Radiogenomic markers enable risk stratification and inference of mutational pathway states in head and neck cancer
European journal of nuclear medicine and molecular imaging(2022)
摘要
Purpose Head and neck squamous cell carcinomas (HNSCCs) are a molecularly, histologically, and clinically heterogeneous set of tumors originating from the mucosal epithelium of the oral cavity, pharynx, and larynx. This heterogeneous nature of HNSCC is one of the main contributing factors to the lack of prognostic markers for personalized treatment. The aim of this study was to develop and identify multi-omics markers capable of improved risk stratification in this highly heterogeneous patient population. Methods In this retrospective study, we approached this issue by establishing radiogenomics markers to identify high-risk individuals in a cohort of 127 HNSCC patients. Hybrid in vivo imaging and whole-exome sequencing were employed to identify quantitative imaging markers as well as genetic markers on pathway-level prognostic in HNSCC. We investigated the deductibility of the prognostic genetic markers using anatomical and metabolic imaging using positron emission tomography combined with computed tomography. Moreover, we used statistical and machine learning modeling to investigate whether a multi-omics approach can be used to derive prognostic markers for HNSCC. Results Radiogenomic analysis revealed a significant influence of genetic pathway alterations on imaging markers. A highly prognostic radiogenomic marker based on cellular senescence was identified. Furthermore, the radiogenomic biomarkers designed in this study vastly outperformed the prognostic value of markers derived from genetics and imaging alone. Conclusion Using the identified markers, a clinically meaningful stratification of patients is possible, guiding the identification of high-risk patients and potentially aiding in the development of effective targeted therapies. Graphical abstract
更多查看译文
关键词
Head and neck cancer,Biomarkers,Radiomics,Machine learning,Artificial intelligence,Cancer genomics
AI 理解论文
溯源树
样例
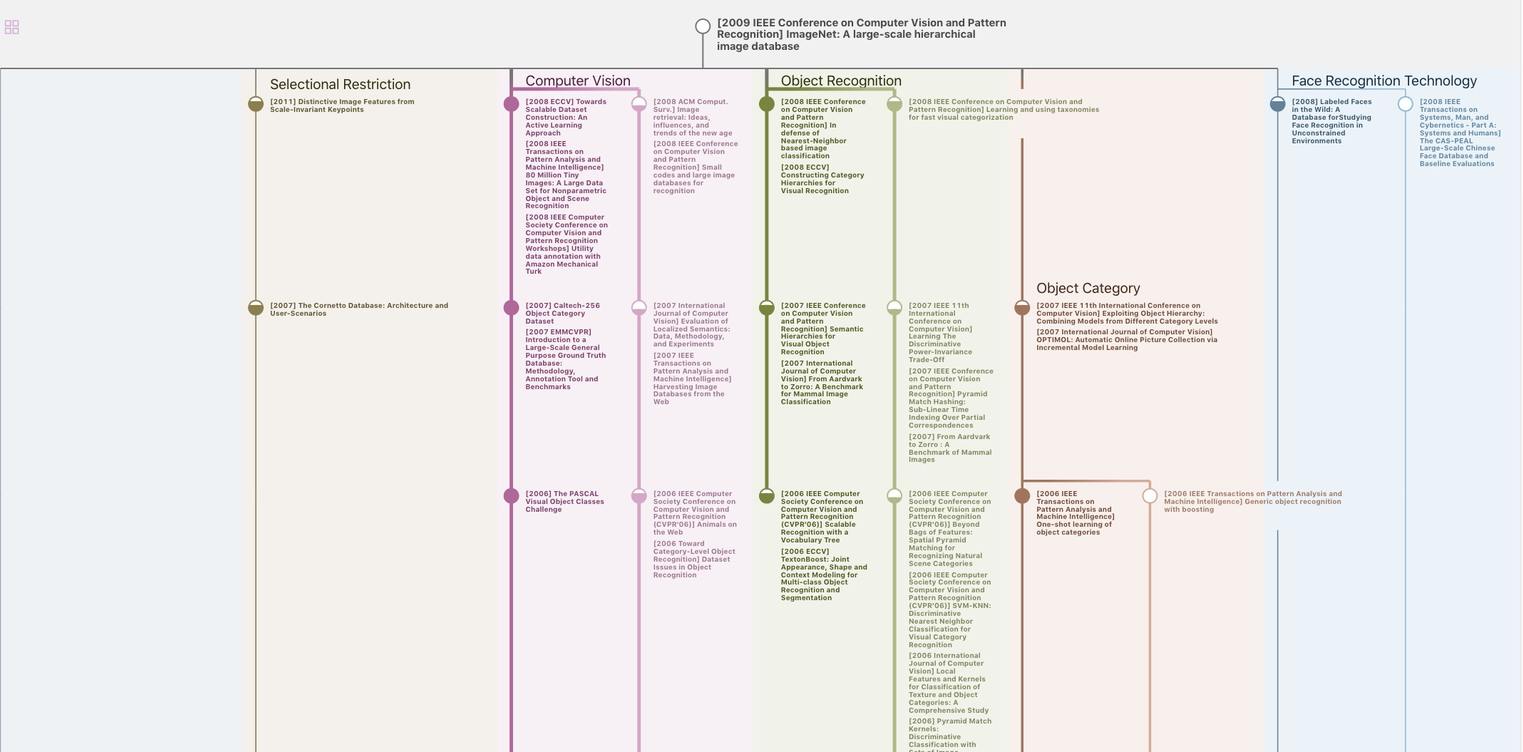
生成溯源树,研究论文发展脉络
Chat Paper
正在生成论文摘要