Exact and efficient multivariate two-sample tests through generalized linear rank statistics
arxiv(2022)
摘要
So-called linear rank statistics provide a means for distribution-free (even in finite samples), yet highly flexible, two-sample testing in the setting of univariate random variables. Their flexibility derives from a choice of weights that can be adapted to any given (simple) alternative hypothesis to achieve efficiency in case of correct specification of said alternative, while their non-parametric nature guarantees well-calibrated $p$-values even under misspecification. By drawing connections to (generalized) maximum likelihood estimation, and exploiting recent work on ranks in multiple dimensions, we extend linear rank statistics both to multivariate random variables and composite alternatives. Doing so yields non-parametric, multivariate two-sample tests that mirror efficiency properties of likelihood ratio tests, while remaining robust against model misspecification. We prove non-parametric versions of the classical Wald and score tests facilitating hypothesis testing in the asymptotic regime, and relate these generalized linear rank statistics to linear spacing statistics enabling exact $p$-value computations in the small to moderate sample setting. Moreover, viewing rank statistics through the lens of likelihood ratios affords applications beyond fully efficient two-sample testing, of which we demonstrate three: testing in the presence of nuisance alternatives, simultaneous detection of location and scale shifts, and $K$-sample testing.
更多查看译文
关键词
efficient multivariate,tests,statistics,two-sample
AI 理解论文
溯源树
样例
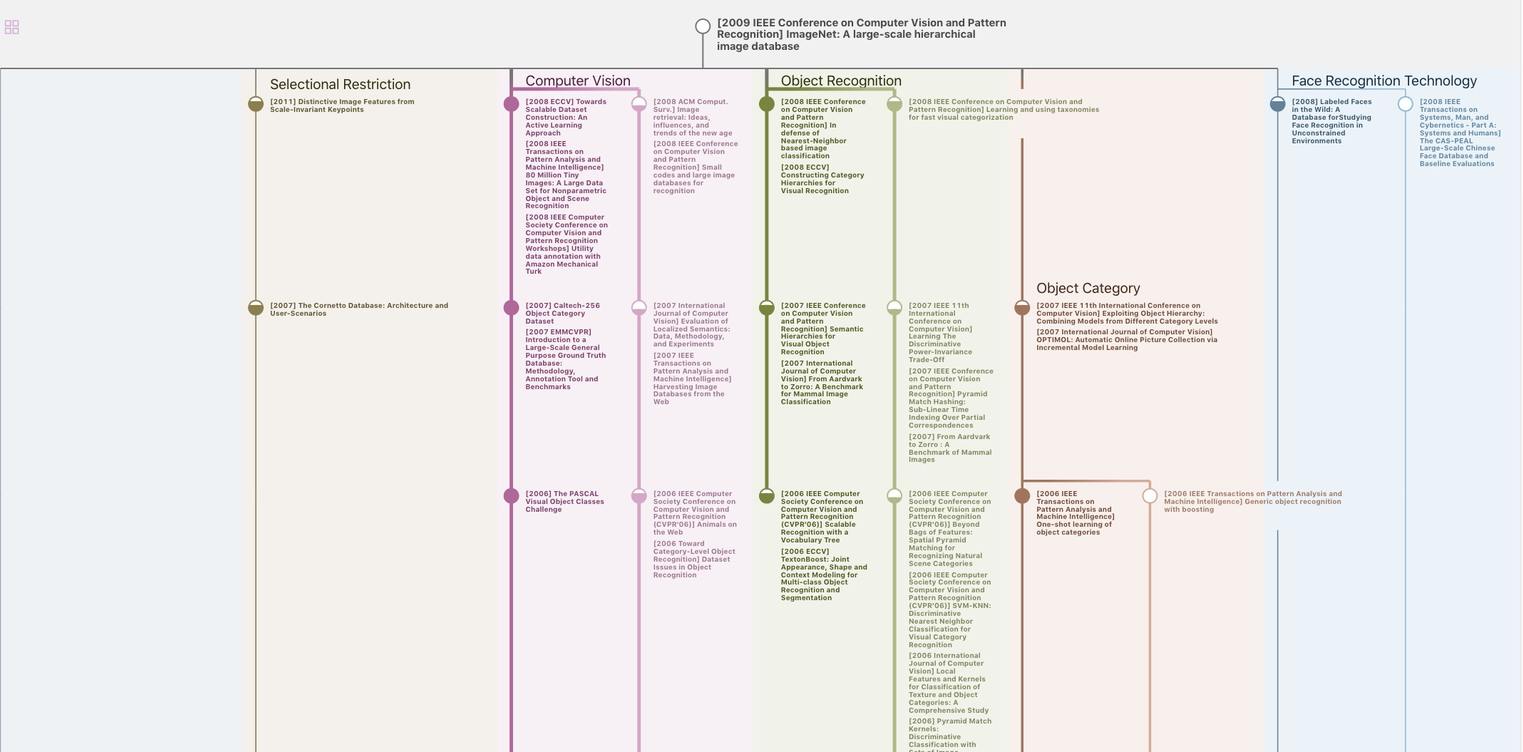
生成溯源树,研究论文发展脉络
Chat Paper
正在生成论文摘要