Regularized Soft Actor-Critic for Behavior Transfer Learning
2022 IEEE Conference on Games (CoG)(2022)
摘要
Existing imitation learning methods mainly focus on making an agent effectively mimic a demonstrated behavior, but do not address the potential contradiction between the behavior style and the objective of a task. There is a general lack of efficient methods that allow an agent to partially imitate a demonstrated behavior to varying degrees, while completing the main objective of a task. In this paper we propose a method called Regularized Soft Actor-Critic which formulates the main task and the imitation task under the Constrained Markov Decision Process framework (CMDP). The main task is defined as the maximum entropy objective used in Soft Actor-Critic (SAC) and the imitation task is defined as a constraint. We evaluate our method on continuous control tasks relevant to video games applications.
更多查看译文
关键词
CMDP,behavior style,video game
AI 理解论文
溯源树
样例
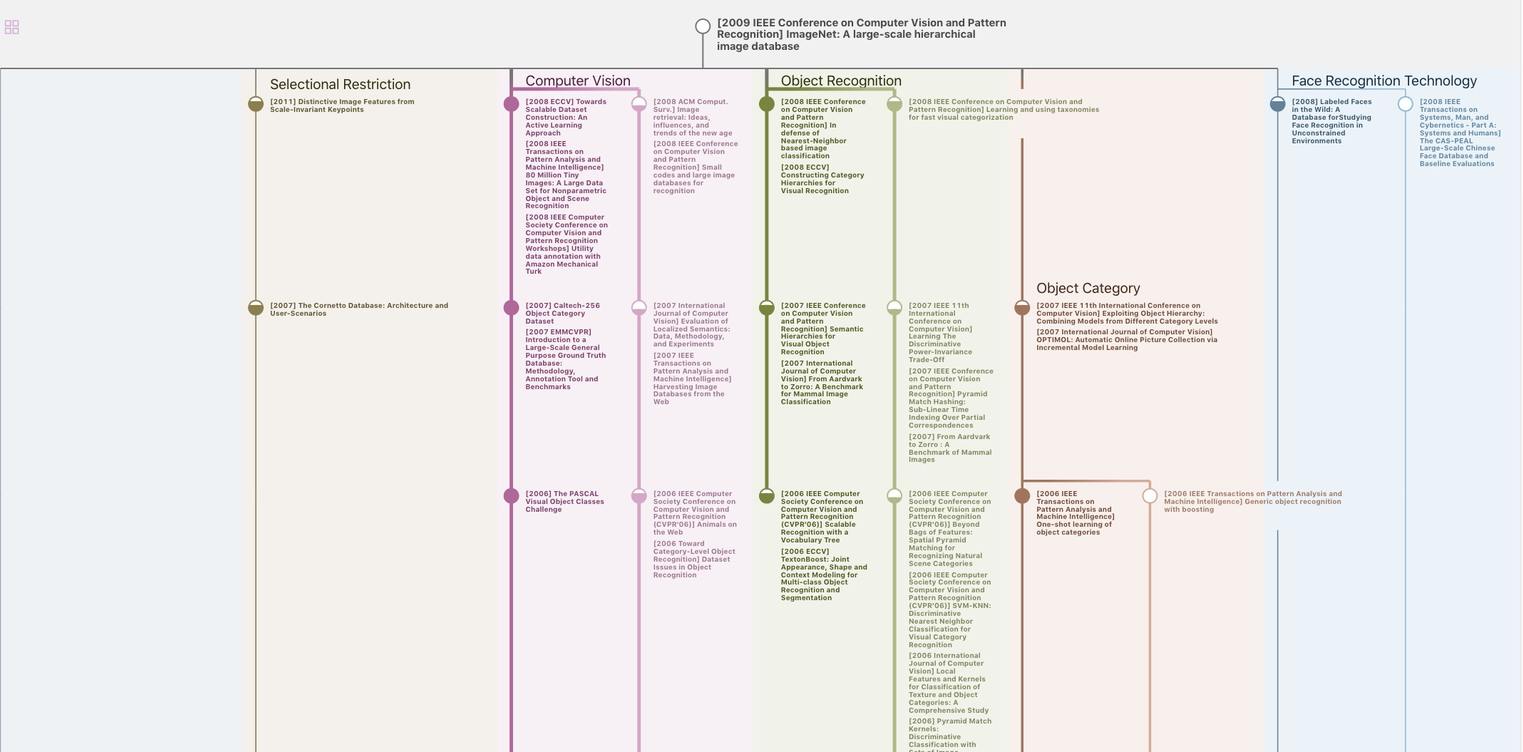
生成溯源树,研究论文发展脉络
Chat Paper
正在生成论文摘要