First-order Conditions for Optimization in the Wasserstein Space
arxiv(2022)
摘要
We study first-order optimality conditions for constrained optimization in the Wasserstein space, whereby one seeks to minimize a real-valued function over the space of probability measures endowed with the Wasserstein distance. Our analysis combines recent insights on the geometry and the differential structure of the Wasserstein space with more classical calculus of variations. We show that simple rationales such as "setting the derivative to zero" and "gradients are aligned at optimality" carry over to the Wasserstein space. We deploy our tools to study and solve optimization problems in the setting of distributionally robust optimization and statistical inference. The generality of our methodology allows us to naturally deal with functionals, such as mean-variance, Kullback-Leibler divergence, and Wasserstein distance, which are traditionally difficult to study in a unified framework.
更多查看译文
关键词
optimization,first-order
AI 理解论文
溯源树
样例
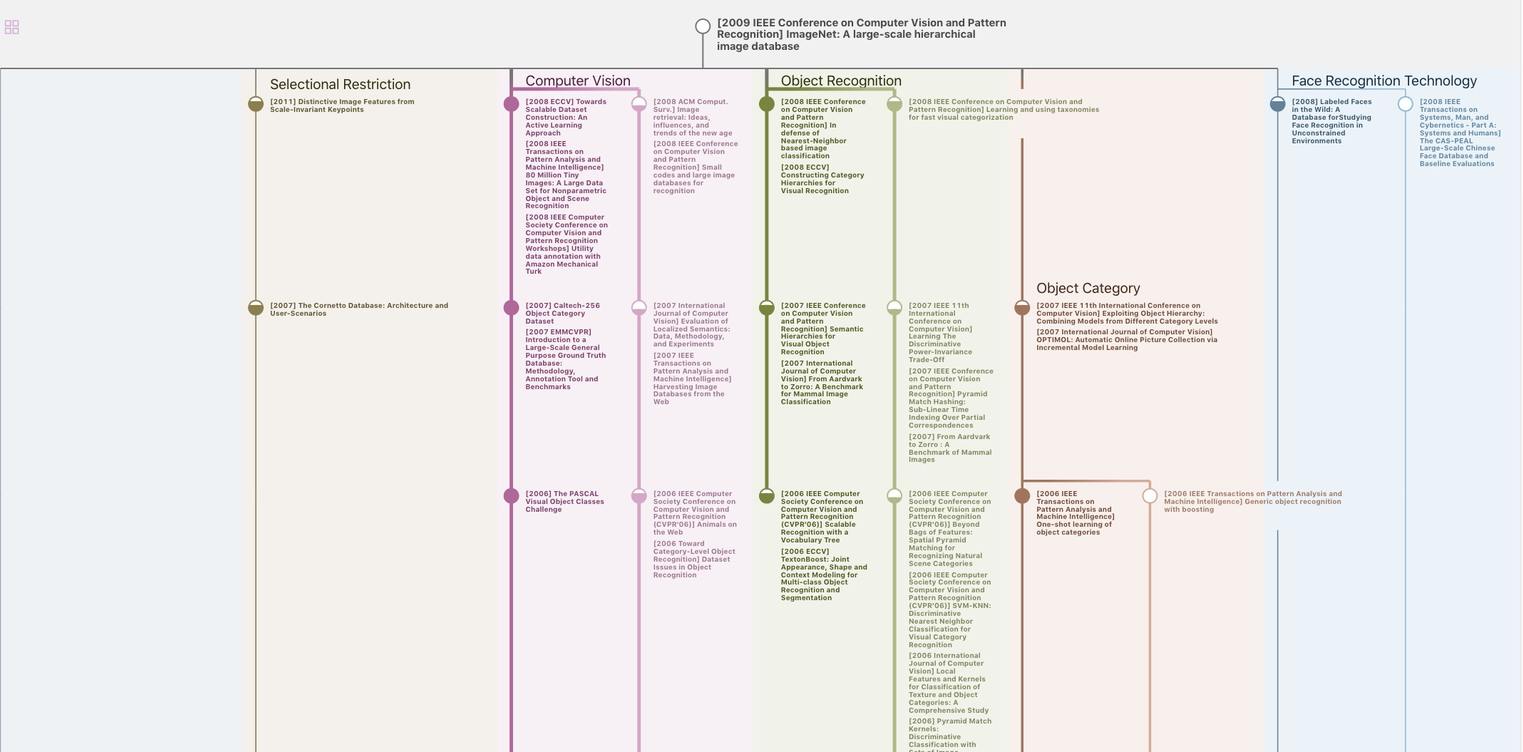
生成溯源树,研究论文发展脉络
Chat Paper
正在生成论文摘要