Improving Primal Heuristics for Mixed Integer Programming Problems based on Problem Reduction: A Learning-based Approach
2022 17th International Conference on Control, Automation, Robotics and Vision (ICARCV)(2022)
摘要
In this paper, we propose a Bi-layer Prediction-based Reduction Branch (BP-RB) framework to speed up the process of finding a high-quality feasible solution for Mixed Integer Programming (MIP) problems. A graph convolutional network (GCN) is employed to predict binary variables' values. After that, a subset of binary variables is fixed to the predicted value by a greedy method conditioned on the predicted probabilities. By exploring the logical consequences, a learning-based problem reduction method is proposed, significantly reducing the variable and constraint sizes. With the reductive sub- MIP problem, the second layer GCN framework is employed to update the prediction for the remaining binary variables' values and to determine the selection of variables which are then used for branching to generate the Branch and Bound (B&B) tree. Numerical examples show that our BP-RB framework speeds up the primal heuristic and finds the feasible solution with high quality.
更多查看译文
关键词
mixed integer programming problems,primal heuristics,problems reduction,learning-based
AI 理解论文
溯源树
样例
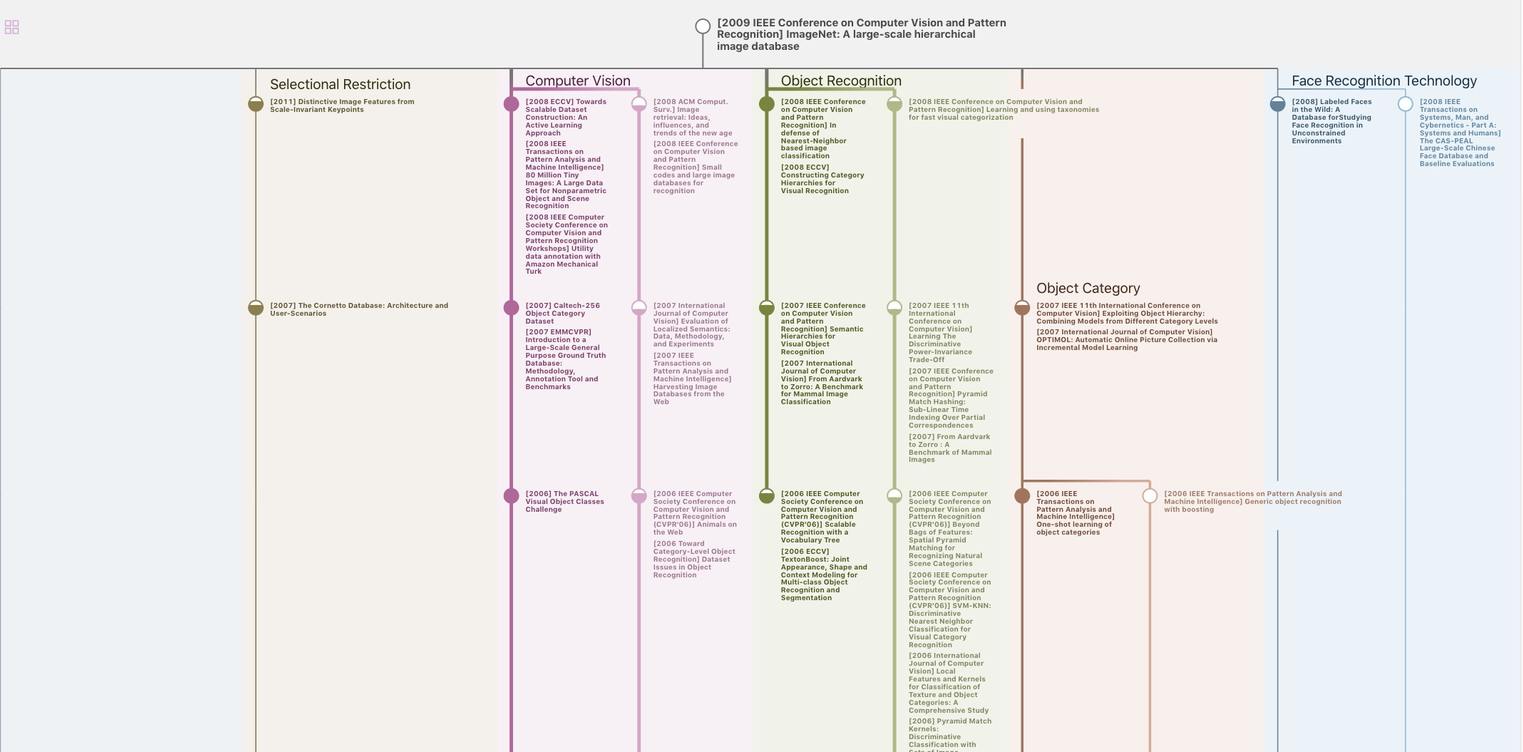
生成溯源树,研究论文发展脉络
Chat Paper
正在生成论文摘要