Spiking GATs: Learning Graph Attentions via Spiking Neural Network
arxiv(2022)
摘要
Graph Attention Networks (GATs) have been intensively studied and widely used in graph data learning tasks. Existing GATs generally adopt the self-attention mechanism to conduct graph edge attention learning, requiring expensive computation. It is known that Spiking Neural Networks (SNNs) can perform inexpensive computation by transmitting the input signal data into discrete spike trains and can also return sparse outputs. Inspired by the merits of SNNs, in this work, we propose a novel Graph Spiking Attention Network (GSAT) for graph data representation and learning. In contrast to self-attention mechanism in existing GATs, the proposed GSAT adopts a SNN module architecture which is obvious energy-efficient. Moreover, GSAT can return sparse attention coefficients in natural and thus can perform feature aggregation on the selective neighbors which makes GSAT perform robustly w.r.t graph edge noises. Experimental results on several datasets demonstrate the effectiveness, energy efficiency and robustness of the proposed GSAT model.
更多查看译文
关键词
graph attentions,gats,neural network,learning
AI 理解论文
溯源树
样例
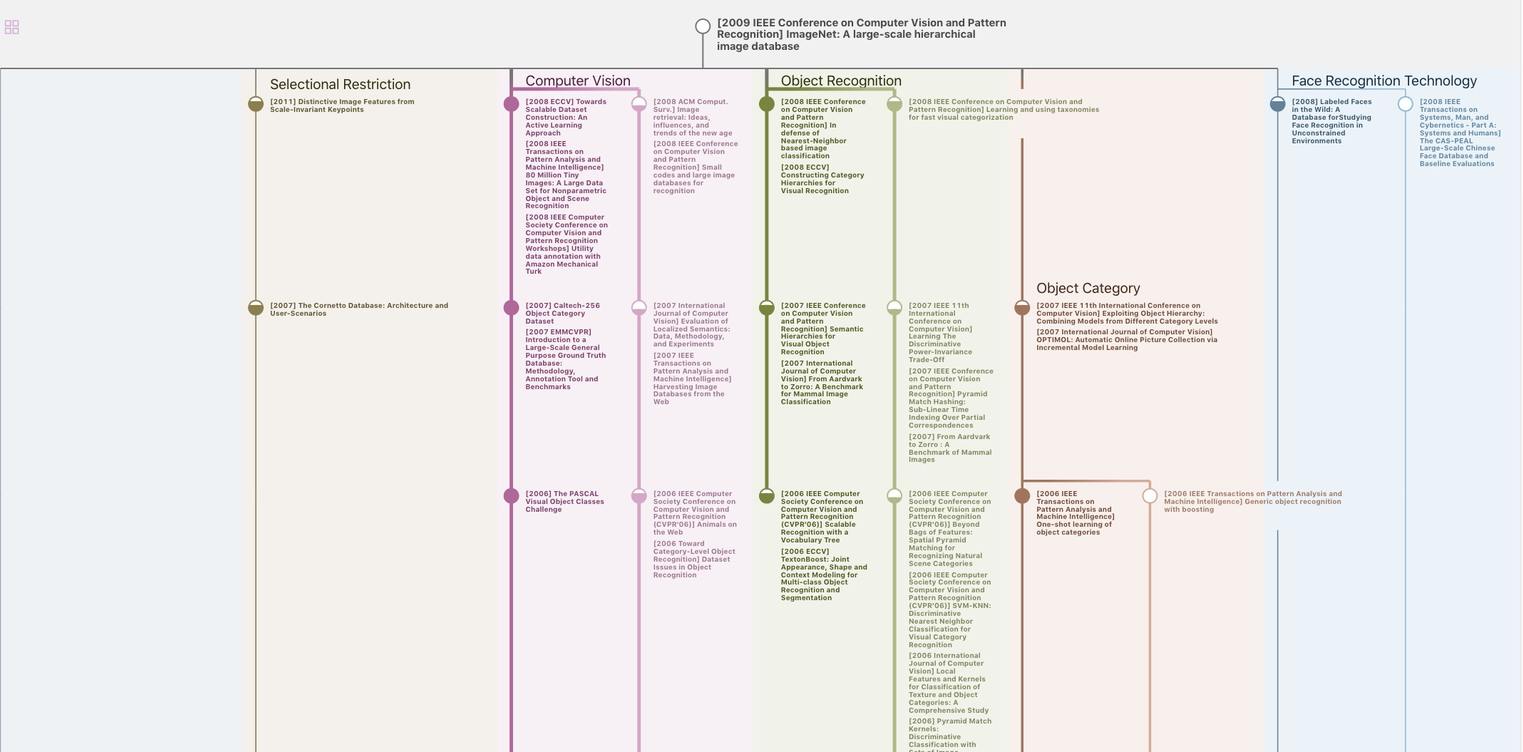
生成溯源树,研究论文发展脉络
Chat Paper
正在生成论文摘要