FINet: An Insulator Dataset and Detection Benchmark Based on Synthetic Fog and Improved YOLOv5
IEEE TRANSACTIONS ON INSTRUMENTATION AND MEASUREMENT(2022)
摘要
The inspection of insulators and their defects is of great significance for ensuring the safety and stability of power system. Small sample is one of the main issues of insulator defect detection based on neural networks. In this research, we release a dataset for insulators and self-explosive defects detection and provide a benchmark based on improved YOLOv5, named Foggy Insulator Network (FINet). In this work, a synthetic fog algorithm is implemented and optimized. A synthetic foggy insulator dataset (SFID) with 13 000 images is constructed and released. The YOLOv5 network is improved into SE-YOLOv5 by introducing the channel attention mechanism, and a robust detection model with 96.2% F1 score for insulators and their defects is trained from scratch and served as a benchmark. The synthetic fog algorithm proposed in this article can be widely used for data augmentation of various datasets. The trained model can be applied in the field of transmission line inspection. The source codes, datasets, and tutorials are available on GitHub.
更多查看译文
关键词
Insulators,Image color analysis,Brightness,Gray-scale,Training,Optimization,Neural networks,Datasets,data augmentation,deep learning,defect detection,insulator,power grid,synthetic fog
AI 理解论文
溯源树
样例
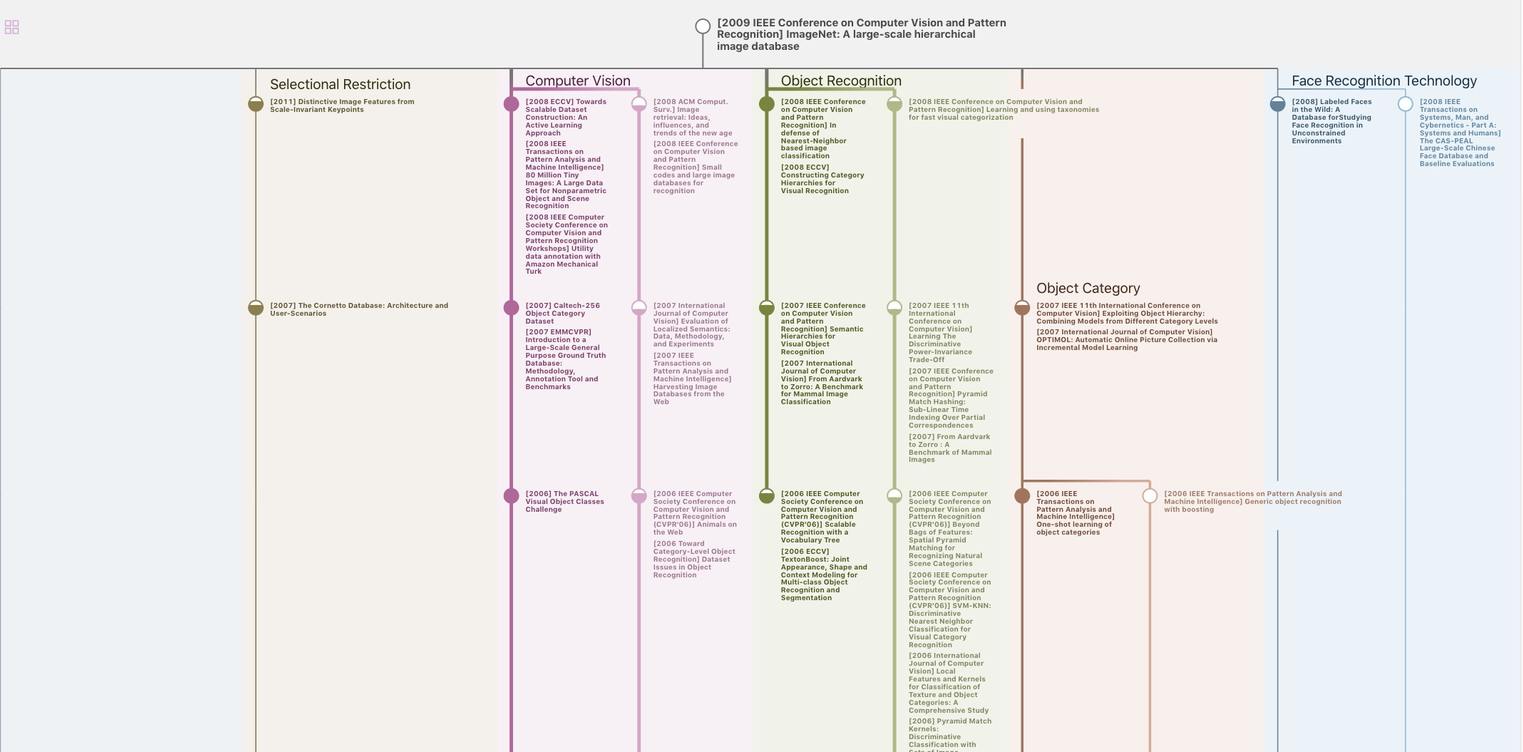
生成溯源树,研究论文发展脉络
Chat Paper
正在生成论文摘要