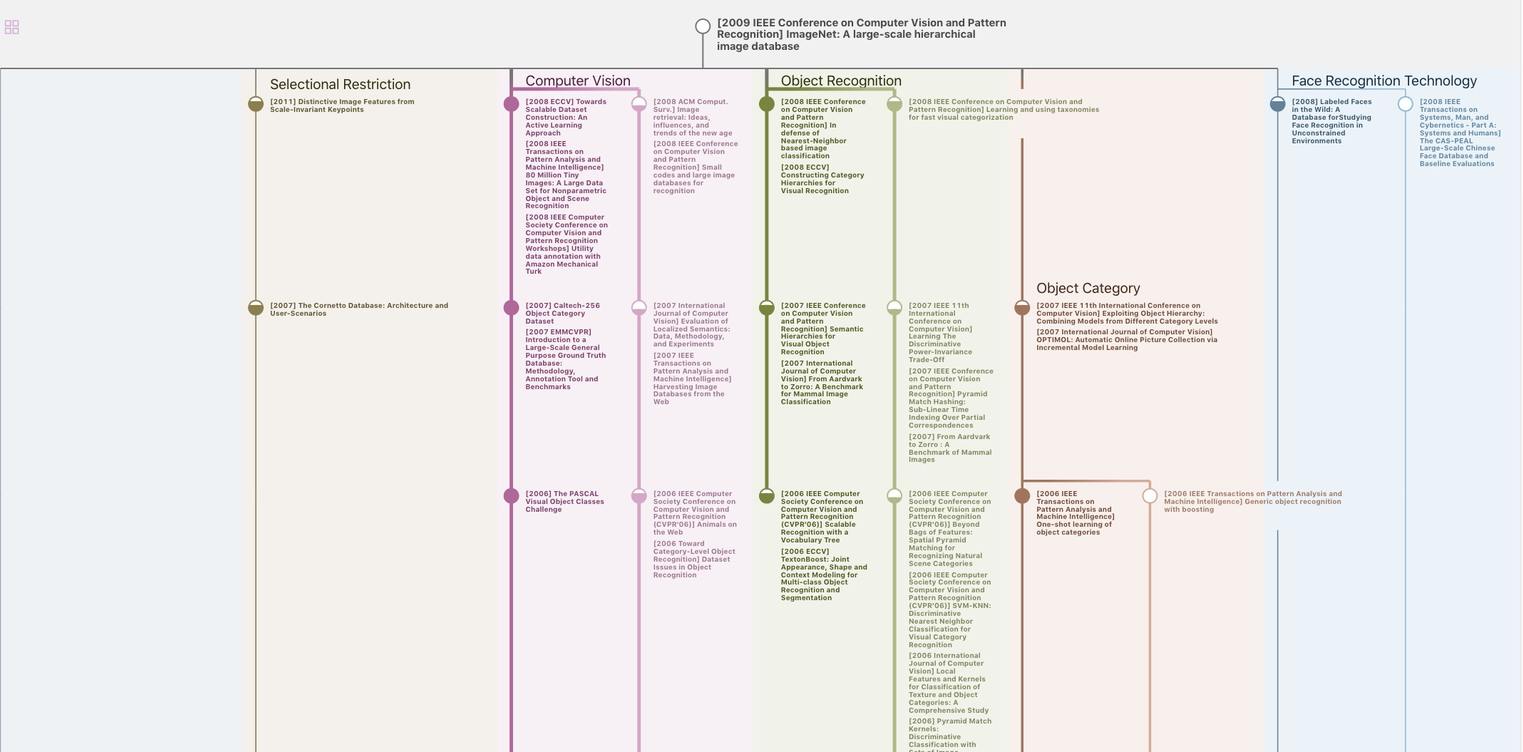
生成溯源树,研究论文发展脉络
Chat Paper
正在生成论文摘要