StyleSwap: Style-Based Generator Empowers Robust Face Swapping
Computer Vision – ECCV 2022(2022)
摘要
Numerous attempts have been made to the task of person-agnostic face swapping given its wide applications. While existing methods mostly rely on tedious network and loss designs, they still struggle in the information balancing between the source and target faces, and tend to produce visible artifacts. In this work, we introduce a concise and effective framework named StyleSwap. Our core idea is to leverage a style-based generator to empower high-fidelity and robust face swapping, thus the generator’s advantage can be adopted for optimizing identity similarity. We identify that with only minimal modifications, a StyleGAN2 architecture can successfully handle the desired information from both source and target. Additionally, inspired by the ToRGB layers, a Swapping-Driven Mask Branch is further devised to improve information blending. Furthermore, the advantage of StyleGAN inversion can be adopted. Particularly, a Swapping-Guided ID Inversion strategy is proposed to optimize identity similarity. Extensive experiments validate that our framework generates high-quality face swapping results that outperform state-of-the-art methods both qualitatively and quantitatively.
更多查看译文
关键词
Face swapping, Style-based generator, GAN
AI 理解论文
溯源树
样例
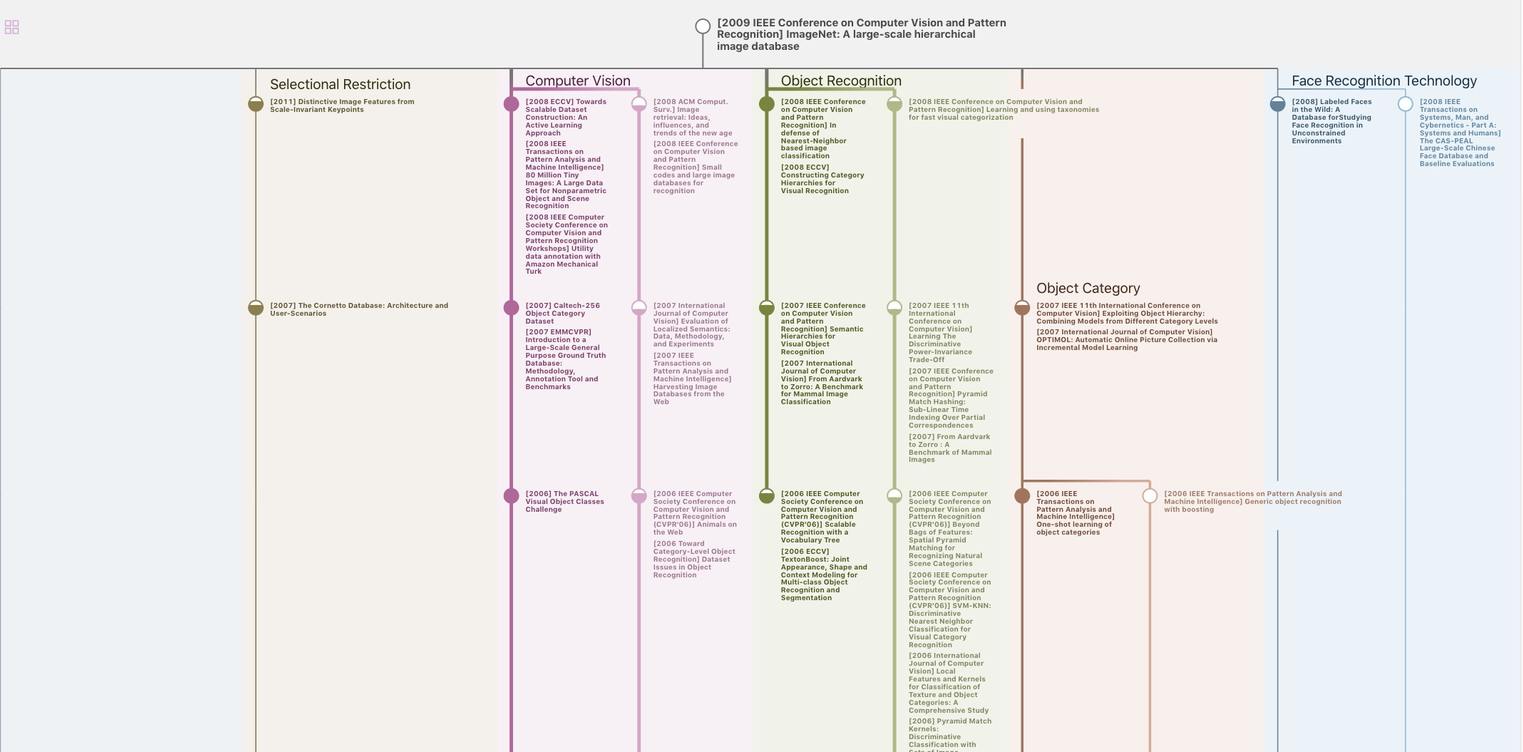
生成溯源树,研究论文发展脉络
Chat Paper
正在生成论文摘要