Predicting survival in metastatic non-small cell lung cancer patients with poor ECOG-PS: A single-arm prospective study
Cancer medicine(2023)
摘要
Background Patients with advanced non-small cell lung cancer (NSCLC) are a heterogeneous population with short lifespan. We aimed to develop methods to better differentiate patients whose survival was >90 days. Methods We evaluated 83 characteristics of 106 treatment-naive, stage IV NSCLC patients with Eastern Cooperative Oncology Group Performance Status (ECOG-PS) >1. Automated machine learning was used to select a model and optimize hyperparameters. 100-fold bootstrapping was performed for dimensionality reduction for a second ("lite") model. Performance was measured by C-statistic and accuracy metrics in an out-of-sample validation cohort. The "lite" model was validated on a second independent, prospective cohort (N = 42). Network analysis (NA) was performed to evaluate the differences in centrality and connectivity of features. Results The selected method was ExtraTrees Classifier, with C-statistic of 0.82 (p < 0.01) and accuracy of 0.81 (p = 0.01). The "lite" model had 16 variables and obtained C-statistic of 0.84 (p < 0.01) and accuracy of 0.75 (p = 0.039) in the first cohort, and C-statistic of 0.706 (p < 0.01) and accuracy of 0.714 (p < 0.01) in the second cohort. The networks of patients with lower survival were more interconnected. Features related to cachexia, inflammation, and quality of life had statistically different prestige scores in NA. Conclusions Machine learning can assist in the prognostic evaluation of advanced NSCLC. The model generated with a reduced number of features showed high accessibility and reasonable metrics. Features related to quality of life, cachexia, and performance status had increased correlation and importance scores, suggesting that they play a role at later disease stages, in line with the biological rationale already described.
更多查看译文
关键词
machine learning,network analysis,non-small cell lung cancer,poor performance status,prognostic markers
AI 理解论文
溯源树
样例
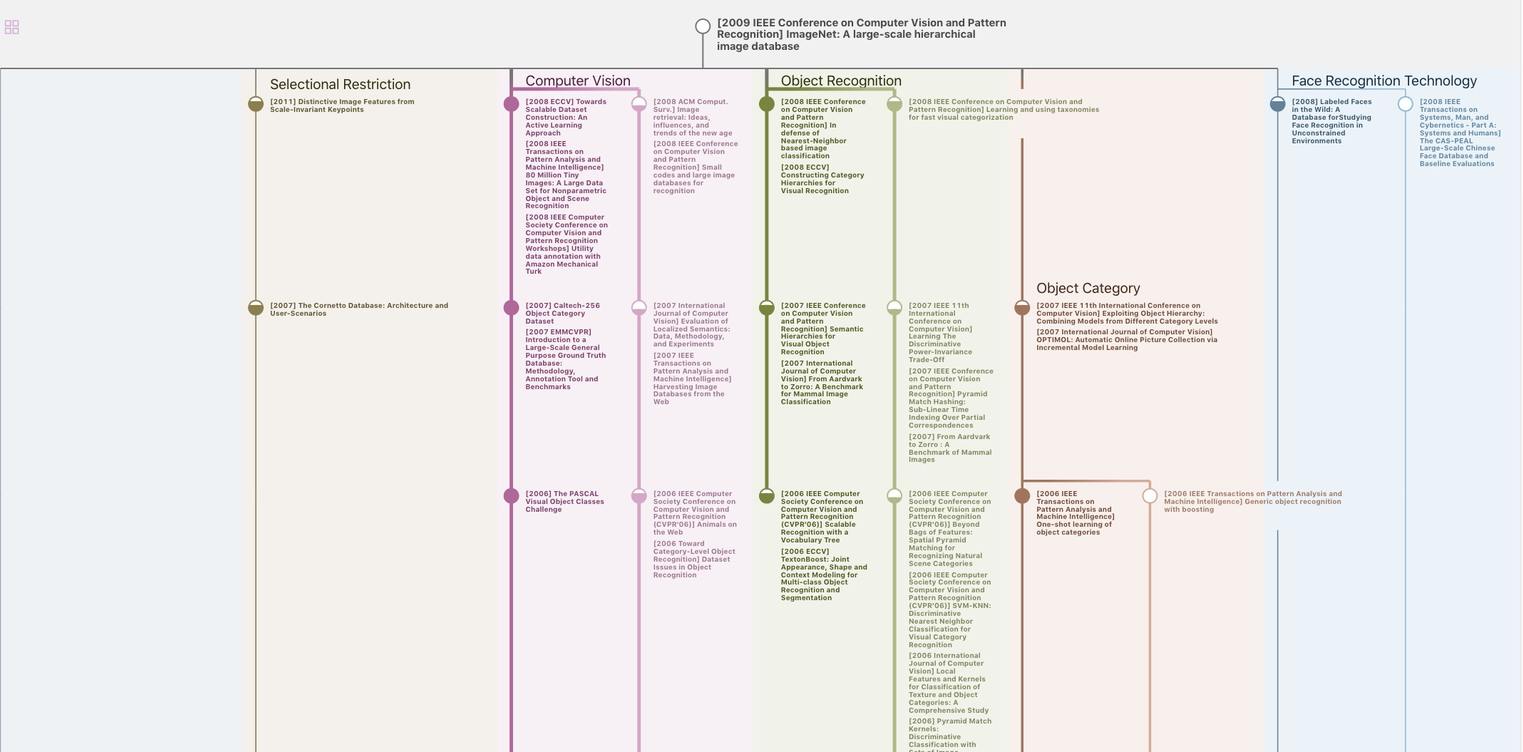
生成溯源树,研究论文发展脉络
Chat Paper
正在生成论文摘要