Cluster-based demand forecasting using Bayesian model averaging: An ensemble learning approach
Decision Analytics Journal(2022)
摘要
Demand forecasting is an important aspect in supply chain management that could contribute to enhancing the profit and increasing the efficiency by aligning the supply channels with anticipated demand. In the retail industry, customers and their needs are diverse making demand forecasting a challenging task. In this regard, this study aims at developing a three-step data-driven cluster-based demand forecasting approach for the retail industry. First, customers are segmented based on their recency, frequency, and monetary (RFM) characteristics. Customers with similar buying behaviors are recognized as a segment, creating an ordered relationship between transactions made by them. In the second step, time-series analysis techniques are used to forecast demand for each customer segment. Finally, Bayesian model averaging (BMA) is adopted to ensemble the forecasting results obtained from alternative time series techniques. The applicability of the proposed approach is presented through a comparative case study analysis with presented improvement in the accuracy of daily demand prediction.
更多查看译文
关键词
Demand forecasting,Customer segmentation,Multivariate time-series forecasting,Ensemble learning
AI 理解论文
溯源树
样例
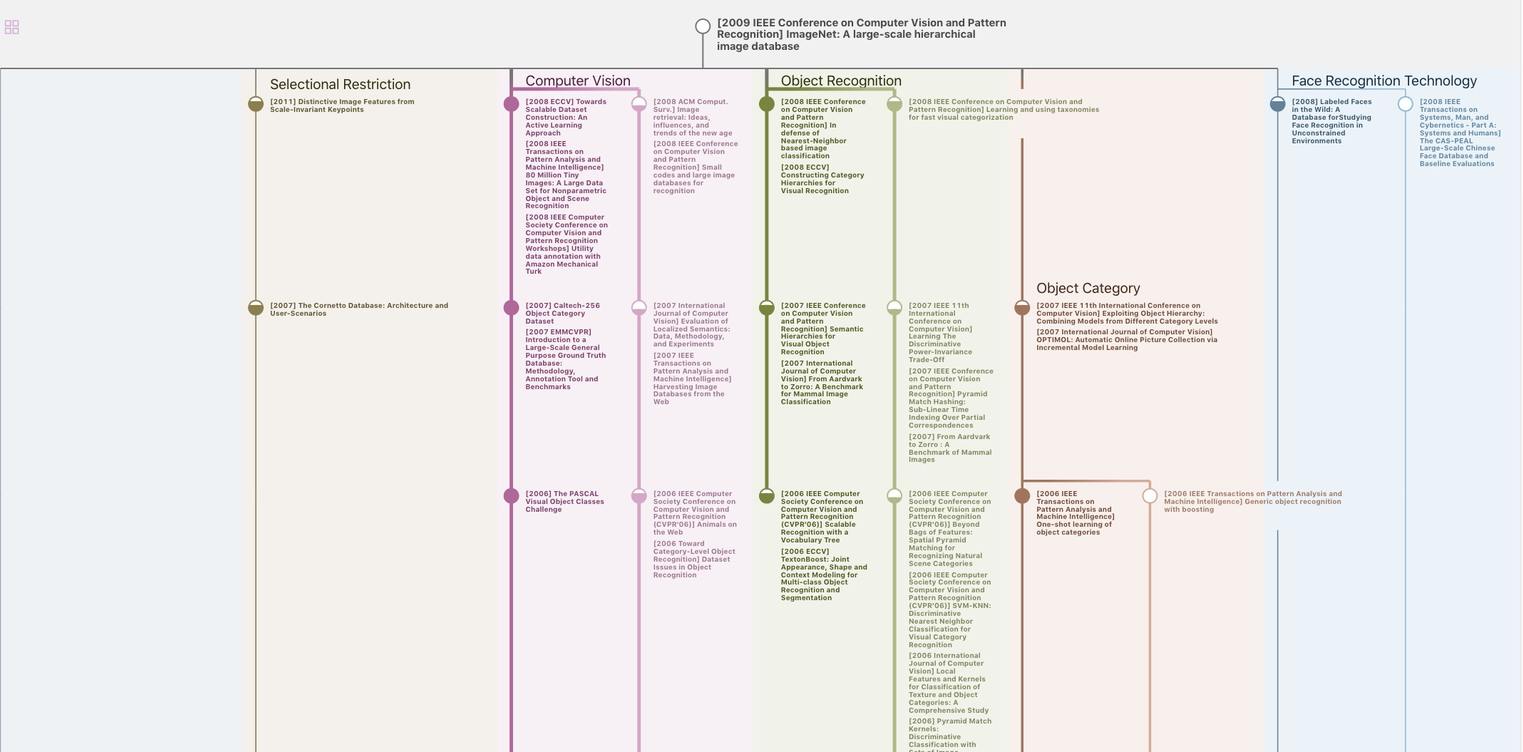
生成溯源树,研究论文发展脉络
Chat Paper
正在生成论文摘要