Weather2vec: Representation Learning for Causal Inference with Non-Local Confounding in Air Pollution and Climate Studies
arxiv(2022)
摘要
Estimating the causal effects of a spatially-varying intervention on a spatially-varying outcome may be subject to non-local confounding (NLC), a phenomenon that can bias estimates when the treatments and outcomes of a given unit are dictated in part by the covariates of other nearby units. In particular, NLC is a challenge for evaluating the effects of environmental policies and climate events on health-related outcomes such as air pollution exposure. This paper first formalizes NLC using the potential outcomes framework, providing a comparison with the related phenomenon of causal interference. Then, it proposes a broadly applicable framework, termed "weather2vec", that uses the theory of balancing scores to learn representations of non-local information into a scalar or vector defined for each observational unit, which is subsequently used to adjust for confounding in conjunction with causal inference methods. The framework is evaluated in a simulation study and two case studies on air pollution where the weather is an (inherently regional) known confounder.
更多查看译文
关键词
causal inference,air pollution,representation learning,weather2vec,non-local
AI 理解论文
溯源树
样例
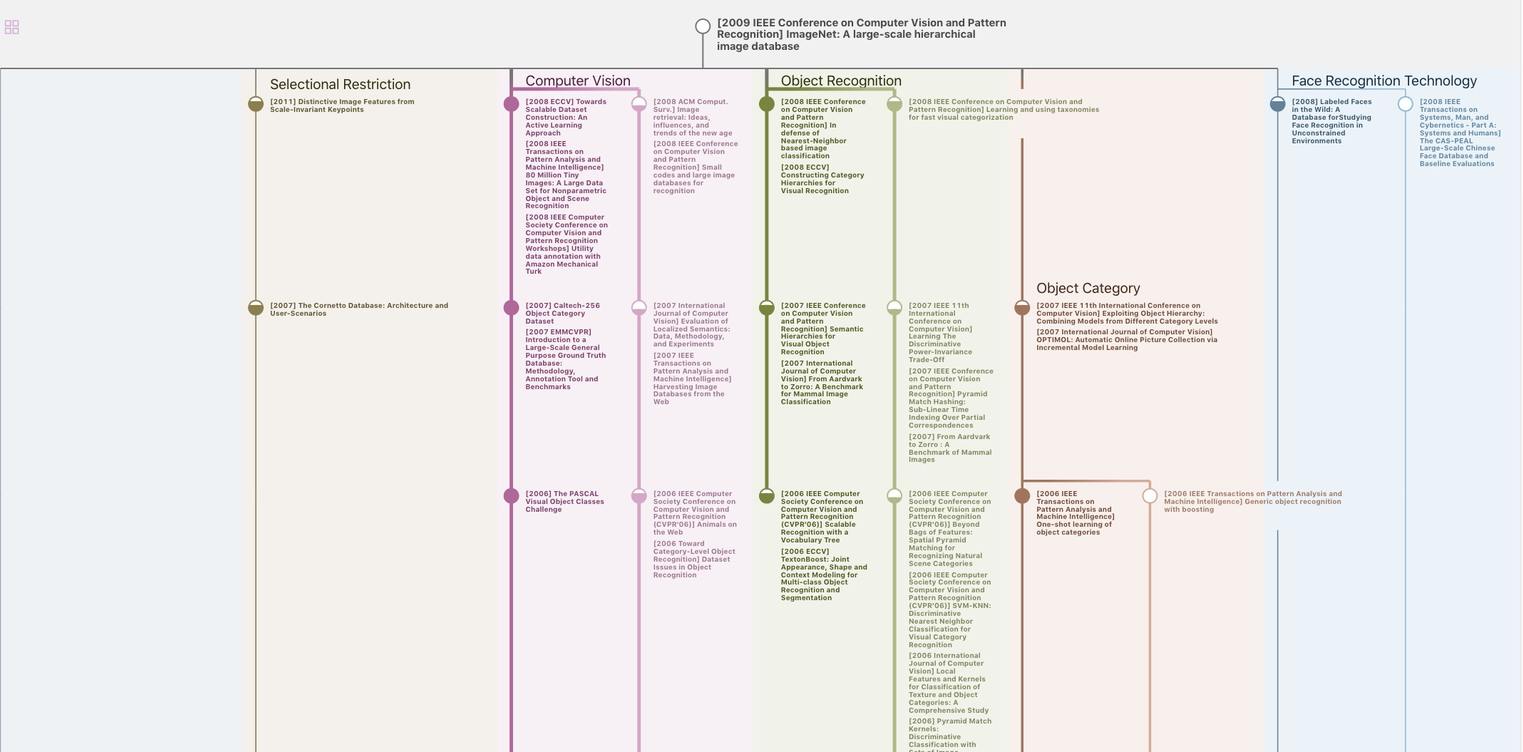
生成溯源树,研究论文发展脉络
Chat Paper
正在生成论文摘要