Multiscale Latent-Guided Entropy Model for LiDAR Point Cloud Compression
IEEE TRANSACTIONS ON CIRCUITS AND SYSTEMS FOR VIDEO TECHNOLOGY(2023)
摘要
The non-uniform distribution and extremely sparse nature of the LiDAR point cloud (LPC) bring significant challenges to its high-efficient compression. This paper proposes a novel end-to-end, fully-factorized deep framework that represents the original LiDAR point cloud into an octree structure and hierarchically constructs the octree entropy model in layers. The proposed framework utilizes a hierarchical latent variable as side information to encapsulate the sibling and ancestor dependence, which provides sufficient context information for the modeling of point cloud distribution while enabling the parallel encoding and decoding of octree nodes in the same layer. Besides, we propose a residual coding framework for the compression of the latent variable, which explores the spatial correlation of each layer by progressive downsampling, and model the corresponding residual with a fully-factorized entropy model. Furthermore, we propose soft addition and subtraction for residual coding to improve network flexibility. The comprehensive experiment results on the LiDAR benchmark SemanticKITTI and MPEG-specified dataset Ford demonstrate that our proposed framework achieves state-of-the-art performance among all the previous LPC frameworks. Besides, our end-to-end, fully-factorized framework is proved by experiment to be high-parallelized and time-efficient, which saves more than 99.8% of decoding time compared to previous state-of-the-art methods on LPC compression.
更多查看译文
关键词
Point cloud compression,end-to-end learning,octree,deep entropy model,sparse convolution
AI 理解论文
溯源树
样例
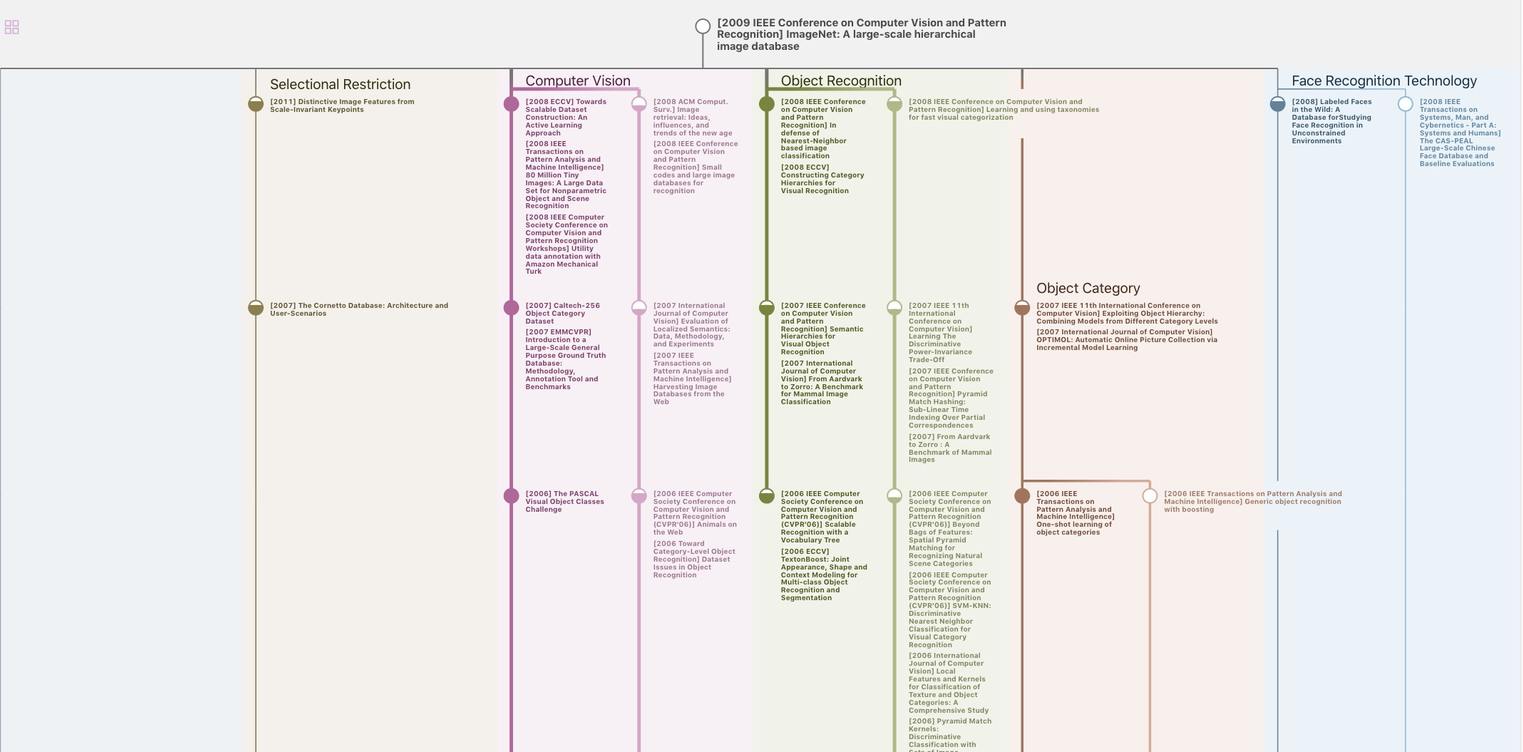
生成溯源树,研究论文发展脉络
Chat Paper
正在生成论文摘要