Global Weighted Tensor Nuclear Norm for Tensor Robust Principal Component Analysis
arxiv(2022)
摘要
Tensor Robust Principal Component Analysis (TRPCA), which aims to recover a low-rank tensor corrupted by sparse noise, has attracted much attention in many real applications. This paper develops a new Global Weighted TRPCA method (GWTRPCA), which is the first approach simultaneously considers the significance of intra-frontal slice and inter-frontal slice singular values in the Fourier domain. Exploiting this global information, GWTRPCA penalizes the larger singular values less and assigns smaller weights to them. Hence, our method can recover the low-tubal-rank components more exactly. Moreover, we propose an effective adaptive weight learning strategy by a Modified Cauchy Estimator (MCE) since the weight setting plays a crucial role in the success of GWTRPCA. To implement the GWTRPCA method, we devise an optimization algorithm using an Alternating Direction Method of Multipliers (ADMM) method. Experiments on real-world datasets validate the effectiveness of our proposed method.
更多查看译文
关键词
tensor robust principal
AI 理解论文
溯源树
样例
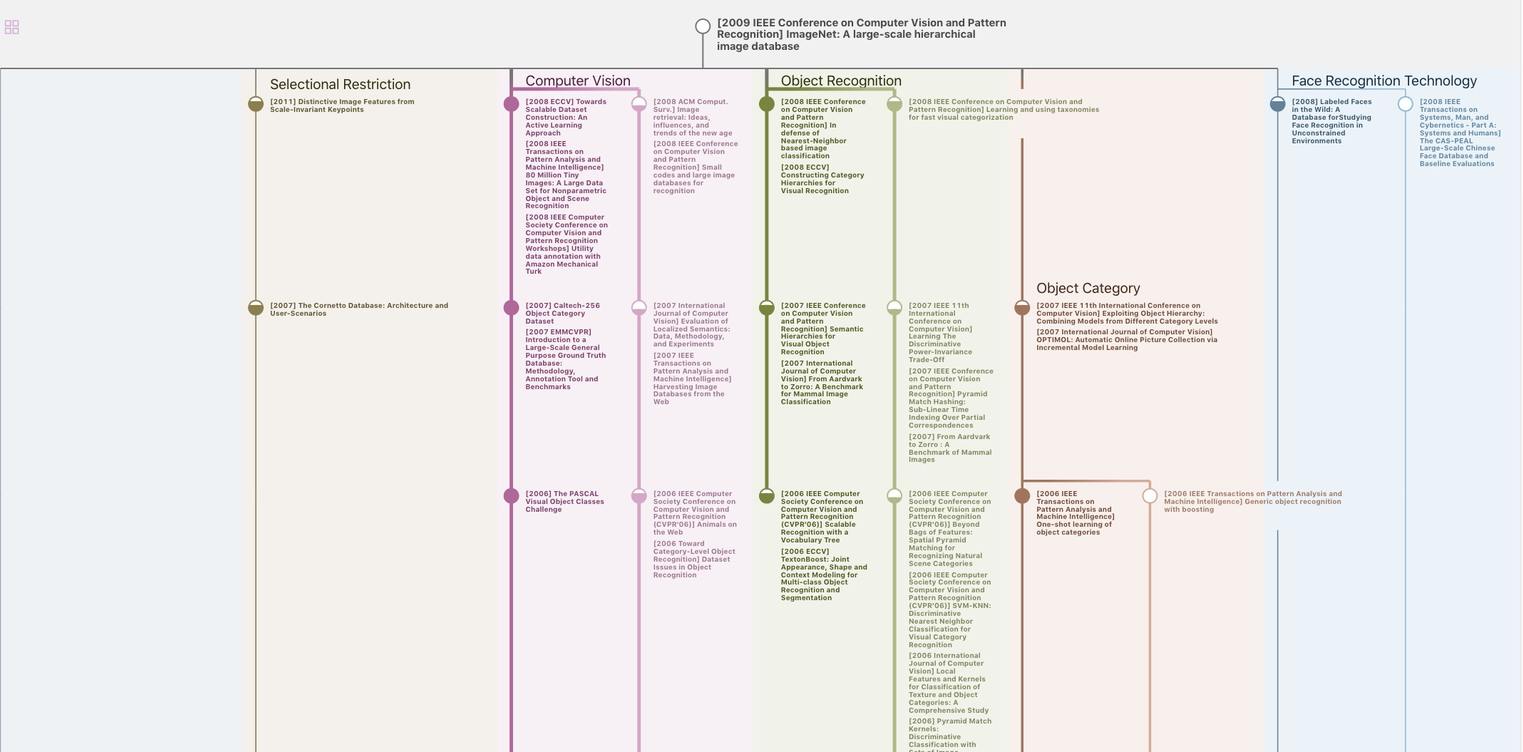
生成溯源树,研究论文发展脉络
Chat Paper
正在生成论文摘要