Estimating individual treatment effect on disability progression in multiple sclerosis using deep learning
NATURE COMMUNICATIONS(2022)
摘要
Disability progression in multiple sclerosis remains resistant to treatment. The absence of a suitable biomarker to allow for phase 2 clinical trials presents a high barrier for drug development. We propose to enable short proof-of-concept trials by increasing statistical power using a deep-learning predictive enrichment strategy. Specifically, a multi-headed multilayer perceptron is used to estimate the conditional average treatment effect (CATE) using baseline clinical and imaging features, and patients predicted to be most responsive are preferentially randomized into a trial. Leveraging data from six randomized clinical trials ( n = 3,830), we first pre-trained the model on the subset of relapsing-remitting MS patients ( n = 2,520), then fine-tuned it on a subset of primary progressive MS (PPMS) patients ( n = 695). In a separate held-out test set of PPMS patients randomized to anti-CD20 antibodies or placebo ( n = 297), the average treatment effect was larger for the 50% (HR, 0.492; 95% CI, 0.266-0.912; p = 0.0218) and 30% (HR, 0.361; 95% CI, 0.165-0.79; p = 0.008) predicted to be most responsive, compared to 0.743 (95% CI, 0.482-1.15; p = 0.179) for the entire group. The same model could also identify responders to laquinimod in another held-out test set of PPMS patients ( n = 318). Finally, we show that using this model for predictive enrichment results in important increases in power.
更多查看译文
关键词
multiple sclerosis,disability progression,deep learning,individual treatment effect
AI 理解论文
溯源树
样例
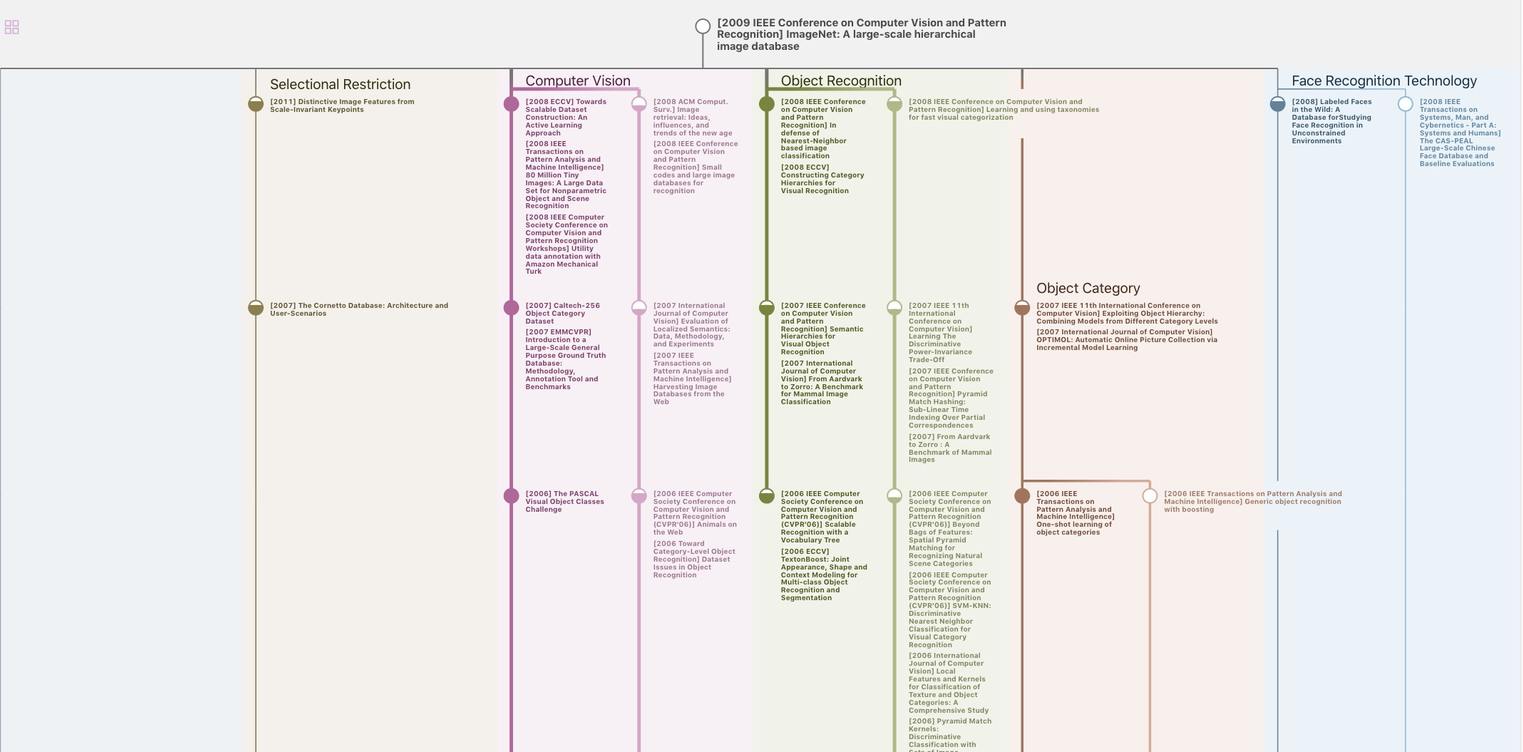
生成溯源树,研究论文发展脉络
Chat Paper
正在生成论文摘要